Experimenting with Different Chunking Strategies via LangChain for LLM Apps
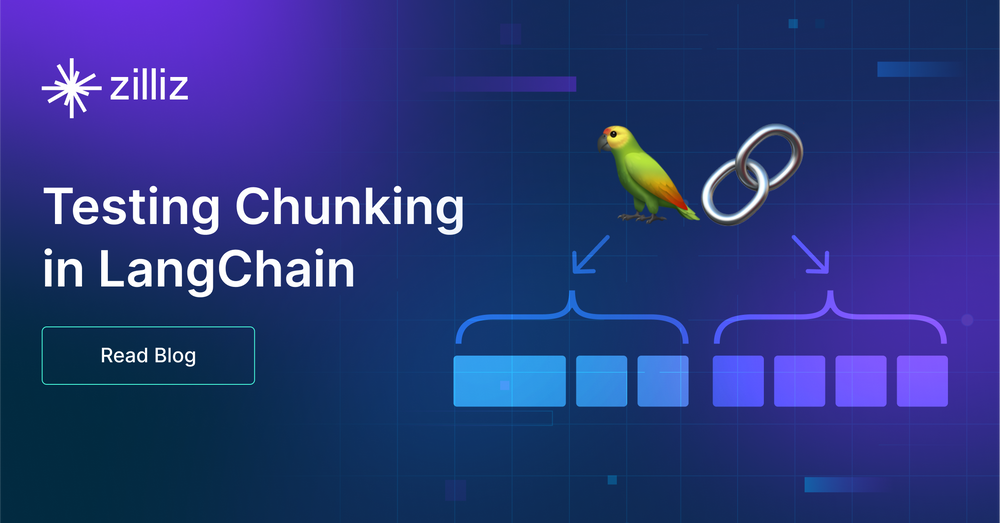
Chunking is among the most challenging problems in building retrieval-augmented generation (RAG) applications. Chunking is the process of sentence splitting into smaller, manageable pieces for downstream processing. While it sounds simple, the devil is in the details. A poorly chosen chunking strategy can lead to irrelevant or incomplete results, making it harder for an AI system to provide accurate responses. For example, overly small chunks may miss context, while overly large chunks may return irrelevant information.
In this tutorial, we explore how different chunking strategies affect retrieval performance for the same dataset, in particular we will focus on a use case with Langchain chunking. By the end of this guide, you’ll understand how chunk size and overlap influence retrieval quality and gain practical insights into choosing the right parameters for your specific use case. The code for this post can be found in this GitHub Repo on LLM Experimentation.
LangChain Overview and Why Chunking Matters
LangChain is an Large Language Model Orchestration framework with built-in tools for document loading and text splitting. Its flexibility makes it a popular choice for building RAG applications, where chunking plays a critical role in determining the relevance and completeness of retrieved information.
Chunking involves selecting two main parameters: a given chunk, size and overlap.
Chunk sizes defines the number of characters or tokens in each chunk. Larger chunks capture more context but risk returning irrelevant information, while smaller chunks ensure precision but may lose necessary context. Overlap determines how much text is shared between consecutive chunks. It helps preserve continuity, especially for connected ideas across paragraphs, but excessive overlap increases processing overhead. Optimizing these parameters ensures a balance between capturing context and maintaining focus when you split text, both critical for accurate retrieval.
Chunking strategies have wide applications beyond RAG workflows. They are crucial in context-aware chatbots, which rely on chunked text to provide concise yet comprehensive responses to user queries. For instance, a support bot may use chunking to break troubleshooting guides into actionable steps without overwhelming the user. Similarly, in knowledge graph construction, chunking extracts entities and relationships from raw text, helping transform unstructured documents into structured data. These examples demonstrate how the right chunking strategy can enhance downstream AI tasks.
Understanding the Challenges of Poor Chunking
A poorly optimized chunking strategy can create significant issues in retrieval. Really small chunk sizes often lack sufficient context, producing fragmented or incomplete results. For instance, querying a product manual might return isolated fragments like, “Step 1: Turn on the power,” with no accompanying information about subsequent steps. Conversely, when your application splits text into overly large chunks might dilute the specificity of the retrieved information. A semantic similarity query that expects concise troubleshooting instructions could return an entire section, making it harder for users to pinpoint relevant details.
Overlap adds another layer of complexity. Without overlap, systems may lose the continuity of thought between consecutive chunks, which is critical for connected narratives or processes. However, excessive overlap can introduce redundancy, increasing storage and processing costs. Balancing these trade-offs is vital to ensure retrieval systems deliver accurate, actionable responses.
LangChain code imports and setup
This first section focuses on imports and other setup tools. Perhaps the first thing you notice about the code below is that there are a BUNCH of imports. The more used ones are: os, and dotenv, so I won’t cover them. They are simply used for your environment variables. Let’s step through text splitting in LangChain with python and the pymilvus client.
At the top, you’ll see our three imports for getting the doc in. First, there’s NotionDirectoryLoader, which loads a directory with markdown/Notion docs. Then, we have the Markdown Header and Recursive Character text splitters. These split the text within the markdown doc based on headers (the header splitter), or a set of pre-selected character breaks (the recursive splitter).
Next, we’ve got the retriever imports. Milvus is our vector database, OpenAIEmbeddings is our embedding model, and OpenAI is our LLM. The SelfQueryRetriever is the LangChain native retriever that allows a vector database to “query itself”. I wrote more about using LangChain to query a vector database in this piece.
Our last LangChain import is AttributeInfo, which passes in an attribute with info into the self-query retriever, as one may guess. Lastly, I want to touch on the pymilvus imports. These are strictly for utility reasons; we don’t need these to work with a vector database in LangChain. I use these imports to clean up the database at the end.
The last thing we do before writing the function is load our environment variables and declare some constants. The headers_to_split_on variable is essential - it lists all the headers we expect to see and want to split on in the markdown. path just tells LangChain where to find the Notion docs.
import os
from langchain.document_loaders import NotionDirectoryLoader
from langchain.text_splitter import MarkdownHeaderTextSplitter, RecursiveCharacterTextSplitter
from langchain.vectorstores import Milvus
from langchain.embeddings import OpenAIEmbeddings
from langchain.llms import OpenAI
from langchain.retrievers.self_query.base import SelfQueryRetriever
from langchain.chains.query_constructor.base import AttributeInfo
from pymilvus import connections, utility
from dotenv import load_dotenv
load_dotenv()
zilliz_uri = os.getenv("ZILLIZ_CLUSTER_01_URI")
zilliz_token = os.getenv("ZILLIZ_CLUSTER_01_TOKEN")
headers_to_split_on = [
("##", "Section"),
]
path='./notion_docs'
Building a chunking experimentation function
Building the experimentation function is the most critical part of the tutorial. As mentioned, this function takes some parameters for document ingestion and experimentation. We need to provide the path to the docs, the headers to split on (splitters), the chunk size, the maximum chunk size overlap, and whether or not we want to clean up by dropping the collections at the end. The collection drop defaults to true
If we can avoid it, we want to create and drop collections as sparingly as possible because they have overheads we can prevent. You may see the script change as I look for good workarounds.
This function is very similar to the one we linked above about using Notion with LangChain. The first section loads the document from the path using the Notion Directory Loader. Notice that we only grab the html content for the first web page (and only have one page).
Next, we grab our splitters. First, we use the markdown splitter to split on the headers we passed in above. Then, we use our recursive splitter method and split based on the chunk size and overlap.
That’s all the splitting we need. With the splitting done, we give a collection name and initialize a LangChain Milvus instance using the default environment variables, OpenAI embeddings, splits, and the collection name. We also create a list of metadata fields via the AttributeInfo object to tell the self-query retriever we have “sections.”
With all this setup, we get our LLM and then pass it into a python self-query retriever. From there, the retriever does its magic when we ask it a question about our docs. I’ve set it up also to tell us which chunk strategy we are testing. Finally, we can drop the collection if we’d like.
def test_langchain_chunking(docs_path, splitters, chunk_size, chunk_overlap, drop_collection=True):
path=docs_path
loader = NotionDirectoryLoader(path)
docs = loader.load()
md_file=docs[0].page_content
# Let's create groups based on the section headers in our page
markdown_splitter = MarkdownHeaderTextSplitter(headers_to_split_on=splitters)
md_header_splits = markdown_splitter.split_text(md_file)
# Define our text splitter
text_splitter = RecursiveCharacterTextSplitter(chunk_size=chunk_size, chunk_overlap=chunk_overlap)
all_splits = text_splitter.split_documents(md_header_splits)
test_collection_name = f"EngineeringNotionDoc_{chunk_size}_{chunk_overlap}"
vectordb = Milvus.from_documents(documents=all_splits,
embedding=OpenAIEmbeddings(),
connection_args={"uri": zilliz_uri,
"token": zilliz_token},
collection_name=test_collection_name)
metadata_fields_info = [
AttributeInfo(
name="Section",
description="Part of the document that the text comes from",
type="string or list[string]"
),
]
document_content_description = "Major sections of the document"
llm = OpenAI(temperature=0)
retriever = SelfQueryRetriever.from_llm(llm, vectordb, document_content_description, metadata_fields_info, verbose=True)
res = retriever.get_relevant_documents("What makes a distinguished engineer?")
print(f"""Responses from chunking strategy:
{chunk_size}, {chunk_overlap}""")
for doc in res:
print(doc)
# this is just for rough cleanup, we can improve this
# lots of user considerations to understand for real experimentation use cases though
if drop_collection:
connections.connect(uri=zilliz_uri, token=zilliz_token)
utility.drop_collection(test_collection_name)
LangChain tests and results
Alright, now comes the exciting part! Let’s look at the tests and results.
Code to test LangChain chunks
This brief block of code below is how we can run our function for experimentation. I’ve added five experiments. This tutorial tests chunk strategies from 32 to 512 in length by powers of 2 with overlaps running from 4 to 64 also by powers of 2. To test, we loop through the list of tuples and call the function we wrote above.
chunking_tests = [(32, 4), (64, 8), (128, 16), (256, 32), (512, 64)]
for test in chunking_tests:
test_langchain_chunking(path, headers_to_split_on, test[0], test[1])
Here’s what the entire output looks like. Now let’s take a peek into individual outputs. Remember that our chosen example question is: "What makes a distinguished engineer?"
Length 32, overlap 4
Okay so from this, we can clearly see that 32 is too short. This sentence is entirely useless. “Is a Distinguished Engineer” is the most circular reasoning possible.
Length 64, overlap 8
64 and 8 isn’t much better from the start. It does give us an example of a distinguished engineer though. Werner Vogels, CTO of Amazon.
Length 128, overlap 16
At 128, we start to see more complete sentences. Less “engineer.” type words and responses. This is not bad, it manages to extract the piece about Werner Vogel and “Has achieved noteworthy technical, professional accomplishments while working as an engineer.” The last entry is actually from the principal engineer section.
One downside here is that we already see examples of these special characters like \xa0
and \n
pop up. This tells us that perhaps we’re going too far on the chunking length.
Length 256, overlap 32
I think this chunking length is definitely too long. It pulls the required entries, but also pulls entries from “Fellow”, “Principal Engineer”, and “Senior Staff Engineer”. The first entry is from Distinguished Engineer though, and it covers three points on it.
Chunk length 512, overlap 64
We already established that 256 is probably too long. However, this 512’s first pull is actually the entire section for distinguished engineers. Now we have a dilemma - do we want individual “lines” or “notes” or to pull an entire section? That depends on your use case.
Summary of experimenting with different chunking strategy
Cool, so, we saw five different text splitter strategies with a parameterized approach that highlights chunks size and chunks overlap strategies in this tutorial using langchain chunking python. One of the dilemmas we saw from just doing these simple 5 chunk strategies is between getting individual tidbits and an entire section back depending on chunk size. We saw that 128 was pretty good for getting individual “lines” or “notes” about distinguished engineers, but that 512 could get our entire section back.
However, 256 wasn’t that good.
These three data points tell us something about the text splitter. It’s not just that finding an ideal chunks size is difficult. It’s also a sign that you need to think about what you want from your responses when crafting your chunk sizes as well.
Note that we haven’t even gotten to testing out different overlaps. After learning and getting a good chunk strategy down, checking overlaps is the logical next step. Maybe we’ll cover it in a future tutorial, maybe with another library. Stay tuned!
Insights from Experimentation
These experiments highlight the nuanced relationship between chunk size and overlap. Smaller chunks excel in tasks requiring pinpoint accuracy, while larger chunks are better suited for questions demanding extensive context. Overlap, on the other hand, plays a pivotal role in balancing these objectives.
It’s worth emphasizing that the trade-offs observed here are not one-size-fits-all. The ideal chunking parameters depend heavily on your specific use case. Applications like conversational agents or quick lookup tools may benefit from smaller chunks for precise answers. On the other hand, research-intensive workflows, such as summarizing complex legal documents, require larger chunks to ensure comprehensive context.
In real-world scenarios, a hybrid approach can often provide the best results. By dynamically adjusting chunk sizes based on the user's query and intent, developers can strike a balance between efficiency and retrieval quality. Such approaches may involve adding layers of intelligent query routing or adaptive chunking pipelines tailored to user needs.
Future Considerations
The experiments conducted in this tutorial are just the beginning. Future explorations could examine hierarchical chunking strategies that combine different chunk sizes for multi-level segmentation. Additionally, expanding to multi-modal data retrieval, such as combining text and image embeddings, opens up possibilities for more complex use cases.
There’s also potential in experimenting with overlaps systematically to better understand how they influence retrieval across diverse datasets. Integrating other frameworks alongside LangChain could further refine chunking approaches and uncover new optimization techniques. For example, frameworks like Haystack offer additional retrieval capabilities that can complement LangChain's workflow.
Finally, incorporating user feedback into the retrieval process could lead to adaptive systems that learn and optimize chunking strategies over time. With more fine-tuned experimentation, it’s possible to establish robust, user-centric RAG pipelines.
Conclusion
Chunking is an indispensable component of retrieval workflows in RAG applications. This tutorial demonstrated the importance of carefully tuning chunk size and overlap, showing how different strategies influence retrieval outcomes.
By balancing trade-offs between context and precision, developers can optimize their systems for a variety of use cases. While this tutorial covered foundational experiments, the possibilities for further exploration are vast. Stay tuned for more advanced tutorials and insights into optimizing AI-powered retrieval systems.
Chunking References
Chunking, or text splitter strategies continue to evolve, so we have started to build a collection of these different strategies to take a look at and potentially implement in your application. Enjoy!
A Guide to Chunking Strategies for Retrieval Augmented Generation (RAG). We explored various facets of chunking strategies within Retrieval-Augmented Generation (RAG) systems in this guide.
A Beginner's Guide to Website Chunking and Embedding for Your RAG Applications. In this post, we'll explain how to extract content from a website and use it as context for LLMs in a RAG application. However, before doing so, we need to understand website fundamentals.
Exploring Three Key Strategies for Building Efficient Retrieval Augmented Generation (RAG). Retrieval Augmented Generation (RAG) is a useful technique for using your own data in an AI-powered Chatbot. This blog post will walk you through three key strategies to get the most out of RAG.
Pandas DataFrame: Chunking and Vectorizing with Milvus. If we store all of the data, including the chunk text and the embedding, inside of Pandas DataFrame, we can easily integrate and import them into the Milvus vector database.
- LangChain Overview and Why Chunking Matters
- Understanding the Challenges of Poor Chunking
- LangChain tests and results
- Summary of experimenting with different chunking strategy
- Chunking References
Content
Start Free, Scale Easily
Try the fully-managed vector database built for your GenAI applications.
Try Zilliz Cloud for FreeKeep Reading
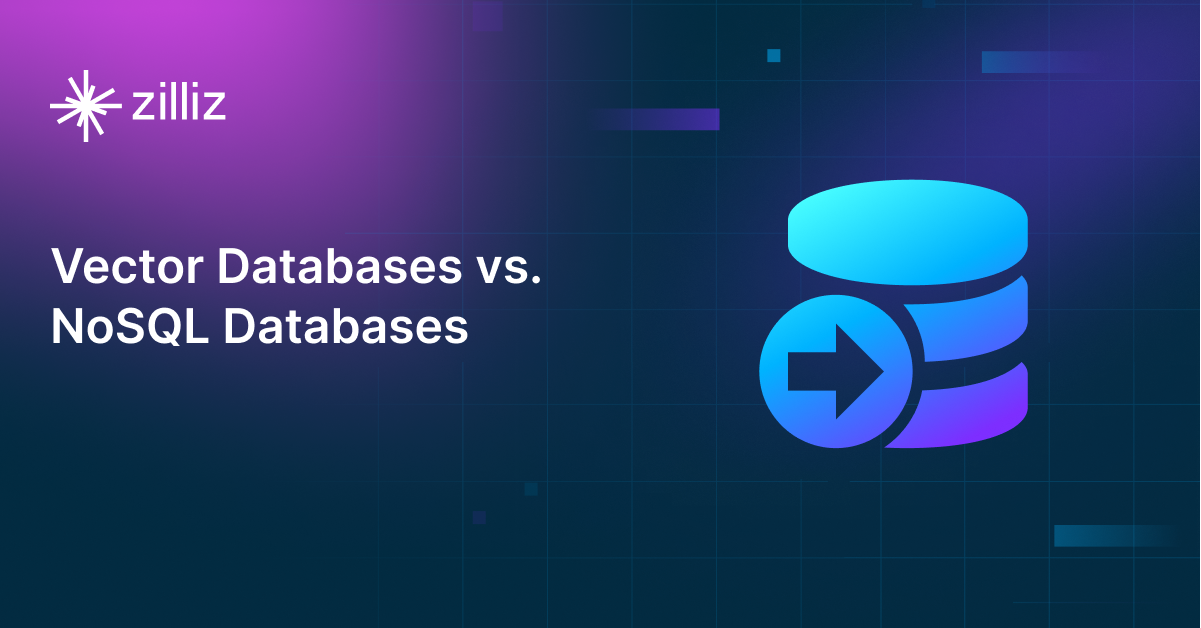
Vector Databases vs. NoSQL Databases
Use a vector database for AI-powered similarity search; use NoSQL databases for flexibility, scalability, and diverse non-relational data storage needs.
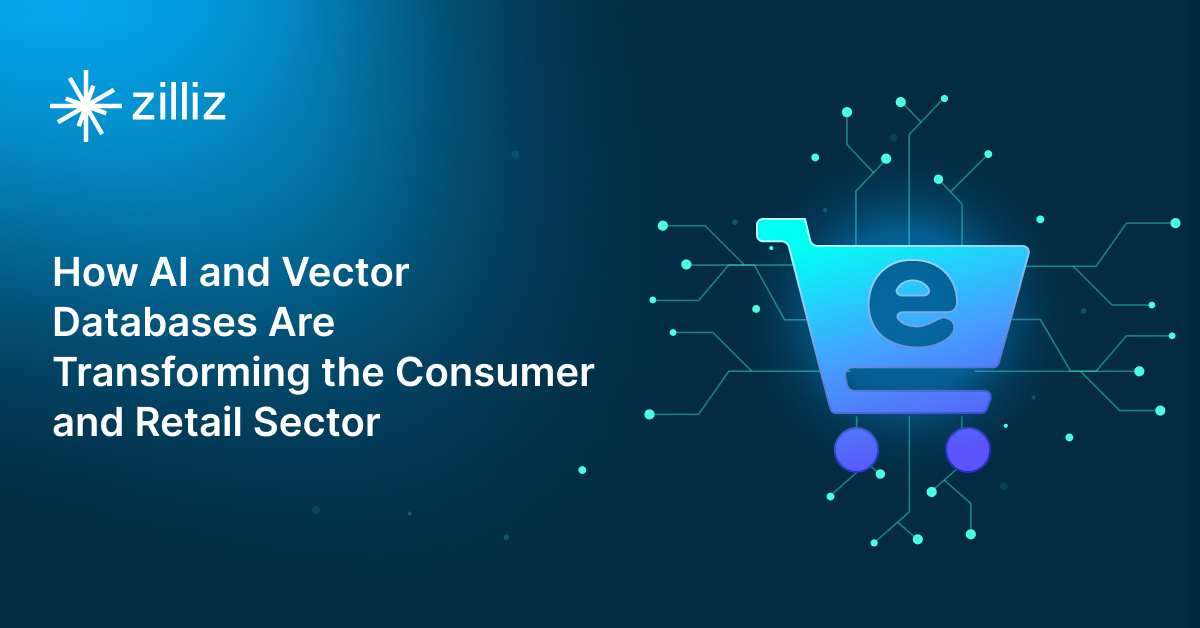
How AI and Vector Databases Are Transforming the Consumer and Retail Sector
AI and vector databases are transforming retail, enhancing personalization, search, customer service, and operations. Discover how Zilliz Cloud helps drive growth and innovation.
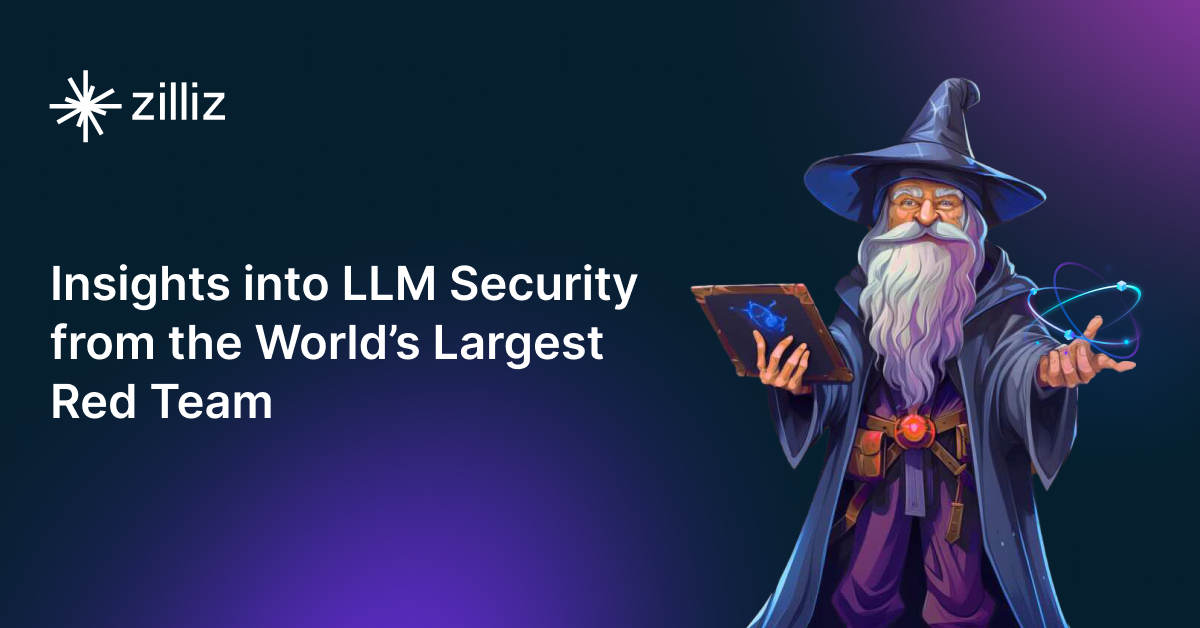
Insights into LLM Security from the World’s Largest Red Team
We will discuss how the Gandalf project revealed LLMs' vulnerabilities to adversarial attacks. Additionally, we will address the role of vector databases in AI security.