Annoy vs ScaNN: Choosing the Right Vector Search Tool for Your Application
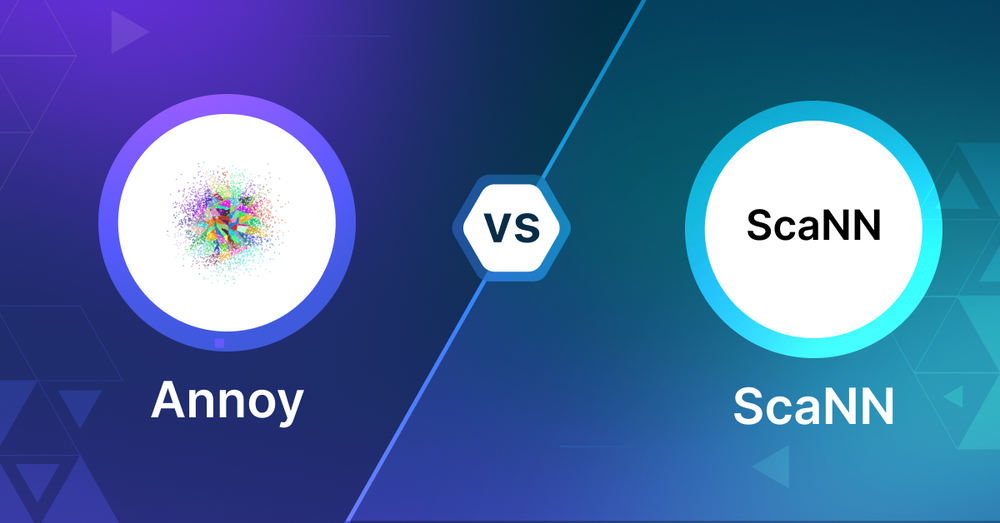
Introduction
Today, vector search has become a fundamental element powering various modern AI applications such as recommendation engines, image retrieval systems, and natural language processing (NLP) tasks. Unlike traditional search engines that rely on keyword matching, vector search allows us to retrieve information based on vector similarity, unlocking deeper insights from unstructured data like images, audio, and text embeddings.
Among the tools available for vector search, Annoy and ScaNN stand out as popular options. Each has its unique strengths and is optimized for different use cases. In this blog, we’ll explore the core features of Annoy and ScaNN, their differences, and the scenarios where one might be more suitable than the other. By the end, you’ll clearly understand which tool aligns best with your needs.
What is Vector Search?
Before diving into the specifics of Annoy and ScaNN, it's essential to understand vector search. Simply put, Vector search, or vector similarity search, finds the closest vectors (data points) in a high-dimensional space to a given query vector. These vectors are often generated by machine learning models to capture the essence of the unstructured data (e.g., the meaning of a sentence or the features of an image).
Unlike traditional databases, where searches are based on exact matches or filtering, vector search focuses on similarity. The goal is to find vectors that are "close" to each other based on a distance metric (such as Euclidean distance or cosine similarity). For instance, vectors can represent words or sentences in natural language processing (NLP), and vector search helps find the most semantically similar words or texts. In recommendation systems, vector search identifies items closest to a user's preferences. Vector searches also play a crucial part in retrieval augmented generation (RAG), a technique that augments the output of large language models (LLMs) by providing them with extra contextual information.
There are many solutions available on the market for performing vector searches, including:
- Vector search libraries such as Annoy and ScaNN.
- Purpose-built vector databases such as Milvus, Zilliz Cloud (fully managed Milvus)
- Lightweight vector databases such as Chroma and Milvus Lite.
- Traditional databases with vector search add-ons
What is Annoy? An Overview
Annoy (Approximate Nearest Neighbors Oh Yeah) is a lightweight open-source library developed by Spotify. It is specifically designed to handle large-scale, read-heavy vector searches. Its primary advantage lies in its minimal memory consumption and simplicity, making it ideal for static datasets that don't change frequently.
Annoy’s search algorithm is based on building multiple random projection trees that divide the vector space into smaller regions. This approach enables fast searches at the cost of accuracy since the results are approximate, not exact. This trade-off is acceptable for many applications because the speed benefits outweigh the small dip in precision.
Annoy is ideal for situations where memory efficiency is a priority. It allows you to store massive datasets on disk, enabling searches without loading the entire dataset into memory. However, this also means that adding or removing vectors requires rebuilding the entire index, which can be cumbersome if you have frequently changing data. Annoy also integrates easily with multiple programming languages like Python, C++, and Go, making it accessible to a broad range of developers.
In short, Annoy is a perfect fit for large, static datasets and fast, memory-efficient searches. However, it may not be the best option if your data needs frequent updates or requires high precision.
What is ScaNN? An Overview
ScaNN (Scalable Nearest Neighbors) is an open-source library developed by Google to perform fast, approximate nearest neighbor (ANN) searches, primarily for high-dimensional vector data. It is optimized for large-scale machine learning applications, where retrieving the closest vectors from a dataset is crucial.
ScaNN uses advanced techniques like partitioning, quantization, and asymmetric hashing to compress data and accelerate search processes, making it particularly well-suited for applications requiring a balance between speed and accuracy. It allows for customizable trade-offs depending on the requirements of the task at hand. One of its key strengths is the ability to integrate with TensorFlow, making it highly efficient for AI workflows where vector searches need to be fast and scalable.
ScaNN competes with libraries like Faiss (by Facebook), Annoy (by Spotify), and HNSWlib (Hierarchical Navigable Small World), which are also popular ANN search algorithms. ScaNN’s strength lies in its ability to integrate with TensorFlow and provide high-speed search while maintaining good precision.
Key Differences Between Annoy and ScaNN
Annoy and ScaNN are designed to solve the nearest neighbor search problem but use different approaches. Let’s explore their key differences in greater detail.
Search Methodology
Annoy and ScaNN rely on different underlying algorithms to perform vector searches, each with distinct trade-offs.
Annoy builds a forest of random projection trees to partition the vector space. When a query is made, it searches across multiple trees to find approximate nearest neighbors. This method is quick but sacrifices some accuracy for the sake of speed, making it suitable for use cases where "close enough" results are acceptable.
ScaNN, in contrast, combines partitioning, quantization, and asymmetric hashing to achieve fast and accurate searches. This allows it to narrow down the search space effectively and deliver more accurate results than Annoy. ScaNN’s methodology is particularly useful when precision is crucial, such as in certain machine learning tasks.
Data Handling
Annoy and ScaNN also handle data differently. Annoy is disk-based, meaning it can operate on datasets that exceed the available memory. This makes it highly scalable in terms of storage, though its performance might degrade as the dataset grows. Annoy is most effective when the data remains relatively static after the initial setup.
ScaNN is optimized for in-memory performance and focuses on managing dynamic datasets. It supports vector compression, allowing for better memory efficiency without compromising too much on accuracy. This makes ScaNN more flexible for applications that deal with constantly changing data or where updates to the dataset are frequent.
Scalability and Performance
In terms of performance, Annoy scales well when handling large, static datasets thanks to its disk-based architecture. However, because Annoy is built around approximate search, it may not always return the most accurate results, particularly as dataset size increases. This trade-off might not be an issue for applications where rough matches are acceptable.
ScaNN, however, is designed to handle massive datasets with both speed and precision. ScaNN’s ability to partition and quantize data means it can search across large datasets while maintaining high accuracy. However, it typically requires more computational resources than Annoy, so for very large-scale applications, you may need to invest in more powerful infrastructure.
Flexibility and Customization
Annoy’s customization options are limited to adjusting the number of trees and the search depth. While this can offer some control over the balance between accuracy and speed, Annoy doesn’t offer the fine-grained customization that ScaNN does.
ScaNN allows users to tweak various parameters related to speed and accuracy, offering more flexibility in optimizing searches for specific use cases. This makes it particularly useful when data or query patterns vary frequently, and performance needs to be fine-tuned based on real-world usage.
Integration and Ecosystem
Annoy is a simple and lightweight tool that integrates with several programming languages. It’s commonly used in recommendation systems and search engines, and because of its simplicity, it’s easy to plug into various applications without significant overhead.
ScaNN’s integration with TensorFlow gives it a powerful edge in machine learning workflows. If you’re already using TensorFlow to generate embeddings or other vector representations, ScaNN can be a natural fit, allowing for seamless integration without changing much of your existing pipeline.
Ease of Use
Annoy is widely regarded for its simplicity. Its lightweight API makes it easy to get started, even if you’re new to vector search. The learning curve is minimal, and you can quickly set up a search system without tweaking too many parameters.
ScaNN, while more powerful, comes with a steeper learning curve. You’ll need to spend some time understanding its various optimization options, and it may require more effort to integrate into your system if you’re not already working with machine learning frameworks like TensorFlow. However, for more complex applications where accuracy and performance are critical, this extra effort is well worth it.
Cost Considerations
Annoy is a cost-effective solution, especially if you’re working with limited computational resources. Its ability to store data on disk means you won’t need high-memory servers, and the approximate search results are often sufficient for many applications. This makes it ideal for projects where budget constraints are a consideration.
ScaNN’s superior performance comes at a cost. It requires more computational power and memory, particularly for very large datasets. If you're working on resource-heavy applications that demand both speed and precision, the investment in infrastructure will be higher.
Security Features
Neither Annoy nor ScaNN has built-in security features like encryption or access control. If security is a concern in your application, you’ll need to implement additional measures to protect your data, such as encryption during storage and transport and robust authentication mechanisms.
When to Choose Annoy
Annoy is better when your application requires a fast, approximate search and your dataset is too large to fit into memory. It’s ideal for use cases where data is relatively static and speed is more important than precision. For instance, if you’re building a recommendation engine or content-based filtering system, Annoy’s speed and simplicity will allow you to scale quickly while keeping costs low.
Annoy also excels in scenarios where performance doesn’t need to be constantly fine-tuned. If your dataset remains consistent over time and you can tolerate approximate results, Annoy is likely the more suitable option.
When to Choose ScaNN
ScaNN is the tool of choice for applications where accuracy and performance are paramount. It’s particularly well-suited for machine learning applications that involve embeddings, such as image search, document retrieval, or natural language processing. If your dataset is large and dynamic, and you need high-speed searches without sacrificing precision, ScaNN offers a more reliable solution.
Its integration with TensorFlow also makes it a strong contender for AI applications. ScaNN’s ability to seamlessly integrate will save you development time and effort if you're already working with a machine learning framework.
Comparing Vector Search Libraries and Purpose-built Vector Databases
Both vector search libraries like Annoy and ScaNN and purpose-built vector databases like Milvus aim to solve the similarity search problem for high-dimensional vector data, but they serve different roles.
Vector search libraries, like Annoy, ScaNN, HNSWlib, and Faiss, focus solely on the task of efficient nearest neighbor search. They offer lightweight, fast solutions for finding vectors similar to a query vector. They are often used in smaller, single-node environments or for applications with static or moderately sized datasets. However, they generally lack features for managing dynamic data, providing persistence, or scaling across distributed systems. Developers using these libraries typically need to manually handle data management, updates, and scaling.
On the other hand, purpose-built vector databases like Milvus and Zilliz Cloud (the managed Milvus) are comprehensive systems designed for large-scale vector data management. These databases go beyond simple vector search, offering features like persistent storage, real-time updates, distributed architecture, and advanced querying capabilities. They support dynamic datasets and can easily handle real-time applications where data is frequently updated. Additionally, vector databases often include integrated support for combining vector searches with traditional filtering and metadata queries, making them ideal for production environments requiring scalability, high availability, and more complex search functionalities.
- Check out the latest new features and enhancements of Zilliz Cloud: Zilliz Cloud Update: Migration Services, Fivetran Connectors, Multi-replicas, and More
When to Choose Each Vector Search Solution
Choose Vector Search Libraries if:
- You have a small to medium-sized, relatively static dataset.
- You prefer full control over indexing and search algorithms.
- You're embedding search in an existing system and can manage the infrastructure.
Choose Purpose-Built Vector Databases if:
- You need to scale to billions of vectors across distributed systems.
- Your dataset changes frequently, requiring real-time updates.
- You prefer managed solutions that handle storage, scaling, and query optimizations for you.
In summary, vector search libraries are best suited for simpler, smaller-scale use cases where speed and memory efficiency are priorities, but operational complexity is minimal. Purpose-built vector databases, by contrast, are designed for large-scale, production-grade systems that demand dynamic data handling, scalability, and ease of use, often providing significant operational benefits for developers managing complex applications.
Evaluating and Comparing Different Vector Search Solutions
OK, now we've learned the difference between different vector search solutions. The following questions are: how do you ensure your search algorithm returns accurate results and does so at lightning speed? How do you evaluate the effectiveness of different ANN algorithms, especially at scale?
To answer these questions, we need a benchmarking tool. Many such tools are available, and two emerge as the most efficient: ANN benchmarks and VectorDBBench.
ANN benchmarks
ANN Benchmarks (Approximate Nearest Neighbor Benchmarks) is an open-source project designed to evaluate and compare the performance of various approximate nearest neighbor (ANN) algorithms. It provides a standardized framework for benchmarking different algorithms on tasks such as high-dimensional vector search, allowing developers and researchers to measure metrics like search speed, accuracy, and memory usage across various datasets. By using ANN-Benchmarks, you can assess the trade-offs between speed and precision for algorithms like those found in libraries such as Faiss, Annoy, HNSWlib, and others, making it a valuable tool for understanding which algorithms perform best for specific applications.
ANN Benchmarks GitHub repository: https://github.com/erikbern/ann-benchmarks
ANN Benchmarks Website: https://ann-benchmarks.com/
VectorDBBench
VectorDBBench is an open-source benchmarking tool designed for users who require high-performance data storage and retrieval systems, particularly vector databases. This tool allows users to test and compare the performance of different vector database systems such as Milvus and Zilliz Cloud (the managed Milvus) using their own datasets, and determine the most suitable one for their use cases. VectorDBBench is written in Python and licensed under the MIT open-source license, meaning anyone can freely use, modify, and distribute it.
VectorDBBench GitHub repository: https://github.com/zilliztech/VectorDBBench
Take a quick look at the performance of mainstream vector databases on the VectorDBBench Leaderboard.
Techniques & Insights on VectorDB Evaluation:
Further Resources about VectorDB, GenAI, and ML
- Introduction
- What is Vector Search?
- What is Annoy? An Overview
- What is ScaNN? An Overview
- Key Differences Between Annoy and ScaNN
- When to Choose Annoy
- When to Choose ScaNN
- Comparing Vector Search Libraries and Purpose-built Vector Databases
- Evaluating and Comparing Different Vector Search Solutions
- Further Resources about VectorDB, GenAI, and ML
Content
Start Free, Scale Easily
Try the fully-managed vector database built for your GenAI applications.
Try Zilliz Cloud for FreeKeep Reading
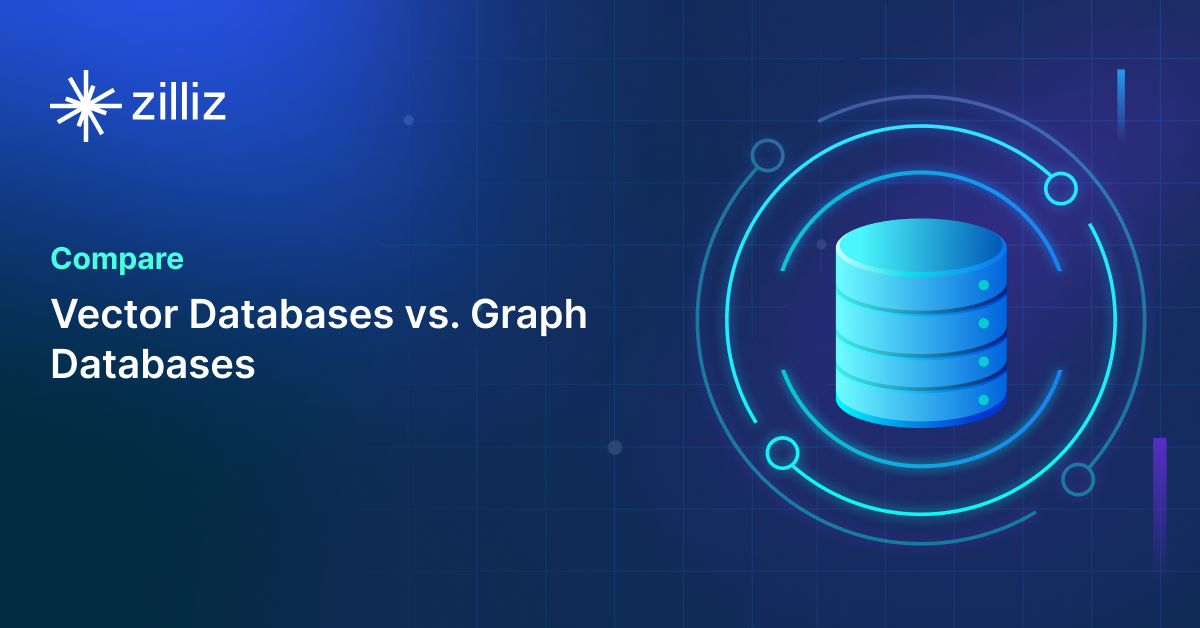
Vector Databases vs. Graph Databases
Use a vector database for AI-powered similarity search; use a graph database for complex relationship-based queries and network analysis.
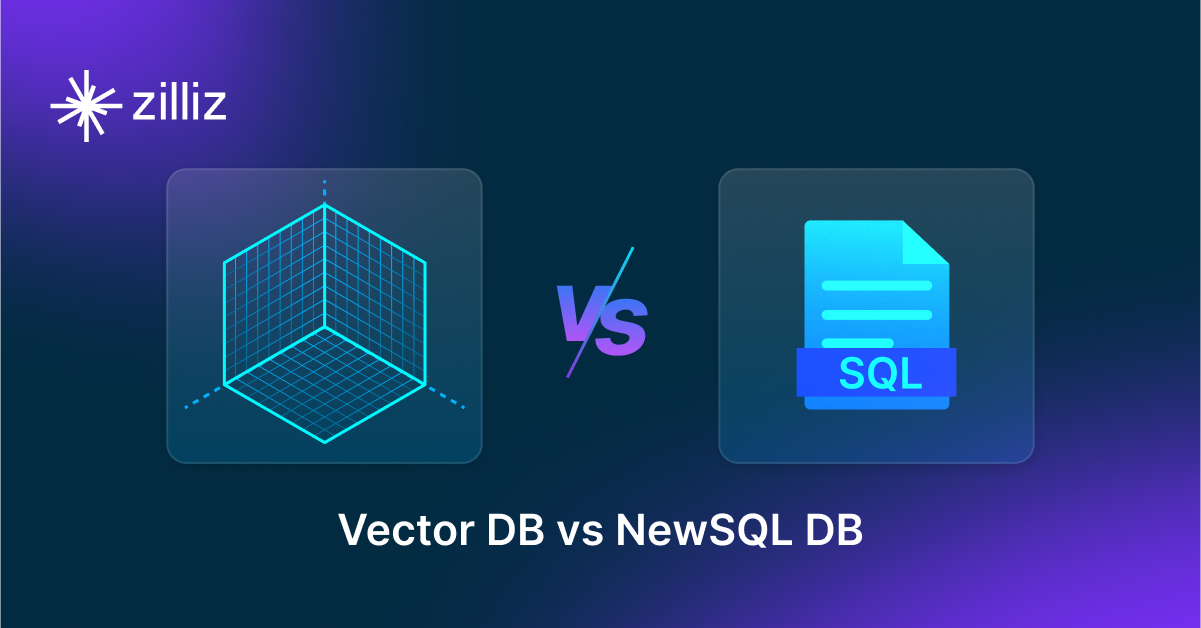
Vector Databases vs. NewSQL Databases
Use a vector database for AI-powered similarity search; use a NewSQL database for scalable transactional workloads requiring strong consistency and relational capabilities.
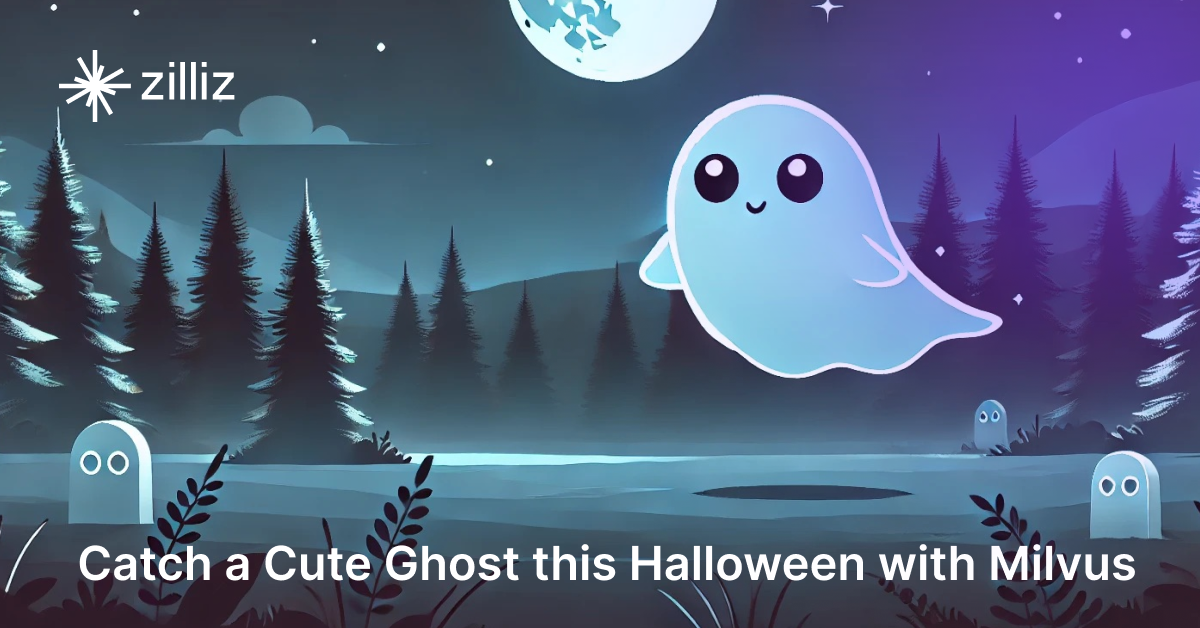
Catch a Cute Ghost this Halloween with Milvus
Run ghastly multimodal analytics and Retrieval Augmented Generation with our "ghosts" collections in the open-source Milvus vector database.
The Definitive Guide to Choosing a Vector Database
Overwhelmed by all the options? Learn key features to look for & how to evaluate with your own data. Choose with confidence.