LanceDB vs Deep Lake Choosing the Right Vector Database for Your AI Apps
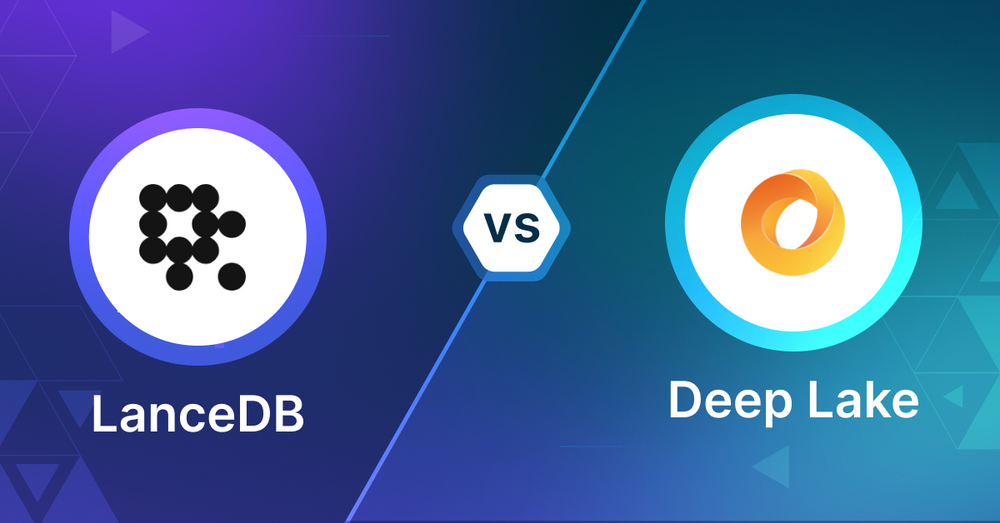
What is a Vector Database?
Before we compare LanceDB and Deep Lake, let's first explore the concept of vector databases.
A vector database is specifically designed to store and query high-dimensional vectors, which are numerical representations of unstructured data. These vectors encode complex information, such as the semantic meaning of text, the visual features of images, or product attributes. By enabling efficient similarity searches, vector databases play a pivotal role in AI applications, allowing for more advanced data analysis and retrieval.
Common use cases for vector databases include e-commerce product recommendations, content discovery platforms, anomaly detection in cybersecurity, medical image analysis, and natural language processing (NLP) tasks. They also play a crucial role in Retrieval Augmented Generation (RAG), a technique that enhances the performance of large language models (LLMs) by providing external knowledge to reduce issues like AI hallucinations.
There are many types of vector databases available in the market, including:
- Purpose-built vector databases such as Milvus, Zilliz Cloud (fully managed Milvus)
- Vector search libraries such as Faiss and Annoy.
- Lightweight vector databases such as Chroma and Milvus Lite.
- Traditional databases with vector search add-ons capable of performing small-scale vector searches.
LanceDB is a serverless vector database and Deep Lake is a data lake optimized for vector embeddings. This post compares their vector search capabilities.
LanceDB: Overview and Core Technology
LanceDB is an open-source vector database for AI that stores, manages, queries and retrieves embeddings from large-scale multi-modal data. Built on Lance, an open-source columnar data format, LanceDB has easy integration, scalability and cost effectiveness. It can run embedded in existing backends, directly in client applications or as a remote serverless database so it’s versatile for many use cases.
Vector search is at the heart of LanceDB. It supports both exhaustive k-nearest neighbors (kNN) search and approximate nearest neighbor (ANN) search using an IVF_PQ index. This index divides the dataset into partitions and applies product quantization for efficient vector compression. LanceDB also has full-text search and scalar indices to boost search performance across different data types.
LanceDB supports various distance metrics for vector similarity, including Euclidean distance, cosine similarity and dot product. The database allows hybrid search combining semantic and keyword-based approaches and filtering on metadata fields. This enables developers to build complex search and recommendation systems.
The primary audience for LanceDB are developers and engineers working on AI applications, recommendation systems or search engines. Its Rust-based core and support for multiple programming languages makes it accessible to a wide range of technical users. LanceDB’s focus on ease of use, scalability and performance makes it a great tool for those dealing with large scale vector data and looking for efficient similarity search solutions.
DeepLake: Overview and Core Technology
Deep Lake is a specialized database built for handling vector and multimedia data—such as images, audio, video, and other unstructured types—widely used in AI and machine learning. It functions as both a data lake and a vector store:
- As a Data Lake: Deep Lake supports the storage and organization of unstructured data (images, audio, videos, text, and formats like NIfTI for medical imaging) in a version-controlled format. This setup enhances performance in deep learning tasks. It enables fast querying and visualization of datasets, making it easier to create high-quality training sets for AI models.
- As a Vector Store: Deep Lake is designed for storing and searching vector embeddings and related metadata (e.g., text, JSON, images). Data can be stored locally, in your cloud environment, or on Deep Lake’s managed storage. It integrates seamlessly with tools like LangChain and LlamaIndex, simplifying the development of Retrieval Augmented Generation (RAG) applications.
Deep Lake uses the Hierarchical Navigable Small World (HNSW) index, based on the Hnswlib package with added optimizations, for Approximate Nearest Neighbor (ANN) search. This allows querying over 35 million embeddings in less than 1 second. Unique features include multi-threading for faster index creation and memory-efficient management to reduce RAM usage.
By default, Deep Lake uses linear embedding search for datasets with up to 100,000 rows. For larger datasets, it switches to ANN to balance accuracy and performance. The API allows users to adjust this threshold as needed.
Although Deep Lake’s index isn't used for combined attribute and vector searches (which currently rely on linear search), upcoming updates will address this limitation to improve its functionality further.
Deep Lake as a Vector Store: Deep Lake provides a robust solution for storing and searching vector embeddings and their associated metadata, including text, JSON, images, audio, and video files. You can store data locally, in your preferred cloud environment, or on Deep Lake's managed storage. Deep Lake also offers seamless integration with tools like LangChain and LlamaIndex, allowing developers to easily build Retrieval Augmented Generation (RAG) applications.
Key Differences
Search Performance and Methodology
LanceDB uses IVF_PQ (Inverted File with Product Quantization) as its core search algorithm, splitting datasets into partitions and compressing vectors for faster retrieval. For smaller datasets, it performs exhaustive k-nearest neighbors search to maintain accuracy. The system supports various distance metrics including Euclidean, cosine similarity, and dot product, enabling precise similarity matching based on use case requirements.
Deep Lake implements HNSW (Hierarchical Navigable Small World) through an optimized version of Hnswlib, capable of querying over 35 million embeddings in under one second. It uses linear search by default for datasets under 100,000 rows, with configurable thresholds. The multi-threading approach for index creation helps balance speed and resource usage.
Data Management
LanceDB builds on the Lance columnar format, providing efficient storage and retrieval for both structured and unstructured data. Its hybrid search capabilities let developers combine vector similarity searches with metadata filtering, making it effective for complex queries that need both semantic and traditional search capabilities.
Deep Lake handles multimedia data like images, audio, and video alongside vector embeddings. Its version control system tracks changes to datasets, making it suitable for machine learning workflows. The current limitation is that combined attribute and vector searches rely on linear search, though updates are planned to address this.
Deployment and Integration
LanceDB provides three main deployment options: embedding in existing backends, direct integration in client applications, or setup as a serverless database. This flexibility lets teams choose the most suitable architecture for their specific needs, whether it's a lightweight embedded instance or a full serverless deployment.
Deep Lake supports local storage, cloud deployment, and managed storage services. Its integration with LangChain and LlamaIndex makes it particularly strong for RAG applications. The system handles data storage across local environments, custom cloud setups, or Deep Lake's managed infrastructure.
Practical Usage Considerations
LanceDB prioritizes simplicity and cost-effectiveness. Its Rust-based core supports Python, JavaScript, and other languages, making it accessible for diverse development teams. The focus on lightweight deployment options helps reduce operational overhead.
Deep Lake excels in managing large multimedia datasets with version control. Its architecture suits machine learning pipelines and RAG applications. The system offers comprehensive dataset visualization and management tools, though this can increase complexity compared to simpler vector stores.
Cost Structure
LanceDB follows an open-source model with self-hosting options. Organizations can deploy and scale it on their infrastructure, potentially reducing costs for teams with existing hardware resources.
Deep Lake offers both self-hosted and managed options. The managed service costs vary based on storage volume and computation needs. While this might increase direct costs, it can reduce operational overhead and maintenance requirements.
LanceDB
Choose LanceDB for lightweight vector search with hybrid querying, embedded deployment or when cost and ease of integration are key, especially when you need metadata filtering alongside vector search.
Deep Lake
Choose Deep Lake for large multimedia datasets, machine learning pipelines that need version control or RAG applications that benefit from LangChain/LlamaIndex, especially when working with images, audio and video.
Conclusion
LanceDB stands out for its efficient IVF_PQ search, columnar data format, and flexible deployment options, while Deep Lake excels in multimedia data handling, version control, and ML tool integration. Your choice should align with specific needs: LanceDB for lightweight, cost-effective vector search with strong hybrid capabilities, or Deep Lake for comprehensive multimedia data management and ML pipeline integration.
Read this to get an overview of LanceDB and Deep Lake but to evaluate these you need to evaluate based on your use case. One tool that can help with that is VectorDBBench, an open-source benchmarking tool for vector database comparison. In the end, thorough benchmarking with your own datasets and query patterns will be key to making a decision between these two powerful but different approaches to vector search in distributed database systems.
Using Open-source VectorDBBench to Evaluate and Compare Vector Databases on Your Own
VectorDBBench is an open-source benchmarking tool for users who need high-performance data storage and retrieval systems, especially vector databases. This tool allows users to test and compare different vector database systems like Milvus and Zilliz Cloud (the managed Milvus) using their own datasets and find the one that fits their use cases. With VectorDBBench, users can make decisions based on actual vector database performance rather than marketing claims or hearsay.
VectorDBBench is written in Python and licensed under the MIT open-source license, meaning anyone can freely use, modify, and distribute it. The tool is actively maintained by a community of developers committed to improving its features and performance.
Download VectorDBBench from its GitHub repository to reproduce our benchmark results or obtain performance results on your own datasets.
Take a quick look at the performance of mainstream vector databases on the VectorDBBench Leaderboard.
Read the following blogs to learn more about vector database evaluation.
Further Resources about VectorDB, GenAI, and ML
- What is a Vector Database?
- LanceDB: Overview and Core Technology
- DeepLake: Overview and Core Technology
- Key Differences
- LanceDB
- Deep Lake
- Conclusion
- Using Open-source VectorDBBench to Evaluate and Compare Vector Databases on Your Own
- Further Resources about VectorDB, GenAI, and ML
Content
Start Free, Scale Easily
Try the fully-managed vector database built for your GenAI applications.
Try Zilliz Cloud for FreeKeep Reading
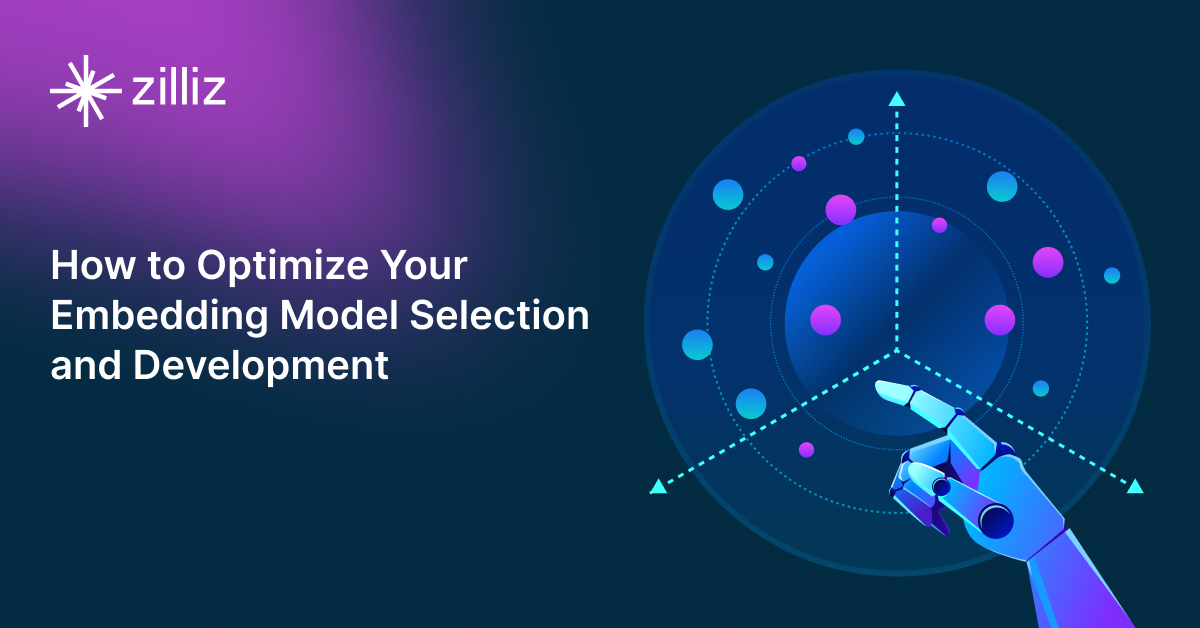
Optimizing Embedding Model Selection with TDA Clustering: A Strategic Guide for Vector Databases
Discover how Topological Data Analysis (TDA) reveals hidden embedding model weaknesses and helps optimize vector database performance.
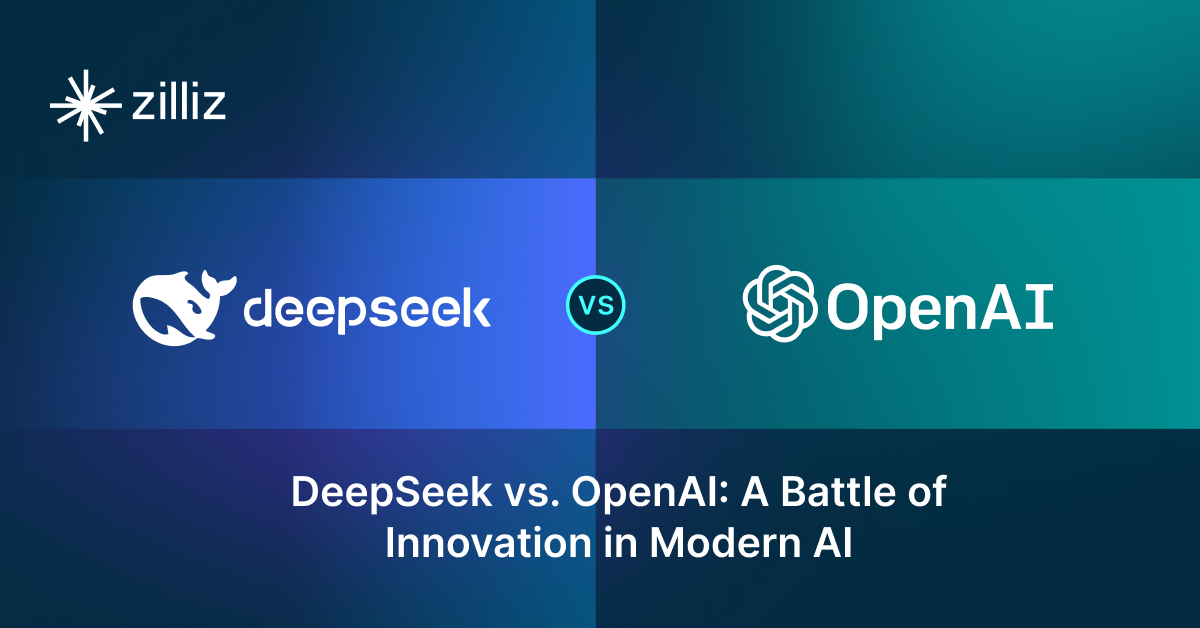
DeepSeek vs. OpenAI: A Battle of Innovation in Modern AI
Compare OpenAI's o1 and o3-mini with DeepSeek R1's open-source alternative. Discover which AI model offers the best balance of reasoning capabilities and cost efficiency.
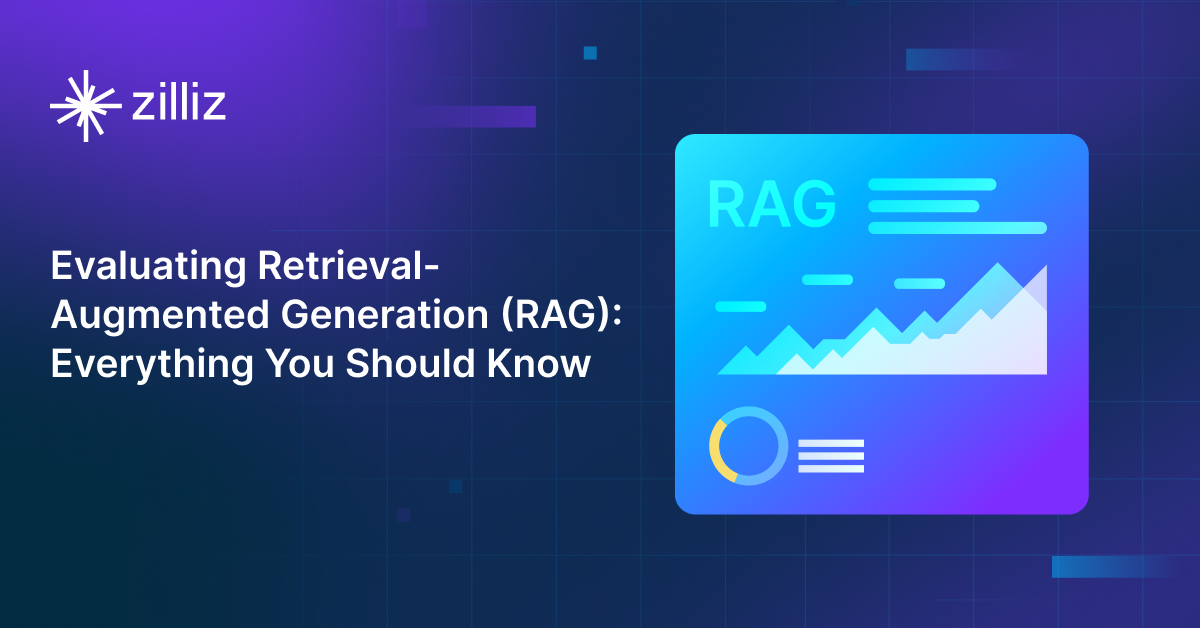
Evaluating Retrieval-Augmented Generation (RAG): Everything You Should Know
An overview of various RAG pipeline architectures, retrieval and evaluation frameworks, and examples of biases and failures in LLMs.
The Definitive Guide to Choosing a Vector Database
Overwhelmed by all the options? Learn key features to look for & how to evaluate with your own data. Choose with confidence.