Customers
Discover how industry leaders and technology pioneers leverage Milvus & Zilliz Cloud to power up their applications and accelerate business growth.
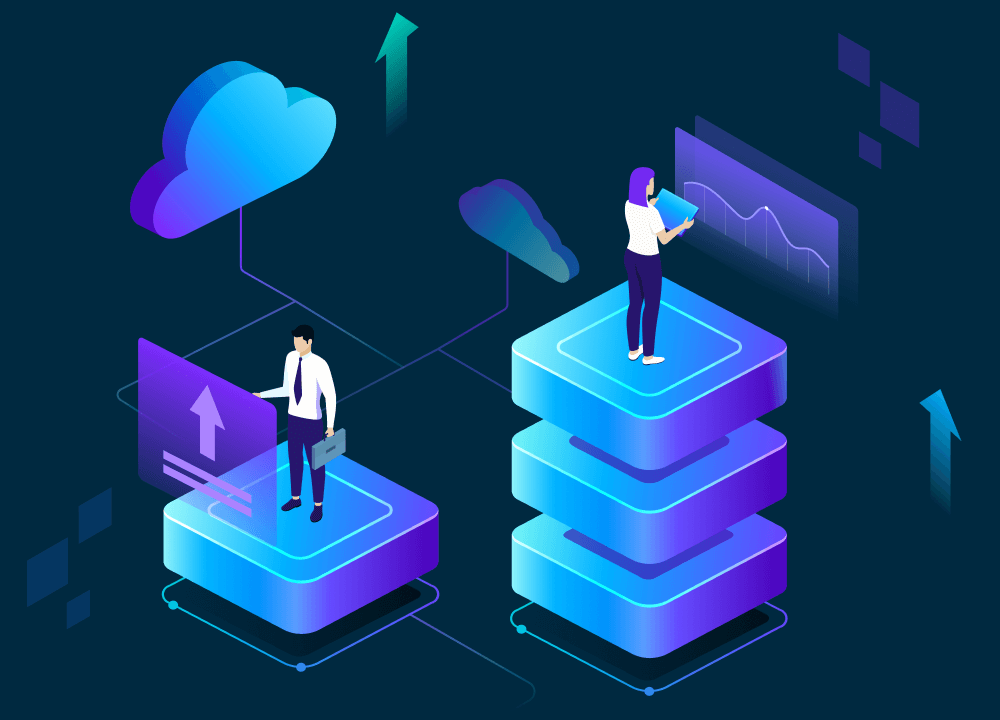
250M+
Vectors
High-Performance
Retrieval at Scale
Cost-Efficient
Serverless Deployment in Production
3 billion vectors
Managed across millions of documents
Semantic Search Architecture
Connecting concepts across documents even when exact terminology differs.
60-80%
Time saved on consuming, digesting, and identifying relevant data points
Legal Tech
Supercharging AI Agents: How Rexera Transforms Real Estate Closings with Zilliz Cloud
Read Now40% increase
in retrieval accuracy through hybrid search
50% Lower Cost
by eliminating Elasticsearch and switching from self-hosted vector databases to Zilliz Cloud’s fully managed platform
Real-time transaction processing
is enabled by Zilliz Cloud's exceptional latency performance
Internet Services
Beatoven.ai Switches from Pinecone to Zilliz Cloud to Fuel AI-Driven Music Generation
Read Now6x
Cost Savings without Performance Loss
Smooth
Migration with Minimal Downtime
2-3 seconds
Overall Improvement in the Generation Time After Switching to Zilliz Cloud
80%
Reduction in Data Collection Costs
~$1.4M
Reduction in Annual Storage Costs
Millisecond-level
Retrieval for Billions of Data Points with a Scalable Architecture
Entertainment
Why Dopple Labs Chose Zilliz Cloud over Pinecone for Secure and High-Performance Vector Searches
Read NowA Pinecone alternative
with granular control, effective scaling, and high performance
A Billion scale
vector data storage and retrieval
Open Source
for enhanced ML & VectorDB performance