The Evolution and Future of AI and Its Influence on Vector Databases: Insights from Charles, CEO of Zilliz
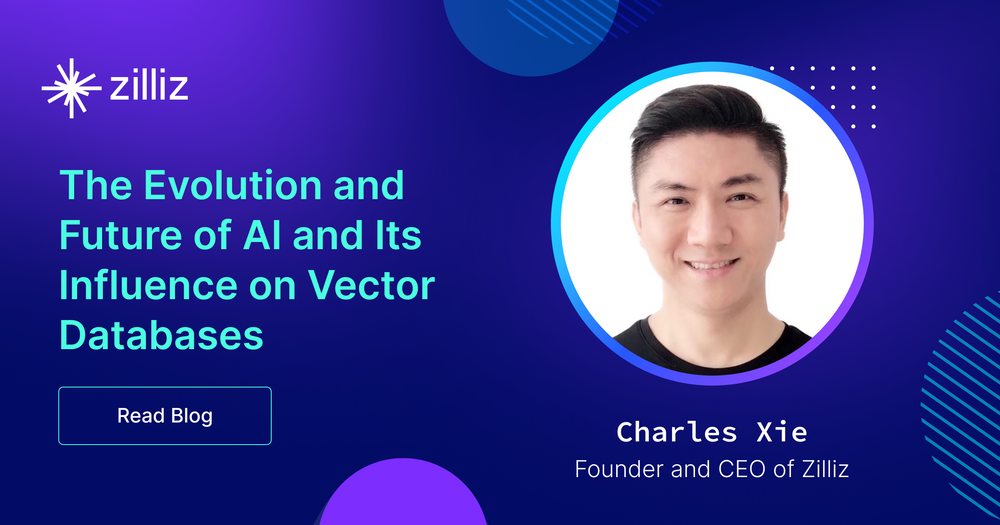
This is the second installment of our two-part blog series.
Welcome back to the second entry in our two-part blog series, where we continue to explore the dynamic interplay between Artificial Intelligence (AI), particularly large language models (LLMs), and vector databases, guided by the insights of Charles Xie, CEO of Zilliz.
In the first part, we laid the groundwork by exploring the nature and current state of vector databases, detailing the development of Milvus, and contemplating the future trajectory of vector database technology. This installment expands upon these foundations, jumping into the evolution of AI technologies, discussing the critical role vector databases play in the age of Foundation Models, and forecasting how these intertwined technologies will shape each other's progress in the future.
Pioneering Vector Databases: Zilliz's Pre-LLM Journey in AI Innovation
Six years ago, we at Zilliz embarked on developing the Milvus vector database, entering a largely uncharted territory well before the advent of large language models. Even then, we believed that vector databases were essential for a broad spectrum of AI applications, opening up extensive use cases and market opportunities.
Back then, while tech giants like Google, DeepMind, and OpenAI made strides in algorithm development, and NVIDIA and Intel enhanced computational power, the critical aspect of data management, particularly for unstructured data, which already comprised 80% of the world's data, was somewhat overlooked. Thus, vector databases emerged as a solution to meet the pressing demand within the pre-LLM AI landscape. They provided a powerful tool for efficiently managing unstructured data in various domains, including language processing, computer vision, and recommendation systems. This technology allows developers to exploit unstructured data more effectively in AI app development, showcasing our pioneering spirit in the vector database domain.
Transitioning from Enterprise-Centric Vector Databases to Democratized AI for All
When we initiated our venture six years ago, our primary goal was to cater to the complex needs of enterprise users and engage with early adopters in areas such as recommendation systems, e-commerce, and image recognition. These sectors demanded a dependable, scalable, and high-performing vector database system for their production environments.
Our journey also saw us forging strategic alliances with established AI firms, particularly those handling massive datasets for natural language processing. At that time, the AI landscape was primarily dominated by hyper scalers and major corporations, where the development of AI algorithms and applications came at a significant cost. These partnerships proved to be invaluable, providing us with unique insights.
However, the AI landscape has undergone significant transformation in the past two years, driven by advancements in modern AI technologies and the proliferation of large language models. We've seen a remarkable increase in the number of independent AI developers, who are mainly small teams or individual contributors. This shift signifies transitioning from a corporate-centric AI era to a more democratized AI environment, where AI is now within reach for a wider array of participants. In response to this changing landscape, our goals have evolved to not only provide high-end enterprise solutions but also to make vector search services more accessible to individual developers and small teams.
On the other hand, While traditional large enterprises have recognized the potential of modern AI technologies and LLMs, they face challenges such as stringent security requirements and a shortage of modern AI expertise. We are helping these enterprises overcome such hurdles by leveraging our AI capabilities and experience in meeting industry standards and requirements.
A prime example of this shift is the creation of Zilliz Cloud, a fully managed version of Milvus on the cloud, which provides affordable offerings and streamlined operations tailored for both developers keen on prototyping and experimenting with AI application development and enterprises seeking modern AI capabilities with scalability, performance, and fault tolerance. This strategic pivot reflects our commitment to democratizing AI, ensuring that cutting-edge vector database technologies are within reach for innovators at every level.
The Significance of Vector Databases in the Rapidly Evolving Era of AI and LLMs
Vector databases are engineered for semantic similarity retrieval within a wide array of unstructured data, including text, images, audio, videos, geospatial data, behavioral patterns, and complex biological sequences, such as genetics and protein structures. These databases enable computers and machines to analyze, understand, and utilize this data through sophisticated deep-learning models and algorithms for knowledge acquisition and decision-making.
The past year has witnessed a dramatic increase in the vector database market, spurred by the rapid advancement of LLMs like ChatGPT. Vector databases serve as LLMs’ long-term memory and external knowledge reservoir, mitigating their issues of "hallucinations."
In addition to large language models, we will also be witnessing the rise of a broader spectrum of Foundation Models over the next few years, already observable in computer vision and soon expanding into fields like autonomous driving, recommendation engines, and biopharmaceutical research. Each Foundation Model necessitates a semantic storage system capable of handling varied data types, including text, images, and user interactions. Vector databases will stand out by offering a practical, accessible, and economical data management solution, enhancing semantic data retrieval across different Foundation Models, thus benefiting developers extensively.
An Expected 80% Increase in RAG Retrieval Accuracy with Milvus 3.0
Retrieval-augmented generation (RAG) is a cutting-edge AI technology that incorporates LLMs and vector databases. Its primary purpose is to address the hallucination issues in LLMs by integrating contextual information. A typical RAG system is composed of a vector database, an LLM, and prompts as code. The vector database plays a crucial role in this system, storing domain-specific or private information and retrieving the most relevant pieces as contexts for the LLM. This process enables the LLM to generate more accurate and higher-quality answers.
Despite significant advancements in RAG technology over the past year, achieving high retrieval accuracy remains a challenge. To address this problem, we are introducing enhanced hybrid search capabilities in our upcoming release of Milvus 3.0. This combination of keyword-based precise and vector-based similarity searches is expected to elevate the recall rates of RAG from the previous 30%-40% to 80%-90%, catering to a broader array of use cases.
What does achieving 80% accuracy entail? Consider an enterprise-grade RAG application: with a 60% accuracy rate, it already outperforms the analytical skills of half the company's workforce. However, boosting this rate to 80% means that the AI's output quality would exceed that of 80% to 90% of the employees, including senior executives. Thus, reaching an 80% accuracy benchmark indicates that AI can provide more precise and quality outcomes, revolutionizing the user experience and amplifying the impact of AI-infused products.
ChatGPT and Vector Databases: Complement or Competitor in the Semantic Search?
Large Language models like ChatGPT and vector databases utilize vector embeddings to represent and understand the semantic meaning of unstructured data. These embeddings are compared using distance metrics like Euclidean or cosine, enabling efficient data analysis and querying based on the data’s semantic similarity. This synergy raises questions about ChatGPT's potential to eclipse vector databases in semantic search.
Despite their overlapping functionalities, ChatGPT is not a substitute for vector databases. ChatGPT's strength lies in understanding and generating coherent and contextually relevant text based on the input it receives rather than functioning as a database to perform exact semantic lookup operations. On the other hand, vector databases are tailor-made to store and retrieve vector embeddings, facilitating efficient semantic similarity searches.
Moreover, the cost implications of conducting semantic searches differ significantly between vector databases and LLMs. Executing semantic search in a vector database system proves to be approximately 100 times more cost-effective than carrying out these operations within a large language model. This substantial cost difference prompts many leading AI companies, including OpenAI, to advocate using vector databases in AI applications for semantic and k-nearest neighbor search.
The distinction between LLMs and vector databases will become more apparent in the next five years. LLMs are poised to emerge as sophisticated semantic processors atop traditional computing infrastructure. At the same time, vector databases have evolved as sophisticated semantic storage solutions, delivering intelligent data retrieval on standard storage mediums like disks, tapes, cloud storage, and file systems.
The Future of AI: The Rise of Affordable General Intelligence within Five Years
In the forthcoming half-decade, as machine learning algorithms evolve, AI is projected to become exponentially smarter, potentially 100x more than today. The crucial challenge, however, lies in making this advanced AI accessible and affordable. While the expenses associated with AI implementations are on a downward trend, the cumulative costs, including hardware acquisition, utilization of large language models, and ongoing operational maintenance, remain considerably high. For instance, reducing the subscription fee of an AI assistant from $20-30 to $3-5 per month could significantly broaden its user base. Thus, the vision for the next five years is the emergence of Affordable General Intelligence (AGI), which aims to make AI-solutions economically viable for all individuals and businesses, thereby achieving the democratization of AI technology.
As a vector database provider, we are committed to contributing to this future by enhancing data management efficiency and reducing costs, thereby enabling broader access to AI technologies.
- Pioneering Vector Databases: Zilliz's Pre-LLM Journey in AI Innovation
- Transitioning from Enterprise-Centric Vector Databases to Democratized AI for All
- The Significance of Vector Databases in the Rapidly Evolving Era of AI and LLMs
- An Expected 80% Increase in RAG Retrieval Accuracy with Milvus 3.0
- ChatGPT and Vector Databases: Complement or Competitor in the Semantic Search?
- The Future of AI: The Rise of Affordable General Intelligence within Five Years
Content
Start Free, Scale Easily
Try the fully-managed vector database built for your GenAI applications.
Try Zilliz Cloud for FreeKeep Reading
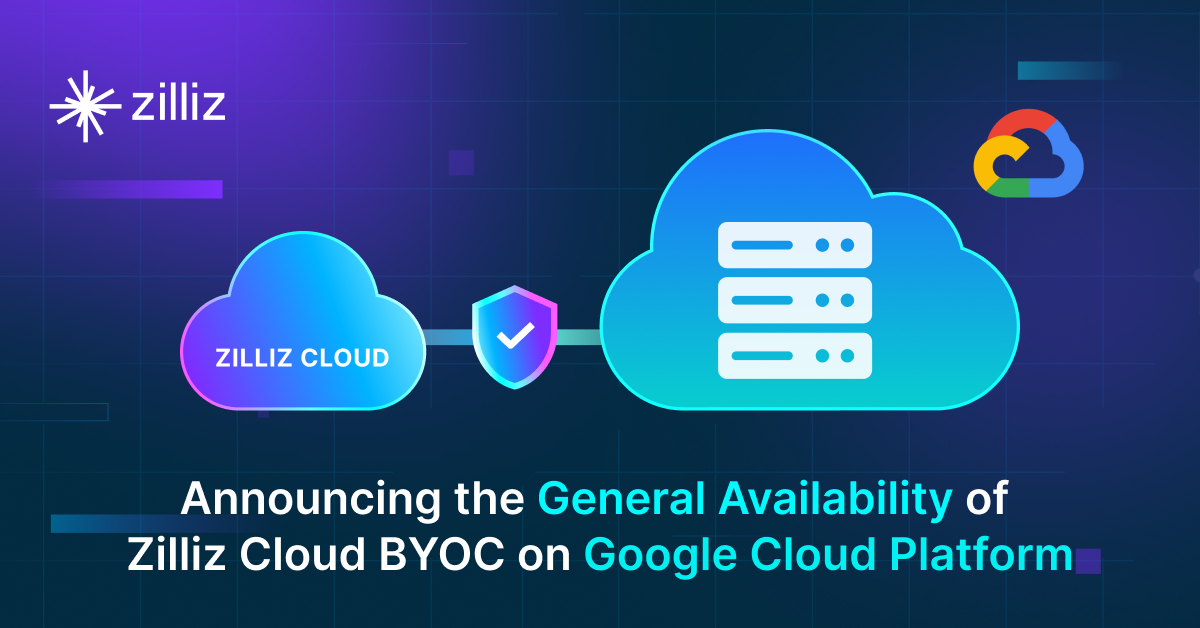
Announcing the General Availability of Zilliz Cloud BYOC on Google Cloud Platform
Zilliz Cloud BYOC on GCP offers enterprise vector search with full data sovereignty and seamless integration.
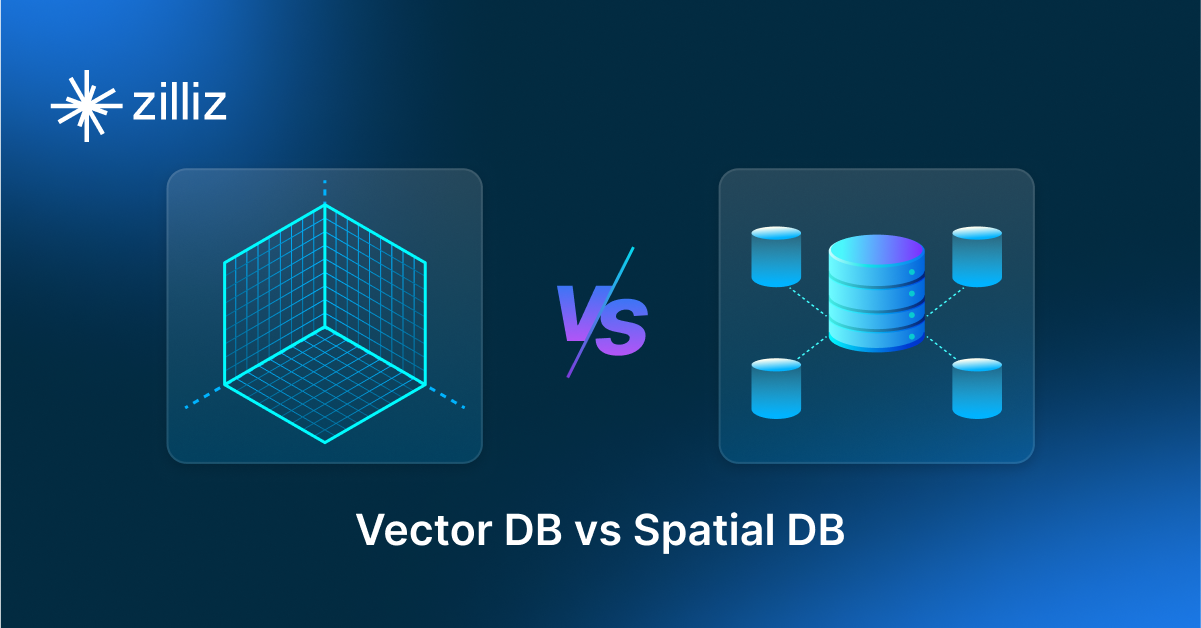
Vector Databases vs. Spatial Databases
Use a vector database for AI-powered similarity search; use a spatial database for geographic and geometric data analysis and querying.
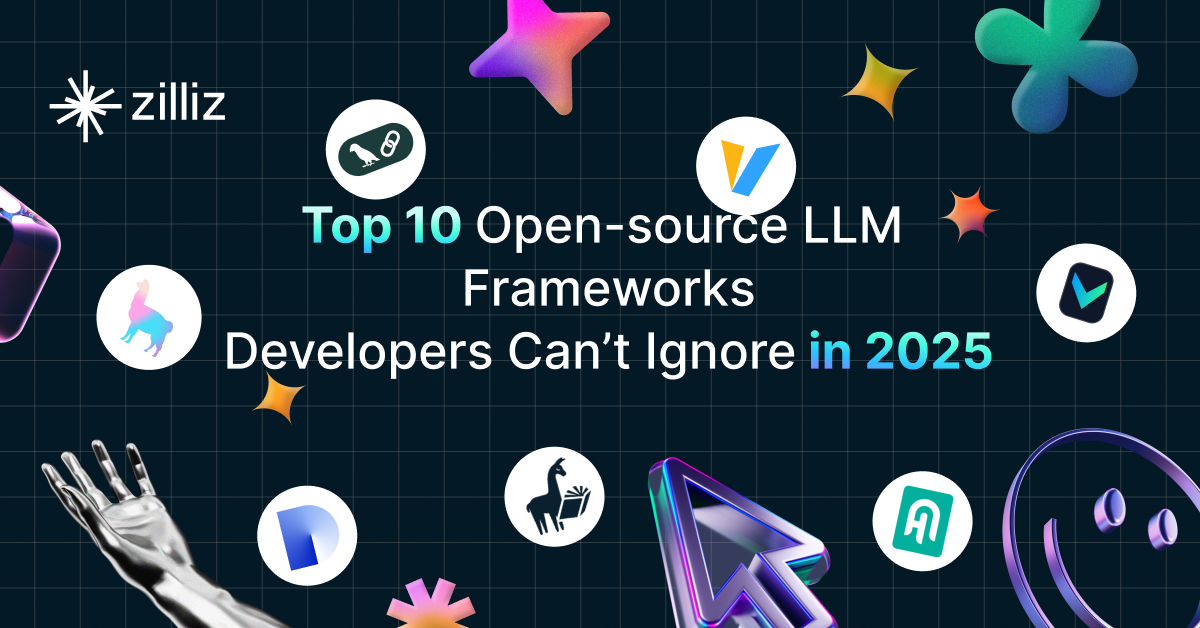
10 Open-Source LLM Frameworks Developers Can’t Ignore in 2025
LLM frameworks simplify workflows, enhance performance, and integrate seamlessly with existing systems, helping developers unlock the full potential of LLMs with less effort.