Top 10 AI Agents to Watch in 2025 🚀
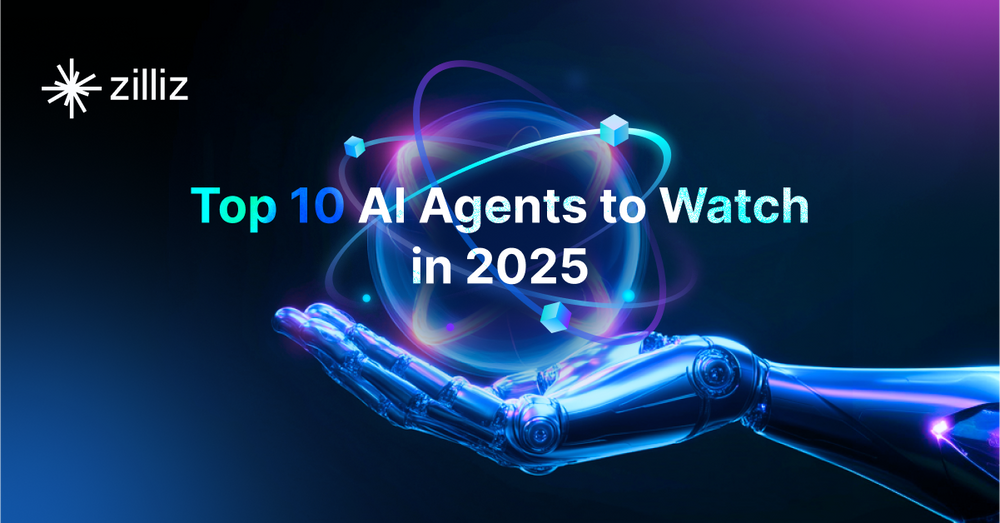
Last year, AI agents weren’t just making headlines—they changed how we work, communicate, and solve problems. From automating tedious tasks to tackling complex challenges, they became indispensable allies for developers and businesses.
As we step into 2025, AI agents are already shaping up to be even more capable and versatile. These systems are like having a supercharged assistant by your side—analyzing data, making decisions, and seamlessly integrating with tools and environments.
Here’s what makes them so game-changing:
🧠 Reasoning: They don’t just follow instructions—they think through problems.
🛠️ Tool Use: Need to connect to APIs or control hardware? They’ve got you covered.
📚 Information Retrieval: With integrations with tools such as large language models (LLMs), vector databases such as Milvus and Zilliz Cloud, and embedding models, they slice through massive datasets like butter.
🌍 Environmental Awareness: They get context in conversation or the physical world.
In this post, we’re breaking down the 10 most impressive AI agents that you should watch in 2025. You’ll want to keep these tools on your radar if you’re interested in building smarter, faster, and more capable apps. Let’s get started! 🌟
OpenAI’s Deep Research: Automating Knowledge Discovery
DeepResearch is OpenAI’s latest AI agent designed to revolutionize how researchers and analysts process vast amounts of information. Built with advanced natural language processing capabilities, DeepResearch autonomously conducts literature reviews, synthesizes key findings, and generates structured reports. By integrating with academic databases, enterprise knowledge bases, and real-time data sources, the AI agent significantly reduces the time required for in-depth analysis.
One of DeepResearch’s standout features is its ability to autonomously refine queries and identify emerging trends in scientific literature, making it an invaluable tool for researchers, policymakers, and industry analysts. Whether it’s uncovering breakthroughs in medicine, analyzing financial trends, or summarizing legal precedents, DeepResearch transforms complex knowledge into actionable insights. As AI-driven research assistants become more sophisticated, DeepResearch is poised to set a new standard for automated knowledge discovery.
Zilliz’s Deep Searcher: A Local Open Source Deep Research Bringing Agentic Capabilities to Everyone
DeepSearcher is an open-source local alternative to OpenAI’s Deep Research. It is also an agentic RAG framework that redefines AI-powered enterprise search. With over 4,900 GitHub stars accumulated in less than a month, this project has quickly gained traction in the developer community.
What sets DeepSearcher apart is its unique combination of advanced reasoning models, sophisticated search features, and an integrated research assistant. By leveraging Milvus (a high-performance vector database built by Zilliz) for local data integration, it delivers faster and more relevant search results while allowing easy model swapping for customized experiences.
Unlike traditional RAG systems that rely on single-query retrieval, DeepSearcher employs agentic RAG capabilities—breaking queries into multiple sub-questions and iteratively refining its search through a closed-loop process of retrieval, processing, validation, and optimization. This approach enables three key advantages: proactive problem-solving instead of passive reactions, dynamic multi-turn retrieval with self-correction, and applicability beyond basic fact-checking to complex reasoning tasks.
For developers, one of DeepSearcher's most valuable features is its comprehensive logging of search and reasoning processes, providing full transparency into how conclusions are reached—crucial for debugging and optimizing RAG systems. Whether you're building enterprise search solutions or need a powerful research assistant for data-intensive projects, DeepSearcher represents the growing trend of open-source, customizable AI agents that bring DeepResearch-like capabilities to local environments.
Google’s Project Astra: Redefining AI Assistance for Daily Life
Google’s Project Astra is an advanced AI agent developed by DeepMind, designed to seamlessly integrate into daily life through multimodal capabilities. Powered by Gemini 2.0, a recently released AI model for the agentic area, Astra processes and responds to diverse inputs—text, images, videos, and audio—making it a highly interactive and intuitive assistant.
Astra’s standout features include real-time memory for contextual understanding, advanced tool usage (e.g., Google Search, Maps, and Lens), and the ability to assist in tasks such as identifying objects or providing recommendations. For example, users can point their phone at a bookshelf and ask Astra to identify the highest-rated book, showcasing its unique ability to connect the digital and physical worlds. With plans to integrate some of its capabilities into other Google products, Astra is set to redefine personal AI in 2025 and beyond.
Currently, Project Astra is being tested by a limited number of trusted users to refine its capabilities and uncover new uses for a universal AI assistant. If interested, you can join the waitlist to become a tester.
Microsoft’s Copilot: Simplifying Workflows and Boosting Productivity
Microsoft Copilot is an AI agent integrated into many Microsoft office platforms like Office 365 and Dynamics 365, designed to streamline everyday tasks and workflows. For example, in Word, Copilot helps you draft reports or refine existing text. In Excel, it helps generate formulas and create visualizations based on natural language input. In Teams, Copilot enhances collaboration by summarizing meetings, highlighting key points, and suggesting action items. Its ability to adapt to the user's context makes it a practical and efficient agent for improving productivity.
For developers, Copilot extends beyond end-user tasks, offering integration with Azure to automate workflows and manage business processes. By embedding AI into the tools people already use daily, Microsoft Copilot simplifies repetitive work, enabling teams to focus on more complex and creative challenges.
Manus AI Assistant: China’s Answer to General AI Agents
Manus AI Assistant is a groundbreaking AI agent developed by the Chinese startup Manus, designed to push the boundaries of autonomous decision-making. Unlike traditional chatbots, Manus operates as a true general AI agent, capable of performing complex multi-step reasoning and executing tasks with minimal human input. The company has partnered with Alibaba’s Qwen AI team to enhance its capabilities, ensuring robust performance across various domains.
A key milestone for Manus AI Assistant is the launch of Monica, its Chinese-language version, which has successfully completed regulatory registrations, allowing it to operate within China’s AI ecosystem. With a growing focus on multimodal interaction and enterprise applications, Manus aims to rival Western AI giants in agent-based intelligence. As the global AI landscape becomes increasingly competitive, Manus is emerging as a strong contender, leveraging China’s AI advancements to redefine what intelligent agents can achieve.
AutoGPT: A Pioneer in Autonomous Task Execution
AutoGPT is an experimental open-source project that transforms GPT models into autonomous agents capable of performing complex, multi-step tasks with minimal human input. By leveraging the power of GPT-4, AutoGPT can break down high-level objectives into smaller, actionable tasks, execute them in sequence, and iterate on their results to achieve the desired outcomes. This makes it one of the earliest examples of an autonomous AI agent widely adopted by developers.
While still in its early stages, AutoGPT has captured the imagination of the AI community by demonstrating the potential of autonomous agents. Developers are experimenting with its capabilities to build applications beyond simple responses, from research assistants to automated workflow managers. AutoGPT highlights the growing trend of AI agents that don’t just provide answers but take action, paving the way for more sophisticated autonomous systems in the future.
BabyAGI: Lightweight Task Automation for Scalable Solutions
BabyAGI is a lightweight, open-source autonomous agent designed to execute tasks iteratively and intelligently. Like AutoGPT, it is powered by GPT-4. It focuses on task management and execution, making it a flexible and powerful tool for developers exploring task-oriented AI solutions. Its modular design allows developers to customize workflows, integrate with various databases, and build solutions tailored to specific needs.
Unlike simpler LLMs that respond to isolated queries, BabyAGI creates a queue of tasks based on an overarching goal, prioritizes them, and completes them step by step. For example, given high-level instructions like "research a topic and summarize findings," BabyAGI will autonomously break it into subtasks, such as gathering resources, analyzing content, and drafting a coherent summary.
What sets BabyAGI apart is its lightweight and scalable design. It delivers core AI capabilities without demanding extensive resources, offering an accessible entry point for organizations with limited budgets. Additionally, its adaptive learning system evolves alongside user needs, ensuring increasingly personalized and efficient assistance over time. Recent updates have also introduced enhanced collaboration features, making BabyAGI a valuable tool for small teams tackling shared projects.
MultiOn’s Agent API: Web Automation Made Simple for Developers
MultiOn's Agent API is a developer-focused platform that enables the integration of AI agents into various applications and devices, automating web-based tasks and enhancing user experiences. Launched in public beta in April 2024, the Agent API allows developers to embed AI agents capable of performing complex actions on the web, such as navigating websites, extracting data, and completing online transactions.
The Agent API supports both Python and JavaScript SDKs, facilitating seamless integration with popular LLM frameworks like LangChain and LlamaIndex. This flexibility allows developers to build custom AI applications that can navigate, scrape, and manipulate web content with ease.
Developers and businesses across various sectors have adopted the Agent API to automate tasks and assist customers. For instance, smart device manufacturers have integrated voice assistants capable of executing actions such as ordering rides, making purchases online, or booking restaurant reservations directly from the device. Similarly, shopping and travel platforms leverage the API to streamline purchasing and booking processes, keeping users engaged and reducing the friction associated with navigating to external sites.
AgentGPT: Your Browser-Based Autonomous AI
AgentGPT is an open-source autonomous AI agent that allows users to deploy task-driven agents directly in their browser. Designed for simplicity and accessibility, AgentGPT enables users to create and manage AI agents capable of executing complex, multi-step tasks with minimal user intervention. It is built to provide a hands-on way for developers and non-developers alike to experiment with the power of autonomous AI.
What makes AgentGPT unique is its in-browser functionality, eliminating the need for extensive setup or external software. This lowers the barrier to entry for experimenting with task-driven AI workflows, making it a valuable tool in the evolving AI landscape.
Vector Databases: The Backbone of Long-Term Agent Memory
AI agents like AutoGPT, Google’s Astra, and others listed in this blog are impressive creations. They don’t just process data—they analyze, reason, and make decisions in ways that feel almost human. However, just like humans, agents need a reliable memory to function effectively. This is where vector databases come in, providing the essential infrastructure for storing, managing, and retrieving contextual data. Many leading AI agents rely on vector databases such as Milvus and Zilliz Cloud to deliver fast, efficient, and scalable memory systems.
Vector databases store information as high-dimensional vectors, capturing the semantic meaning of unstructured data like text, images, or audio. This structure enables AI agents to perform similarity searches and retrieve contextually relevant information instantly. For example, when an agent faces a new query, it can tap into its memory system to find similar past interactions or relevant knowledge, helping it make informed decisions and adapt to new situations. Without such memory, agents would lack the continuity required for advanced reasoning and adaptive learning.
To get started quickly on building an AI agent yourself, check out the tutorials below.
Tutorial: Agentic RAG with Claude 3.5 Sonnet, LlamaIndex, and Milvus
Tutorial: Building an AI Agent for RAG with Milvus and LlamaIndex
Tutorial: Stop Waiting, Start Building: Voice Assistant With Milvus and Llama 3.2
Conclusion
The AI agents featured in this list are just the beginning of what’s possible in 2025. From Google’s Project Astra redefining personal assistance to Amazon Bedrock Agents streamlining enterprise workflows, these tools showcase how AI is changing our ability to work, create, and interact with technology.
Of course, this isn’t an exhaustive list. Other promising agents, such as Anthropic’s Claude Agents, Hugging Face’s Transformers Agents, and Llamaindex’s Llama Agents, are also shaping the future of agentic AI. The diversity and specialization of today’s AI agents mean there’s something for every developer, business, or tech enthusiast looking to unlock the full potential of artificial intelligence. And if none of these agents meet your needs, you can always build your own AI agent tailored to your specific requirements—tools like vector databases, LLMs, and frameworks make it easier than ever to create customized solutions.
As the world of AI evolves, so will the agents that power it. Whether you’re a developer integrating these tools or a business leveraging their capabilities, the key is staying informed and ready to adapt. With so many possibilities, the next breakthrough AI agent might already be in the works—just waiting to transform 2025 and beyond.
Related Resources
Mixture-of-Agents (MoA): How Collective Intelligence Elevates LLM Performance
The Evolution of Multi-Agent Systems From a Methodological Perspective - Zilliz Learn
The Evolution of Multi-Agent Systems From an Algorithmic Perspective - Zilliz Learn
Case study: How Semiconductor Manufacturing Uses Domain-Specific Models and Agentic AI for Problem-solving
- OpenAI’s Deep Research: Automating Knowledge Discovery
- Zilliz’s Deep Searcher: A Local Open Source Deep Research Bringing Agentic Capabilities to Everyone
- Google’s Project Astra: Redefining AI Assistance for Daily Life
- Microsoft’s Copilot: Simplifying Workflows and Boosting Productivity
- Manus AI Assistant: China’s Answer to General AI Agents
- AutoGPT: A Pioneer in Autonomous Task Execution
- BabyAGI: Lightweight Task Automation for Scalable Solutions
- MultiOn’s Agent API: Web Automation Made Simple for Developers
- AgentGPT: Your Browser-Based Autonomous AI
- Vector Databases: The Backbone of Long-Term Agent Memory
- Conclusion
- Related Resources
Content
Start Free, Scale Easily
Try the fully-managed vector database built for your GenAI applications.
Try Zilliz Cloud for FreeKeep Reading
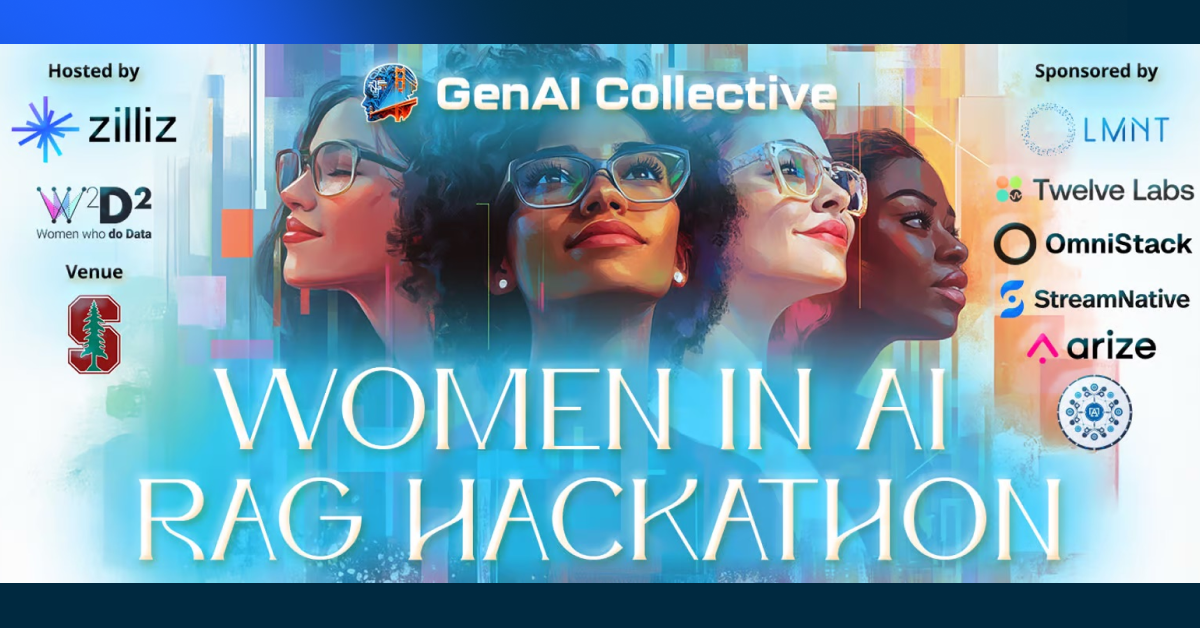
Empowering Innovation: Highlights from the Women in AI RAG Hackathon
Over the course of the day, teams built working RAG-powered applications using the Milvus vector database—many of them solving real-world problems in healthcare, legal access, sustainability, and more—all within just a few hours.
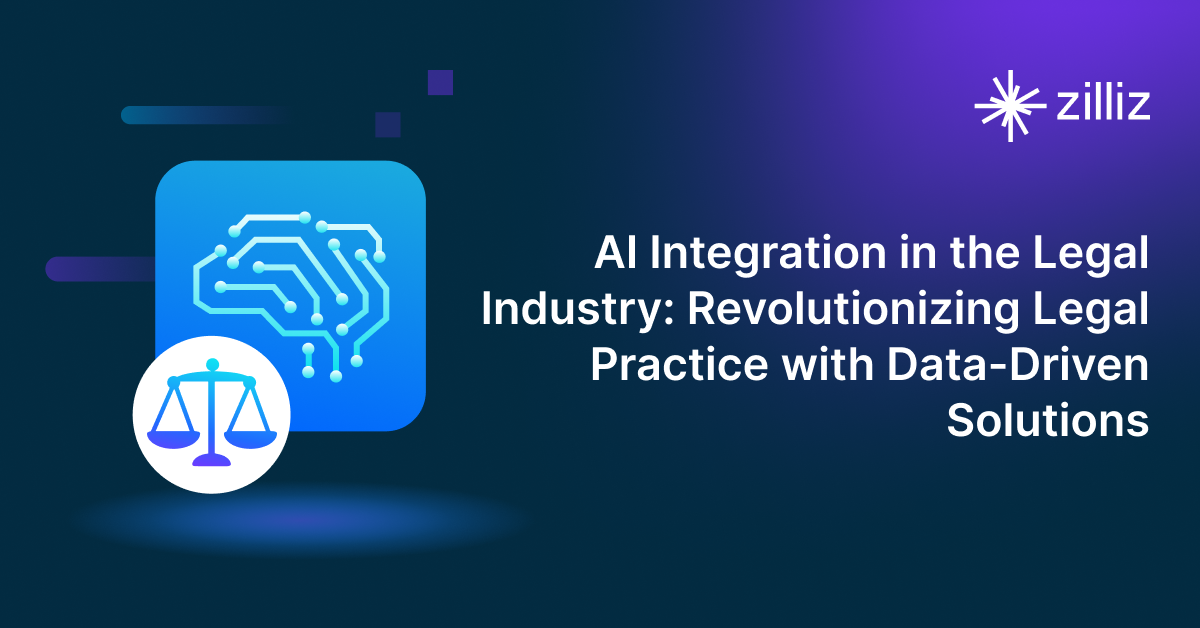
AI Integration in the Legal Industry: Revolutionizing Legal Practice with Data-Driven Solutions
Discover how AI and vector databases are revolutionizing legal work through advanced document processing, semantic search, and contract analysis capabilities.
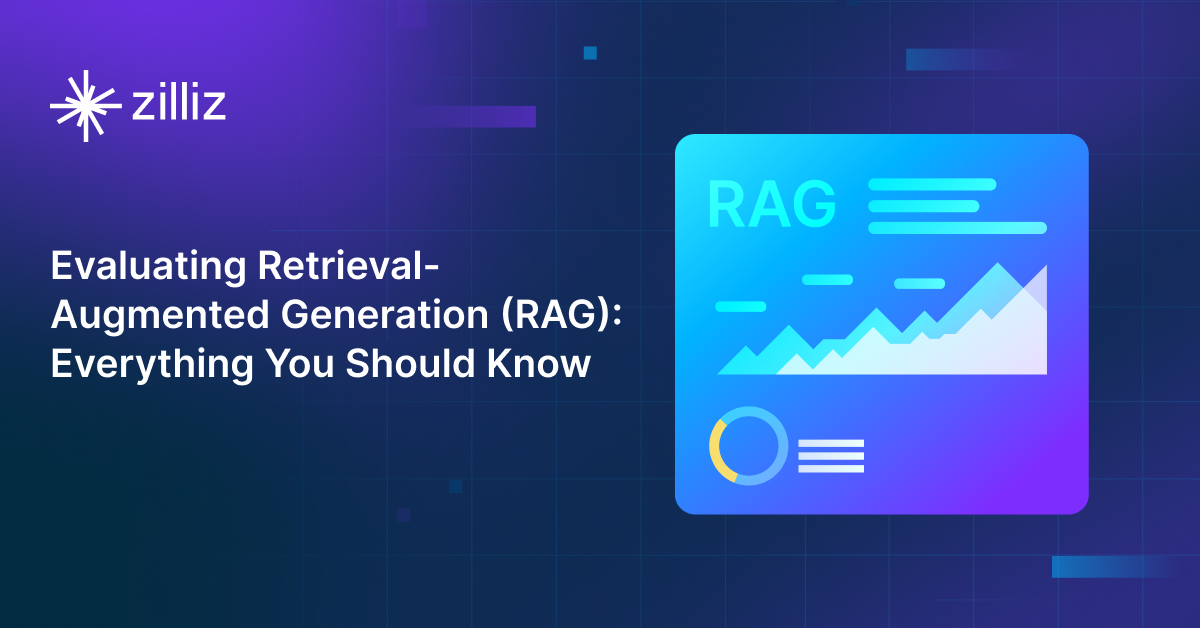
Evaluating Retrieval-Augmented Generation (RAG): Everything You Should Know
An overview of various RAG pipeline architectures, retrieval and evaluation frameworks, and examples of biases and failures in LLMs.