Apache Cassandra vs. Vald: Choosing the Right Vector Database for Your AI Applications
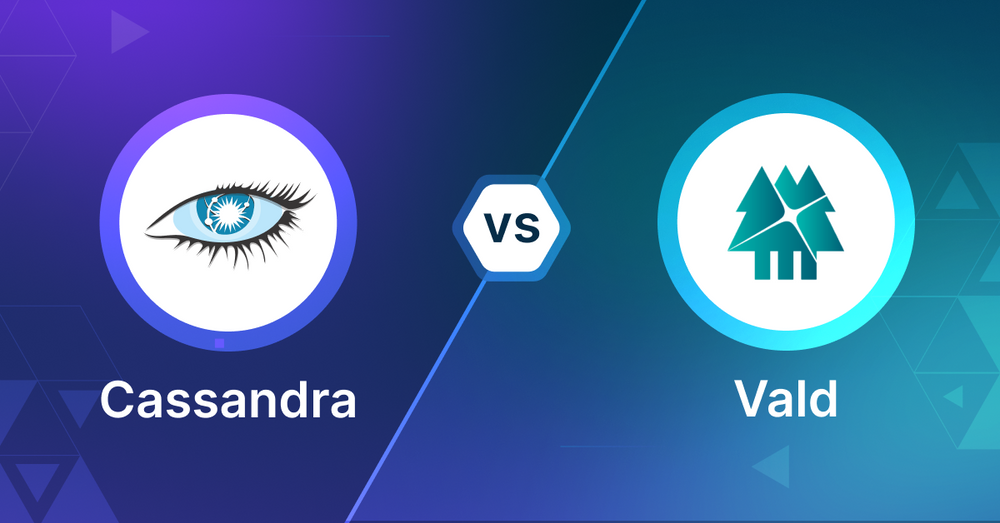
As AI-driven applications become more prevalent, developers and engineers face the challenge of selecting the right database to handle vector data efficiently. Two popular options in this space are Apache Cassandra and Vald. This article compares these technologies to help you make an informed decision for your vector database needs.
What is a Vector Database?
Before we compare Apache Cassandra and Vald, let's first explore the concept of vector databases. A vector database is specifically designed to store and query high-dimensional vectors, which are numerical representations of unstructured data. These vectors encode complex information, such as text's semantic meaning, images' visual features, or product attributes. By enabling efficient similarity searches, vector databases play a pivotal role in AI applications, allowing for more advanced data analysis and retrieval.
Vector databases are adopted in many use cases, including e-commerce product recommendations, content discovery platforms, anomaly detection in cybersecurity, medical image analysis, and natural language processing (NLP) tasks. They also play a crucial role in Retrieval Augmented Generation (RAG), a technique that enhances the performance of large language models (LLMs) by providing external knowledge to reduce issues like AI hallucinations.
There are many types of vector databases available in the market, including:
Purpose-built vector databases such as Milvus, Zilliz Cloud (fully managed Milvus)
Lightweight vector databases such as Chroma and Milvus Lite.
Traditional databases with vector search add-ons capable of performing small-scale vector searches.
Cassandra and Vald represent different approaches to vector databases. Cassandra is a traditional database that has evolved to include vector search capabilities and Vald, on the other hand, is a purpose-built vector database. It was designed from the ground up to handle vector data and perform similarity searches efficiently. As a specialized solution, Vald focuses exclusively on vector operations and is optimized for tasks like similarity search and recommendations.
##Apache Cassandra: Overview and Core Technology
Apache Cassandra is an open-source, distributed NoSQL database known for its scalability and availability. Cassandra's features include a masterless architecture for availability, scalability, tunable consistency, and a flexible data model. With the release of Cassandra 5.0, it now supports vector embeddings and vector similarity search through its Storage-Attached Indexes (SAI) feature. While this integration allows Cassandra to handle vector data, it's important to note that vector search is implemented as an extension of Cassandra's existing architecture rather than a native feature.
Cassandra's vector search functionality is built on its existing architecture. It allows users to store vector embeddings alongside other data and perform similarity searches. This integration enables Cassandra to support AI-driven applications while maintaining its strengths in handling large-scale, distributed data.
A key component of Cassandra's vector search is the use of Storage-Attached Indexes (SAI). SAI is a highly-scalable and globally-distributed index that adds column-level indexes to any vector data type column. It provides high I/O throughput for databases to use Vector Search as well as other search indexing. SAI offers extensive indexing functionality, capable of indexing both queries and content (including large inputs like documents, words, and images) to capture semantics.
Vector Search is the first instance of validating the extensibility of SAI, leveraging its new modularity. This combination of Vector Search and SAI enhances Cassandra's capabilities in handling AI and machine learning workloads, making it a strong contender in the vector database space.
Vald: Overview and Core Technology
Vald is a powerful tool for searching through huge amounts of vector data really fast. It's built to handle billions of vectors and can easily grow as your needs get bigger. The cool thing about Vald is that it uses a super quick algorithm called NGT to find similar vectors.
One of Vald's best features is how it handles indexing. Usually, when you're building an index, everything has to stop. But Vald is smart - it spreads the index across different machines, so searches can keep happening even while the index is being updated. Plus, Vald automatically backs up your index data, so you don't have to worry about losing everything if something goes wrong.
Vald is great at fitting into different setups. You can customize how data goes in and out, making it work well with gRPC. It's also built to run smoothly in the cloud, so you can easily add more computing power or memory when you need it. Vald spreads your data across multiple machines, which helps it handle huge amounts of information.
Another neat trick Vald has is index replication. It stores copies of each index on different machines. This means if one machine has a problem, your searches can still work fine. Vald automatically balances these copies, so you don't have to worry about it. All of this makes Vald a solid choice for developers who need to search through tons of vector data quickly and reliably.
Key Differences
Search Methodology
Cassandra and Vald employ different approaches to search. Cassandra has integrated vector similarity search into its existing architecture using Storage-Attached Indexes (SAI), which allows it to perform vector searches alongside its traditional database operations. Vald, in contrast, is built from the ground up for vector search, utilizing the NGT algorithm specifically designed for fast approximate nearest neighbor searches in dense vector spaces. This fundamental difference in design philosophy results in distinct search capabilities and performance characteristics.
Data Handling
Cassandra's roots as a NoSQL database allow it to handle structured and semi-structured data efficiently, with the added capability to store and search vector embeddings alongside other data types. This versatility makes Cassandra suitable for a wide range of applications that require both traditional data storage and vector search capabilities. Vald, however, is primarily focused on handling and searching vector data. It is optimized for high-dimensional feature vectors, making it particularly well-suited for applications that deal exclusively or primarily with vector representations.
Scalability and Performance
Both systems offer robust scalability, but through different mechanisms. Cassandra provides a masterless architecture that ensures high availability and scalability, with the added benefit of tunable consistency. This allows users to balance between consistency and performance based on their specific needs. Vald takes a different approach, built from the ground up for high scalability in vector search operations. It distributes vector indexes across multiple agents and supports horizontal scaling of both memory and CPU resources, allowing it to efficiently handle billions of vectors.
Flexibility and Customization
Cassandra offers flexibility through its established NoSQL data model, allowing users to adapt it to various use cases within its database paradigm. The addition of vector search capabilities extends this flexibility to AI-driven applications. Vald, being more specialized, offers high customizability in areas directly related to vector search operations. It provides extensive options for customizing ingress/egress filtering and integrates well with gRPC interfaces, allowing users to tailor its functionality to specific vector search requirements.
When to Choose Vald or Apache Cassandra
Cassandra: Cassandra is a great choice when you need a powerful database that can now handle vector search too. It's perfect for big projects where you're dealing with tons of data spread across many machines. If you're already using Cassandra for other stuff and want to add some AI features that need vector search, it's super convenient. Cassandra is also awesome if you need to be able to tweak how consistent your data is across all your machines. So, if you're running a big app that needs both regular data storage and some vector searching, Cassandra could be your go-to. Vald: Vald is the way to go when your main focus is searching through massive amounts of vector data really fast. If you're building an app that's all about finding similar things quickly - like recommending products or finding similar images - Vald is designed just for that. It's great if you need to keep searching even while you're updating your data, thanks to its clever indexing system. Vald is also a good pick if you want something that can easily grow as your data gets bigger, especially if you're running things in the cloud. If you're dealing with billions of vectors and need lightning-fast search results, Vald is built to handle that kind of workload.
Conclusion
In conclusion, Cassandra and Vald each have their own strengths that make them suitable for different situations. Cassandra shines as a versatile NoSQL database with added vector search capabilities, making it ideal for applications that need to handle various data types alongside vector operations. Its distributed architecture and tunable consistency offer robust scalability for large-scale deployments. Vald, on the other hand, is a specialized powerhouse for high-performance vector search, excelling in situations that demand rapid similarity searches across billions of vectors. Its distributed indexing and real-time update capabilities make it particularly well-suited for dynamic, vector-centric applications. When choosing between these technologies, it's important to consider your specific use case, the types of data you're working with, and your performance requirements. If you need a general-purpose database with vector search abilities, Cassandra might be the way to go. But if your primary focus is on fast, scalable vector search operations, Vald could be the better fit. Always evaluate your project's unique needs to make the best choice.
While this article provides an overview of Cassandra and Vald, it's key to evaluate these databases based on your specific use case. One tool that can assist in this process is VectorDBBench, an open-source benchmarking tool designed for comparing vector database performance. Ultimately, thorough benchmarking with specific datasets and query patterns will be essential in making an informed decision between these two powerful, yet distinct, approaches to vector search in distributed database systems.
Using Open-source VectorDBBench to Evaluate and Compare Vector Databases on Your Own
VectorDBBench is an open-source benchmarking tool designed for users who require high-performance data storage and retrieval systems, particularly vector databases. This tool allows users to test and compare the performance of different vector database systems such as Milvus and Zilliz Cloud (the managed Milvus) using their own datasets and determine the most suitable one for their use cases. Using VectorDBBench, users can make informed decisions based on the actual vector database performance rather than relying on marketing claims or anecdotal evidence.
VectorDBBench is written in Python and licensed under the MIT open-source license, meaning anyone can freely use, modify, and distribute it. The tool is actively maintained by a community of developers committed to improving its features and performance.
Download VectorDBBench from its GitHub repository to reproduce our benchmark results or obtain performance results on your own datasets.
Take a quick look at the performance of mainstream vector databases on the VectorDBBench Leaderboard.
Read the following blogs to learn more about vector database evaluation.
Further Resources about VectorDB, GenAI, and ML
- What is a Vector Database?
- Vald: Overview and Core Technology
- Key Differences
- When to Choose Vald or Apache Cassandra
- Conclusion
- Using Open-source VectorDBBench to Evaluate and Compare Vector Databases on Your Own
- Further Resources about VectorDB, GenAI, and ML
Content
Start Free, Scale Easily
Try the fully-managed vector database built for your GenAI applications.
Try Zilliz Cloud for FreeKeep Reading
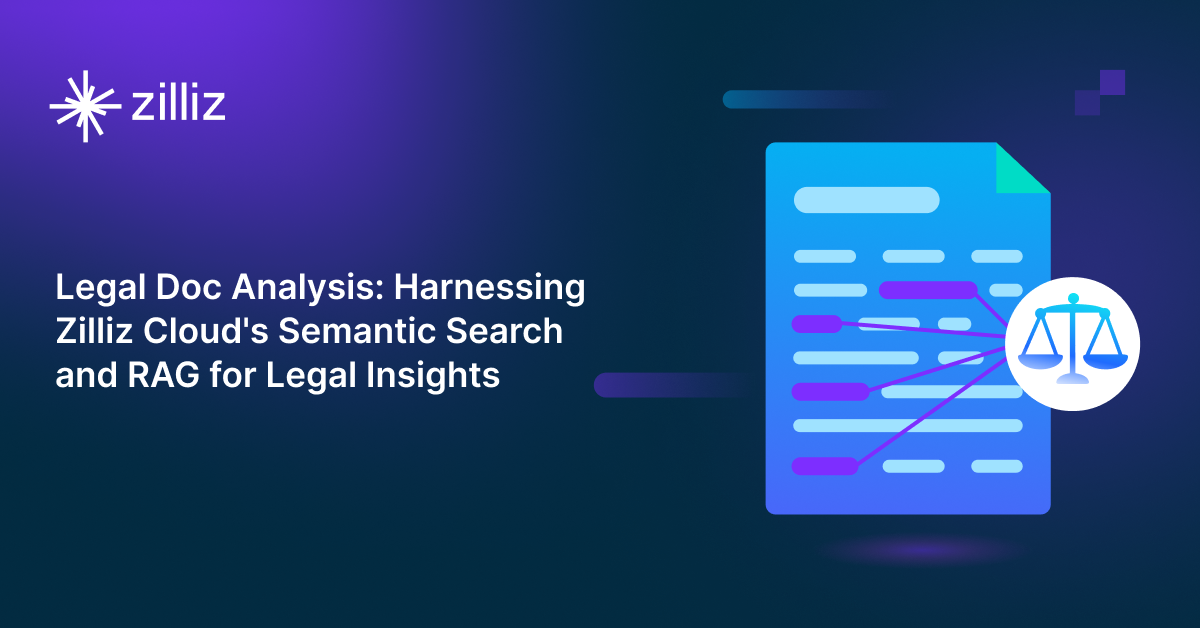
Legal Document Analysis: Harnessing Zilliz Cloud's Semantic Search and RAG for Legal Insights
Zilliz Cloud transforms legal document analysis with AI-driven Semantic Search and Retrieval-Augmented Generation (RAG). By combining keyword and vector search, it enables faster, more accurate contract analysis, case law research, and regulatory tracking.
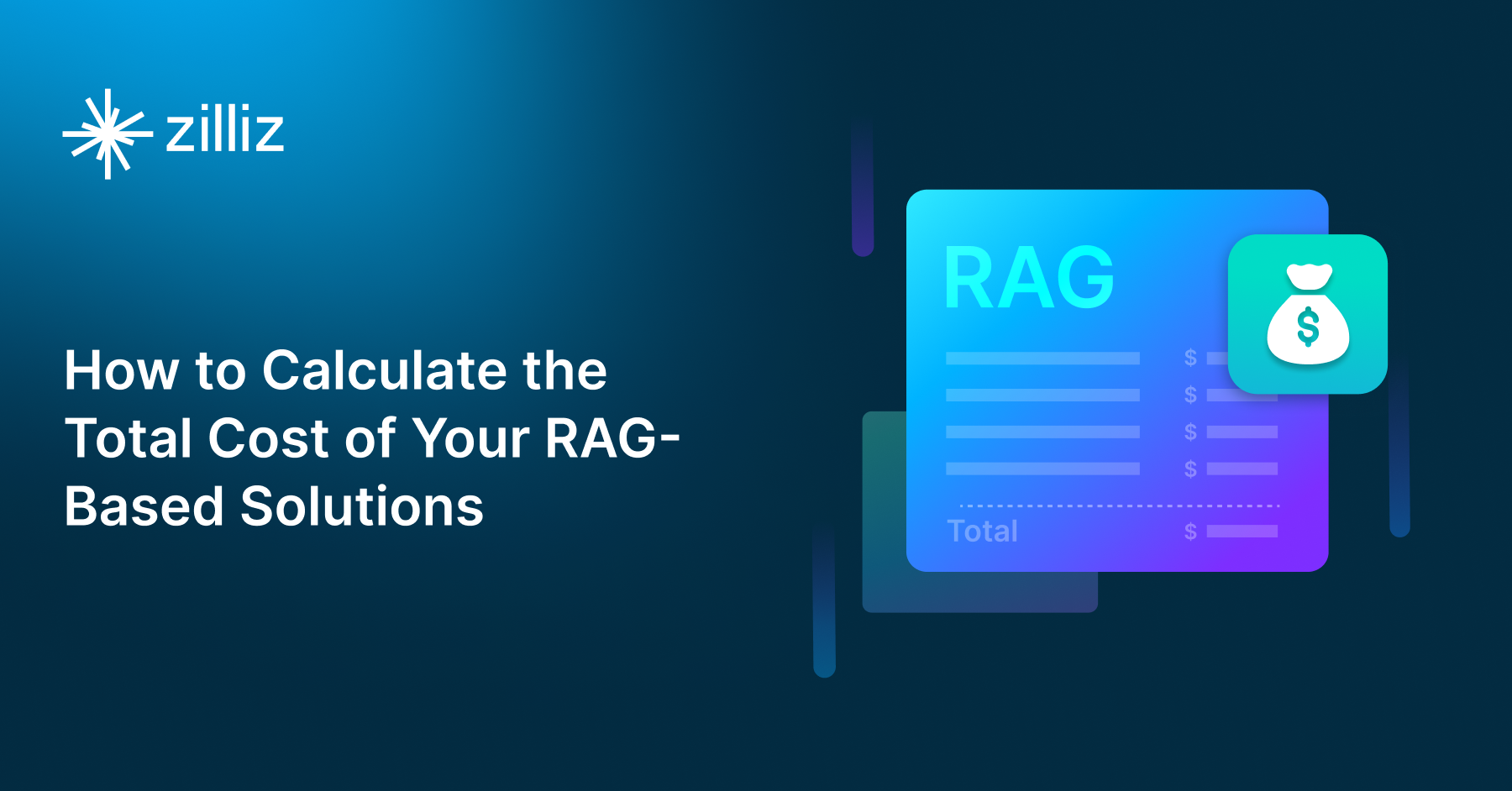
How to Calculate the Total Cost of Your RAG-Based Solutions
In this guide, we’ll break down the main components of RAG costs, show you how to calculate these expenses using the Zilliz RAG Cost Calculator, and explore strategies to manage spending efficiently.
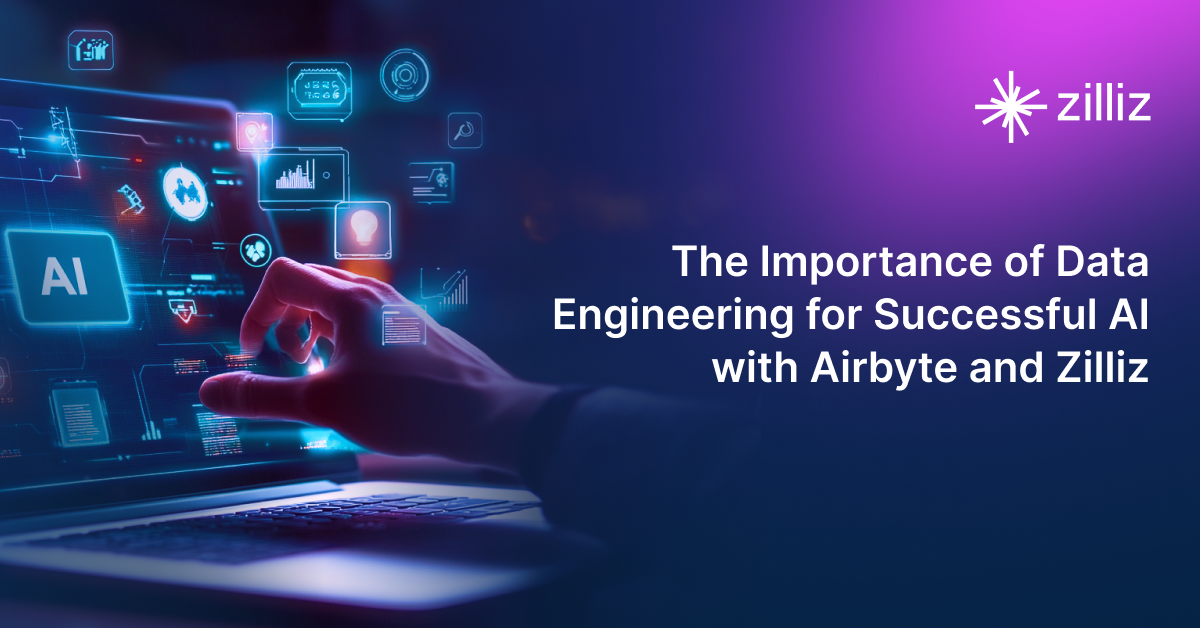
The Importance of Data Engineering for Successful AI with Airbyte and Zilliz
Learn how data engineering can resolve common challenges associated with deploying and scaling effective AI usage.
The Definitive Guide to Choosing a Vector Database
Overwhelmed by all the options? Learn key features to look for & how to evaluate with your own data. Choose with confidence.