Enhancing Customer Experience with Vector Databases: A Strategic Approach
Understand how vector databases process data to enhance customer experience and drive business growth.
Read the entire series
- Image-based Trademark Similarity Search System: A Smarter Solution to IP Protection
- HM-ANN Efficient Billion-Point Nearest Neighbor Search on Heterogeneous Memory
- How to Make Your Wardrobe Sustainable with Vector Similarity Search
- Proximity Graph-based Approximate Nearest Neighbor Search
- How to Make Online Shopping More Intelligent with Image Similarity Search?
- An Intelligent Similarity Search System for Graphical Designers
- How to Best Fit Filtering into Vector Similarity Search?
- Building an Intelligent Video Deduplication System Powered by Vector Similarity Search
- Powering Semantic Similarity Search in Computer Vision with State of the Art Embeddings
- Supercharged Semantic Similarity Search in Production
- Accelerating Similarity Search on Really Big Data with Vector Indexing (Part II)
- Understanding Neural Network Embeddings
- Making Machine Learning More Accessible for Application Developers
- Building Interactive AI Chatbots with Vector Databases
- The 2024 Playbook: Top Use Cases for Vector Search
- Leveraging Vector Databases for Enhanced Competitive Intelligence
- Revolutionizing IoT Analytics and Device Data with Vector Databases
- Everything You Need to Know About Recommendation Systems and Using Them with Vector Database Technology
- Building Scalable AI with Vector Databases: A 2024 Strategy
- Enhancing App Functionality: Optimizing Search with Vector Databases
- Applying Vector Databases in Finance for Risk and Fraud Analysis
- Enhancing Customer Experience with Vector Databases: A Strategic Approach
- Transforming PDFs into Insights: Vectorizing and Ingesting with Zilliz Cloud Pipelines
- Safeguarding Data: Security and Privacy in Vector Database Systems
- Integrating Vector Databases with Existing IT Infrastructure
- Transforming Healthcare: The Role of Vector Databases in Patient Care
- Creating Personalized User Experiences through Vector Databases
- The Role of Vector Databases in Predictive Analytics
- Unlocking Content Discovery Potential with Vector Databases
- Leveraging Vector Databases for Next-Level E-Commerce Personalization
- Mastering Text Similarity Search with Vectors in Zilliz Cloud
- Enhancing Customer Experience with Vector Databases: A Strategic Approach
Vector databases are the secret to personalized recommendations and relevant search results in modern applications. Efficient data manipulation, retrieval, and storage are the keys to enhanced customer experience (CX). Before vector databases, customers had a frustrating experience with information retrieval, product recommendations, social media interactions, and real-time insights. Today, vector databases offer customers a personalized experience with sophisticated retrieval algorithms and efficient data handling of ever-growing data volumes without compromising accuracy or speed.
This article will explore how vector databases enhance CX through advanced data handling and personalization capabilities.
Vector Databases Explained
Vector databases are designed to process, store, and retrieve high-dimensional data with scalability and flexibility. They store data in vectors and use similarity metrics to find the closest match during a search, making them efficient for handling complex, high-dimensional data.
Vector databases also integrate with machine learning workflows to store high-dimensional data and use semantic search to power various AI applications, including anomaly detection, image recognition, and recommendation systems.
Improving Personalization and Recommendations
Vector databases enable businesses to deliver personalized customer experiences with features like precise similarity search, fast information retrieval, and scalability. They are powerful tools for finding similar data points, empowering businesses to understand the context behind user queries. This can lead to accurate search results and recommendations.
A few examples of businesses using vector databases to enhance customer experience are:
E-commerce Search and Recommendations
E-commerce platforms use vector databases to boost search functionality and yield context-aware results. For example, when a customer enters the query “soft warm socks,” instead of matching the query with only product descriptions, the vector database finds close matches by computing the similarity of “soft warm socks” with other attributes like fabric, weather suitability, and durability. Vector databases also recommend products to customers based on their search history, user profile, and purchase history.
Vipshop, a Chinese retailer, uses Zilliz Cloud to offer customers a faster search experience and efficient product recommendations. The Milvius database provided a 10x boost to query performance and a less than 30 ms response time for result retrieval.
Content Curation
Vector databases understand user intent by analyzing their profile, search history, and interaction to personalize content recommendations. This leads to increased customer engagement on streaming and social media platforms and helps content creators reach a more targeted audience.
Sohu uses Zilliz to generate highly personalized recommendations for its customers and curate engaging content for them. Milvus improved Sohu’s retrieval speed by ten times and raised the short-news classification accuracy rate to over 95%.
Optimizing Search and Discovery
Relevant search results are the product of context-aware similarity algorithms, scalability, and flexibility of vector databases. Here’s how this is achieved:
Semantic Understanding
Converting data points into vector embeddings allows databases to understand hidden details in data. The embeddings are passed through modern deep learning models for context-aware semantic understanding of the data.
Multimodal Search
Unlike traditional databases, vector databases excel at managing multiple data formats. These include audio, video, images, and text data. Multimodal data management and search enhance user experience by offering customers various ways to search and store their data. For example, Google Lens allows users to upload screenshots to find similar products, and text processing allows voice search.,
Real-time Processing
Vector databases process information in real-time by saving data points as vector embeddings and using sophisticated similarity metrics. Instant search results enhance customer experience by reducing search time and providing accurate results. search time and providing accurate results.
Visualizing Customer Insights
Vector databases use advanced algorithms to process and visualize customer data, revealing hidden patterns to improve customer experience. Let’s suppose an e-commerce store uses a vector database to create a personalized experience for its customers. The following image highlights the role of vector databases in improving the e-commerce customer experience:
How an e-commerce application uses a vector database for similarity search and personalized recommendations
The above image highlights the following steps involved in analyzing and visualizing customer data in an e-commerce store with a vector database:
A vector database stores and processes user data to derive customer insights through data analysis and visualization.
A user performs a search in an e-commerce store.
The e-commerce store queries the vector database to retrieve relevant search results.
The vector database finds matching products and processes user data to display personalized recommendations.
The e-commerce store displays the search results and recommendations online.
Future Trends in CX and Vector Databases
With ever-growing demands for high-dimensional data processing and CX trends inclining towards personalization, vector databases are becoming more prominent in customer experience. Though vector databases are a powerful solution for enhanced customer experience, the technology advancements will bring further innovation in vector databases. These include:
Increased support for a wider range of data types with improved accuracy. This allows for the processing of all kinds of customer data in one place and creates a comprehensive understanding of their personalities.
A rise in hybrid database solutions for even faster search results with high precision. Faster search results lead to quick and easy customer interaction.
No-code, low-code vector database solutions democratize the use of vector databases among non-technical users. Easier access to vector databases allows subject experts to analyze CX trends and eliminates hindrances in customer research and personalization.
Conclusion
Vector databases store and process high-dimensional data in real-time, supporting companies in several ways to achieve their business strategy. Multimodal search and accurate insights enhance customer experience by offering highly personalized results.
With growing data needs, integrating a vector database in your CX toolbox becomes crucial. This allows for building innovative projects and exceeding customer expectations, ultimately increasing customer loyalty and trust.
- Vector Databases Explained
- Improving Personalization and Recommendations
- Optimizing Search and Discovery
- Visualizing Customer Insights
- Future Trends in CX and Vector Databases
- Conclusion
Content
Start Free, Scale Easily
Try the fully-managed vector database built for your GenAI applications.
Try Zilliz Cloud for FreeKeep Reading
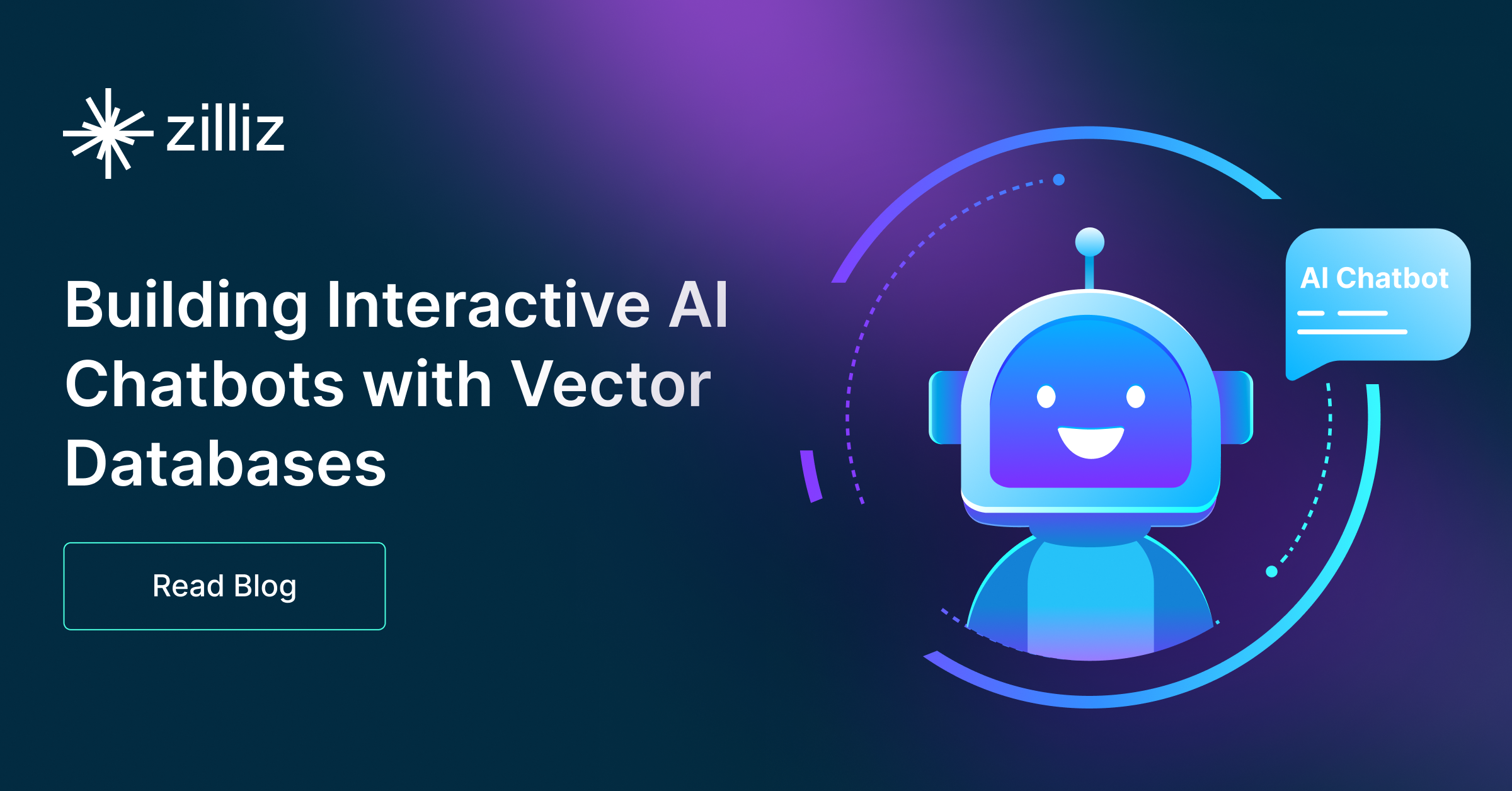
Building Interactive AI Chatbots with Vector Databases
Vector database-powered AI chatbots deliver personalized, context-aware interactions, optimizing user experience through advanced NLP and tech integration.
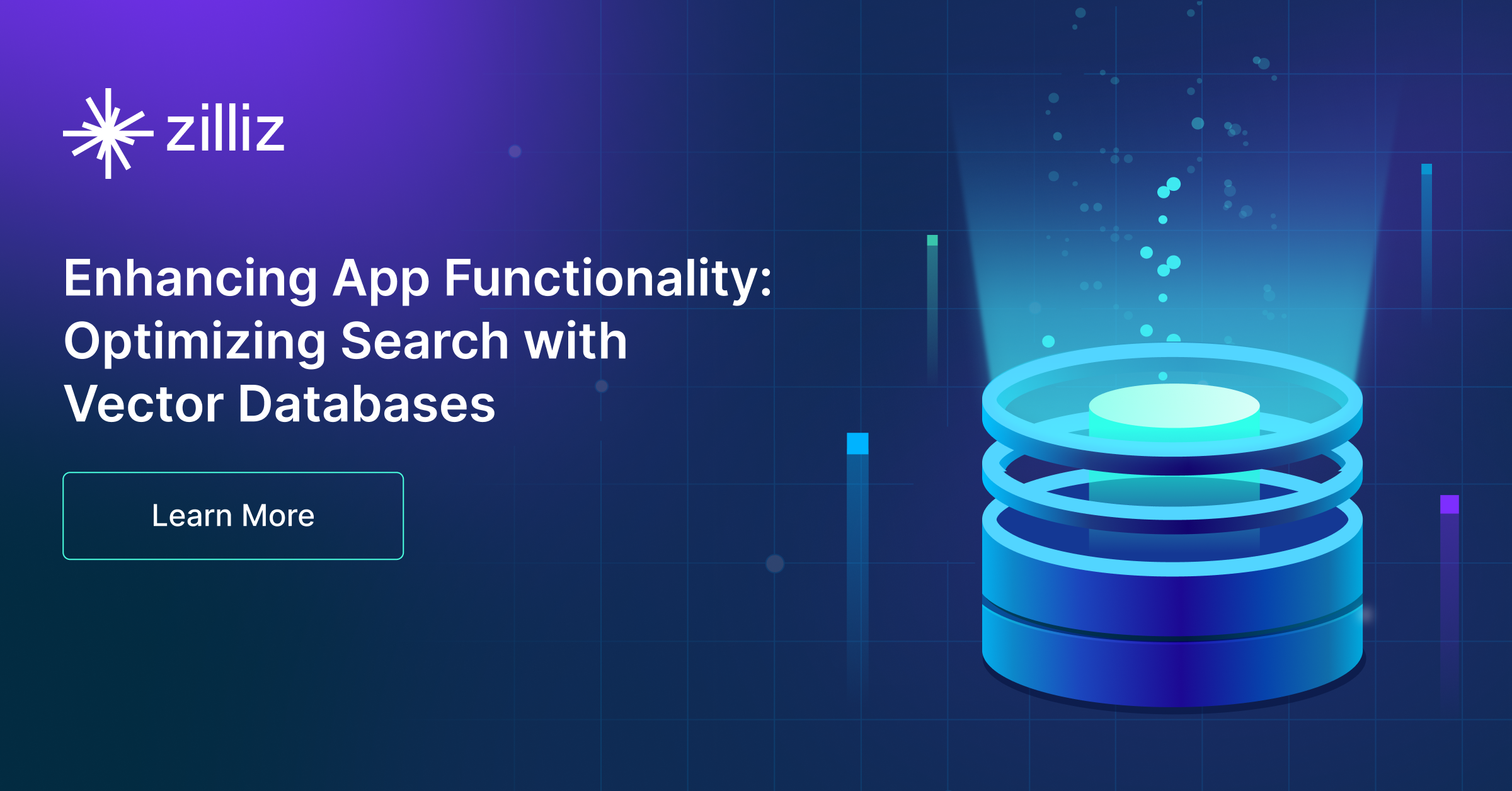
Enhancing App Functionality: Optimizing Search with Vector Databases
Vector databases revolutionize app development by enhancing search functionalities with their ability to perform fast, accurate, and semantic searches.
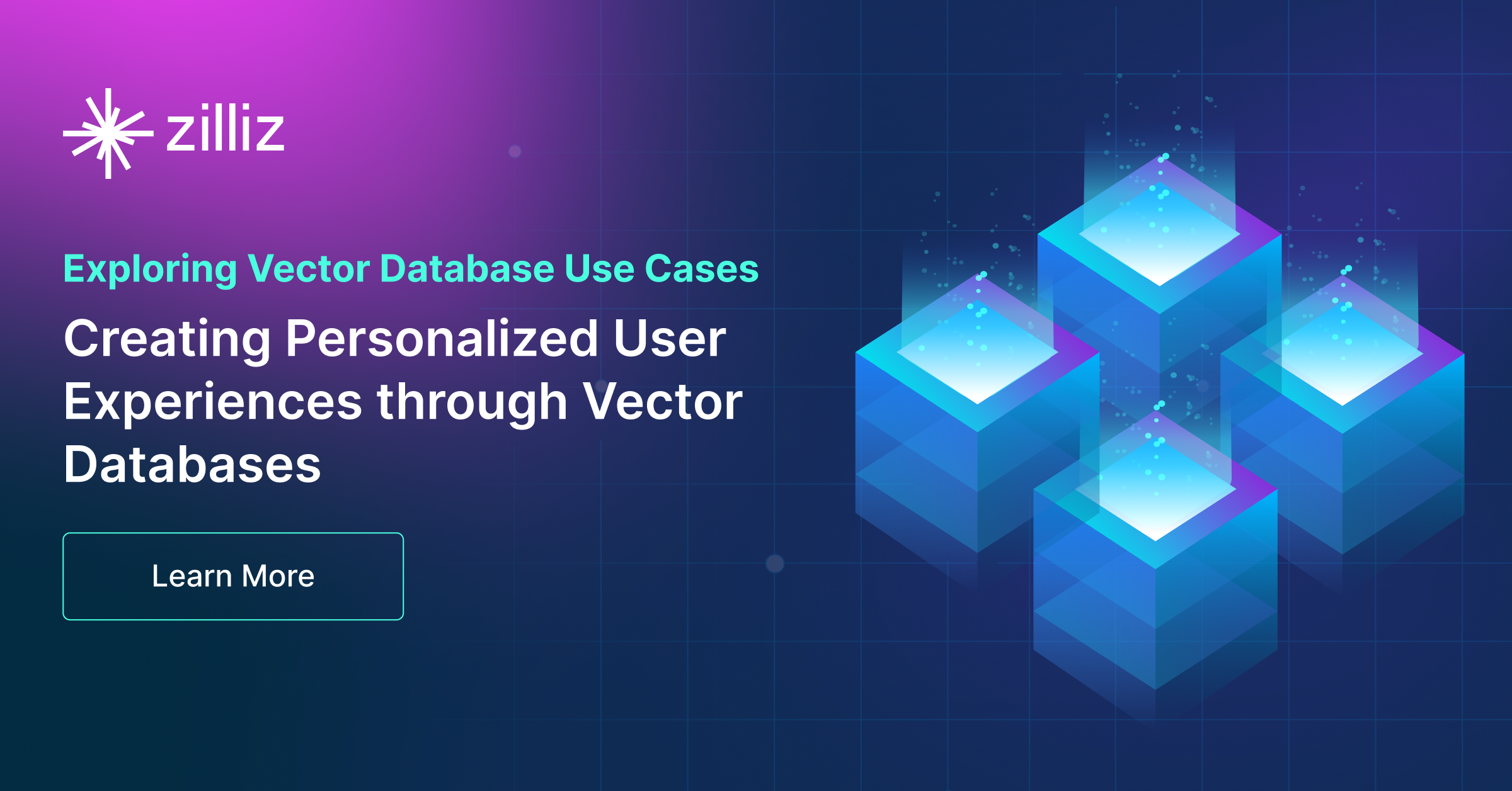
Creating Personalized User Experiences through Vector Databases
Explore how vector databases enhance personalized user experiences