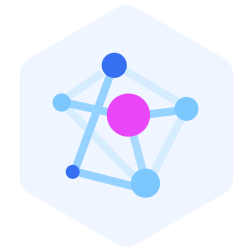
What Are Vector Embeddings?
Vector embeddings are numerical representations of data points in a high-dimensional space in which similar data points are closer together and dissimilar points are farther apart. This process enables the ability to process and identify related data more effectively. For example, the distance between words can indicate semantic similarities in a vector space. Paris and Tokyo are close to each other but far from Apple.
Vector embeddings are commonly used in machine learning and artificial intelligence to capture the semantic meaning of unstructured data (such as text, videos, images, audio, etc.), enabling more efficient and accurate analysis, search, and retrieval. Vector embeddings are usually generated by Neural Networks models (or more modern Transformer architecture) models.
Because the embedding vector representation of a data object contains just numbers, vector similarity search is sometimes called “dense vector search” or “embedding vector search,” which differs from traditional keyword search, where exact matches must occur between the words in the search and the data returned. “Lexical search” and “sparse vector search” are terms that usually refer to traditional keyword searches.
Vector embeddings are usually stored in a modern vector database for indexing and efficient similarity search.
How Are Vector Embeddings Created?
Vector embeddings are often created using machine learning models (also called embedding models) that learn to map raw data to a vector space. The embedding model converts each unit of information into a vector of numbers, and the distance between two vectors signifies how similar they are semantically.
Here are key steps for creating vector embeddings:
- The first step is selecting the data and deciding which variables will form vectors. These may include words in a text corpus, visual imagery, and user preferences.
Next, relevant features from the data will be extracted using tokenization and stemming, and word removal for text data will be stopped. Consider using convolutional neural networks (CNNs) to recognize and extract image features for image data.
After preprocessing the data, input it into a machine learning model such as Word2Vec, GloVe, OpenAI, or another deep neural network model. During the training, the model learns to create vector representations for every item in the dataset. The model rescales these vectors to minimize differences among similar items while increasing differences among different ones.
The result is a multidimensional vector space in which a specific vector represents each item in the dataset. Similar items are closer together in this space, and dissimilar items are further apart.
Sparse, Dense, Binary Vector Embeddings
Vector embeddings can be broadly categorized into three types based on their representation and properties: dense embeddings, sparse embeddings, and binary embeddings. Each type has its own advantages and use cases.
Dense Embeddings (Dense Vectors)
Dense embeddings or dense vectors are vectors where most elements are non-zero, providing a compact numerical representation, that captures rich, continuous data features. Typically, low-dimensional, dense embeddings can efficiently condense information into a smaller space, making them useful for storage and computation. Dense embeddings are commonly found in applications like word embeddings (e.g., Word2Vec, GloVe, FastText), sentence embeddings (e.g., Universal Sentence Encoder, InferSent, Sentence-BERT), and image embeddings derived from convolutional neural networks (e.g., ResNet, VGG). Dense embeddings are highly advantageous in capturing semantic information. They are particularly suitable for neural network-based models and deep learning, which rely on such detailed representations for classification, clustering, and similarity search tasks.
Sparse Embeddings (Sparse Vectors)
Sparse embeddings, or sparse vectors, in contrast, are vectors where most elements are zero, often resulting in high-dimensional representations highlighting the presence or absence of specific features. These embeddings are used in text mining and information retrieval through methods like TF-IDF (Term Frequency-Inverse Document Frequency) and bag-of-words. Sparse embeddings are simple to understand and implement, making them effective for traditional machine-learning algorithms and linear models.
Binary Embeddings (Binary Vectors)
Binary embeddings or binary vectors are vectors where each element is either 0 or 1, creating compact representations that are highly efficient for storage and retrieval. These embeddings are often used in applications requiring high efficiency, such as locality-sensitive hashing (LSH) for approximate nearest neighbor search (ANN) in high-dimensional spaces, binary neural networks where weights and activations are binary, and feature hashing (the hashing trick) to convert large categorical features into fixed-size binary vectors. Binary vector embeddings aid in efficiency regarding storage and computation, making them ideal for large-scale data processing and real-time applications. They are particularly useful for similarity search and retrieval tasks due to their compact nature.
A Simple Guide to Creating Vector Embeddings
Here's an example of using a pre-trained embedding model to generate embeddings for our own words. To follow along, you'll need to install Python and set up the Milvus vector database for vector storage and retrieval.
First, install dependencies: milvus, pymilvus, and gensim. Pymilvus is a Python SDK for Milvus, and gensim is a Python library for natural language processing (NLP).
Gensim is an open-source Python library for topic modeling and document similarity analysis using various unsupervised algorithms. It specializes in processing large-scale text corpora and is widely used in natural language processing (NLP) tasks. Gensim supports training and using word embeddings through machine learning models like Word2Vec, FastText, and Doc2Vec. These embeddings capture semantic relationships between words and can be used for various NLP tasks.
pip install milvus, pymilvus, gensim
Import the libraries.
import gensim.downloader as api
from pymilvus import (
connections,
FieldSchema,
CollectionSchema,
DataType,
Collection)
Create a connection to the Milvus server.
connections.connect(
alias="default",
user='username',
password='password',
host='localhost',
port='19530'
)
Create a collection.
#Creates a collection:
fields = [
FieldSchema(name="pk", dtype=DataType.INT64, is_primary=True, auto_id=False),
FieldSchema(name="words", dtype=DataType.VARCHAR, max_length=50),
FieldSchema(name="embeddings", dtype=DataType.FLOAT_VECTOR, dim=50)
]
schema = CollectionSchema(fields, "Demo to store and retrieve embeddings")
demo_milvus = Collection("milvus_demo", schema)
Load the pre-trained model from gensim.
model = api.load("glove-wiki-gigaword-50")
Generate text embeddings used for sample words.
ice = model['ice']
water = model['water']
cold = model['cold']
tree = model['tree']
man = model['man']
woman = model['woman']
child = model['child']
female = model['female']
Here's an example of the vector embedding for the word "female."
array([-0.31575 , 0.74461 , -0.11566 , -0.30607 , 1.524 , 1.9137 ,
-0.392 , -0.67556 , -0.1051 , -0.17457 , 1.0692 , -0.68617 ,
1.2178 , 1.0286 , 0.35633 , -0.40842 , -0.34413 , 0.67533 ,
-0.5443 , -0.21132 , -0.61226 , 0.95619 , 0.43981 , 0.59639 ,
0.02958 , -1.1064 , -0.48996 , -0.82416 , -0.97248 , -0.059594,
2.396 , 0.74269 , -0.16044 , -0.69316 , 0.55892 , 0.22892 ,
0.013605, -0.44858 , -0.52965 , -0.96282 , -0.54444 , 0.18284 ,
0.16551 , 0.33446 , 0.53432 , -1.4824 , -0.34574 , -0.82834 ,
0.10107 , 0.024414], dtype=float32)
Insert the generated vector embeddings into the collection
#Insert data in collection
data = [
[1,2,3,4,5,6,7,8], # field pk
['ice','water','cold','tree','man','woman','child','female'], # field words
[ice, water, cold, tree, man, woman, child, female], # field embeddings
]
insert_result = demo_milvus.insert(data)
# After final entity is inserted, it is best to call flush to have no growing segments left in memory
demo_milvus.flush()
Create indexes on the entities.
index = {
"index_type": "IVF_FLAT",
"metric_type": "L2",
"params": {"nlist": 128},
}
demo_milvus.create_index("embeddings", index)
Load the collection to memory to confirm the successful upload and do a vector similarity search.
demo_milvus.load()
# performs a vector similarity search:
data = [cold]
search_params = {
"metric_type": "L2",
"params": {"nprobe": 10},
}
result = demo_milvus.search(data, "embeddings", search_params, limit=4, output_fields=["words"])
Loop through the results and print the words.
for i in range(0,4):
hit = result[0][i]
print(hit.entity.get('words'))
And here's the expected output.
cold
ice
water
man
How Do Embeddings Work?
By transforming data like images, text, and audio into numerical representations, embeddings allow machines to understand the underlying semantic meaning and relationships within the raw data. This opens doors to innovative applications across diverse domains.
Finding Similar Images, Videos, or Audio Files
Imagine searching for similar photos or videos based on their content, not just keywords. For example, image search by sound (birds are more easily heard than seen) or video search by image. Vector embeddings make this type of search possible. By extracting embeddings from images, video frames, or audio segments via techniques like Convolutional Neural Networks (CNNs), we can store these embeddings in vector databases like Milvus or Zilliz Cloud. When a user searches with a query image, video, or audio clip, the system retrieves similar items by comparing sequential data with their embedded vector representations.
Accelerating Drug Discovery
Drug discovery is a complex and lengthy process. Vector embeddings can accelerate this process by helping scientists identify promising drug candidates. By encoding the chemical structures of drug compounds into vector embeddings generated above, we can measure their similarity with target proteins. This allows researchers to focus on the most promising leads, leading to expedited drug discovery and development.
Boosting Search Relevance with Semantic Search
Traditional search engines often struggle to understand the true intent behind user queries, leading to irrelevant results. Suppose a company embeds its internal documents into vectors and stores them in a vector database. Now, employees can search those docs using normal human chat. The closest data embeddings to the employee’s chat question embedding could be retrieved by the vector database and sent to ChatGPT as part of a prompt to generate a human-like text answer to the employee’s question. The answer would be the closest answer based on the company’s data. This overall process is called RAG (Retrieval Augmented Generation). Adding a semantic understanding of a company’s documents can significantly enhance internal search relevance for that company and avoid AI hallucinations.
Recommender Systems
Recommender systems are crucial for online platforms, but generic recommendations can be disappointing. Vector embeddings offer a solution by allowing us to represent both users and items as embeddings. By measuring the similarity between the user and the item to generate vector embeddings, we can make personalized recommendations tailored to each user's unique preferences. This leads to more effective recommender recommendation systems and, hopefully, increased user engagement.
Anomaly Detection
Identifying unusual patterns in data is crucial for various applications like fraud detection, network security, and industrial equipment monitoring. Vector embeddings provide a powerful tool for anomaly detection. By representing data points as embeddings, we can calculate distances or dissimilarities between data points. Considerable distances may signal potential anomalies that need to be investigated. This allows us to proactively identify problems, aiding in early anomaly identification and prevention.
Different Types of Vector Embeddings Based on the Nature of the Data Being Represented
Vector embeddings can also be divided into word embeddings, image embeddings, graph embeddings, multimodal embeddings, etc, depending on the application and the nature of the data being represented. Here are some of the most common types of vector embeddings.
- Word Embeddings: Word embeddings represent words as numerical vectors in a continuous vector space. They are trained on large text corpora like Wikipedia using various models like Word2Vec, GloVe, or fastText. These models use varying mechanisms to represent each word as a vector of numbers. Word2Vec generates word embeddings by forecasting a word in an enormous textual corpus. It defines linguistic relations among words. For example, it may represent "king" and "queen" as vectors close together in the space, showing their semantic similarity. GloVe, on the other hand, relies on the co-occurrence data of words to construct vectors. GloVe might represent the words "ice" and "water" as vectors that are close together because they often appear together in text and share a semantic relationship. fastText takes word embeddings to a sub-word level, allowing one to deal with out-of-vocabulary (OOV) words or variations.
- Sentence and Document Embeddings: Sentence and document embeddings represent entire sentences or documents as numerical vectors using models like Doc2Vec and BERT. Doc2Vec builds upon the Word2Vec paradigm to generate document-level embeddings, allowing the encoding of entire documents or passages. BERT (Bidirectional Encoder Representations from Transformers) considers the context of each term in a sentence, leading to highly context-aware vectors of embeddings.
- Image Embeddings: CNNs can produce image embeddings through feature extraction at varying network layers. They provide a valuable approach to image classification and retrieval. For example, a photo of a cat might have an image embedding vector with features that represent its ears, fur, and tail. Other models like Inception and ResNet also have layers that can extract features from images. An Inception model can generate an embedding that represents the visual attributes of an image, like objects or patterns.
- Time Series Embeddings: You can embed time series data using long short-term memory (LSTM) and gated recurrent unit (GRU) neural networks, which can capture temporal dependencies. LSTM—or GRU-based embeddings can capture temporal dependencies for time series data like stock prices. For instance, these vector embeddings can represent patterns in stock price movements.
- Audio Embeddings: In audio processing, mel-frequency cepstral coefficients (MFCC) embeddings represent audio data for audio classification tasks. In speech recognition, MFCC embeddings capture the acoustic characteristics of audio signals. For example, they can represent the spectral content of spoken words.
- Graph Embedding: Consider a social network graph. Node2Vec can generate node embeddings where similar users (nodes) have closer vectors. For instance, users with similar interests might have similar vector embeddings. You can also use graph neural networks (GNNs) to generate embeddings for nodes and capture complex relationships in graphs. GNNs can represent users and items in a recommendation system and predict user-item interactions.
Applications of Vector Embeddings
Here's how you can apply vector embeddings in different domains.
- Image, video, and audio vector similarity search: You can extract embeddings from images, video frames, or audio segments using techniques like CNNs for image or feature extraction. Store these embeddings in a vector database. When a user queries with an image, video frame, or audio clip, retrieve similar items by measuring the similarity between their embeddings.
- AI drug discovery: By encoding the chemical structures of compounds into embeddings, you can use these embeddings to measure the similarity between drug compounds and predict the potential target proteins for drug compounds.
- Semantic search engine: Vector embeddings enhance search engine capability by matching the meaning of queries to relevant documents, improving search relevance.
- Recommender system: You can represent users and items as embeddings. Measure user-item similarity to make personalized recommendations. Tailor-made recommendations enhance user experience.
- Anomaly detection: By representing data points as embeddings, you can identify unusual patterns in data. You achieve this by calculating the distance or dissimilarity between data points. Data points with huge distances are potential anomalies. This can be helpful in fraud detection, network security, industrial equipment, or process monitoring.
See more vector embedding and vector database use cases.
Why Use Vector Embeddings
- Pattern recognition: Vector embeddings capture patterns, similarities, and dissimilarities in data. Machine learning models benefit from embeddings representing meaningful patterns, improving performance in tasks like classification and clustering.
- Dimensionality reduction: Embeddings reduce the dimensionality of data. They transform high-dimensional data into lower-dimensional vectors, simplifying computational tasks and often improving efficiency.
- Semantic understanding: Vector embeddings encode semantic relationships between data points, making it easier for machines to understand and interpret complex information.
- Efficient processing: Numerical vectors are computationally efficient. Machine learning algorithms can process numerical data faster and with less computational cost.
- Transfer learning: Pretrained embeddings, such as word embeddings in NLP or image embeddings in computer vision, can be fine-tuned for specific tasks. This reduces the need for vast amounts of labeled data, accelerates model convergence, and enhances model performance.
Vector Embedding FAQs
Where Do You Store Vector Embeddings?
You can store vector embeddings in a vector database like Milvus, an in-memory database like Redis, databases like PostgreSQL, or your file system. The choice of where to store vector embeddings depends on factors like data volume, access patterns, and the application's specific requirements.
What Is the Difference Between Vector Databases and Vector Embeddings?
Vector databases are specialized databases that index, store, and retrieve vector data. In contrast, vector embeddings are numerical representations of data points (such as sentences, images, or other objects) in a continuous vector space. The embeddings capture meaningful relationships and patterns within the data, while databases store the vectors and optimize similarity searches based on vector similarity metrics.
What Are Vectors?
Vectors represent unstructured data using multi-dimensional arrays of numbers. Examples include audio vectors, image vectors, video, or text. They enable operations like vector search. Search algorithms like KNN, ANNS, or HNSW organize data in such a way as to make calculating the distance between vectors fast. Distance between vectors determines similarity, and the closest vectors are returned in the search. This is useful for clustering, anomaly detection, reverse image search, answering chat questions, or making recommendations. Creating the data structure for efficient vector search is called creating the vector index.
Are Embeddings Different From Vectors?
Embeddings vs. vectors: Technically, a vector is a fundamental mathematical object represented by a multidimensional array of numbers, while embeddings are a technique for transforming unstructured data into vector representations using mathematical operations that capture semantic relationships between data points. But in most cases, especially in the context of machine learning, “vectors” and “embeddings” can be used interchangeably.
What Is a Vector Index?
A vector index, also known as a vector database index or similarity index, is a data structure used in vector databases to organize and optimize the retrieval of vector data based on similarity metrics. Examples of indexes are FLAT, IVF_FLAT, IVF_PQ, IVF_SQ8, HNSW, and SCANN for CPU-based ANN searches and GPU_IVF_FLAT and GPU_IVF_PQ for GPU-based ANN searches.
What Is the Difference Between Pre-trained Embeddings and Custom Embeddings?
Pretrained embeddings come from pre-trained deep-learning models called checkpoints. These checkpoints are usually open-source transformer models that have been trained on public data. Custom embeddings come from your own deep learning models trained on your own custom, domain-specific training data too.
Does Zilliz Vector Database Create Vector Embeddings?
Yes. Zilliz Cloud is a fully managed vector database service of Milvus, capable of creating, storing, and retrieving billions of vector embeddings.
Zilliz Cloud boasts a powerful Pipelines feature, which allows you to convert unstructured data into high-quality searchable vector embeddings. This feature streamlines the vectorization workflow for developers, covering vector creation, retrieval, and deletion for swift and efficient operations. It also minimizes maintenance costs for developers and businesses by eliminating the need to adopt additional tech stacks like embedding models to build their applications.
Best Vector Databases for Vector Embeddings
There is no universal “best” vector database; the choice depends on your needs. Therefore, it is vital to evaluate a vector database’s scalability, functionality, performance, and compatibility with your particular use cases.
Modern vector embedding databases should be capable of efficiently searching vectors, filtering using metadata, and performing both semantic search and keyword searches. They should also be scalable, configurable, and performant to handle enterprise-level workloads.
One well-recognized open-source benchmarking tool to help evaluate is ANN-Benchmark. ANN-Benchmark allows you to graph the results of testing recall/queries per second of various algorithms based on a number of pre-computed datasets. It plots the recall rate on the x-axis against QPS on the y-axis, illustrating each algorithm’s performance at different levels of retrieval accuracy.
In addition to using benchmarking tools, you can also refer to these comparison charts of mainstream open-source vector databases and fully managed vector database services on their architecture, scalability, performance, use cases, costs, and feature sets.
- What Are Vector Embeddings?
- How Are Vector Embeddings Created?
- Sparse, Dense, Binary Vector Embeddings
- A Simple Guide to Creating Vector Embeddings
- How Do Embeddings Work?
- Different Types of Vector Embeddings Based on the Nature of the Data Being Represented
- Applications of Vector Embeddings
- Why Use Vector Embeddings
- Vector Embedding FAQs
Content
Start Free, Scale Easily
Try the fully-managed vector database built for your GenAI applications.
Try Zilliz Cloud for Free