How to Build RAG with Milvus, QwQ-32B and Ollama
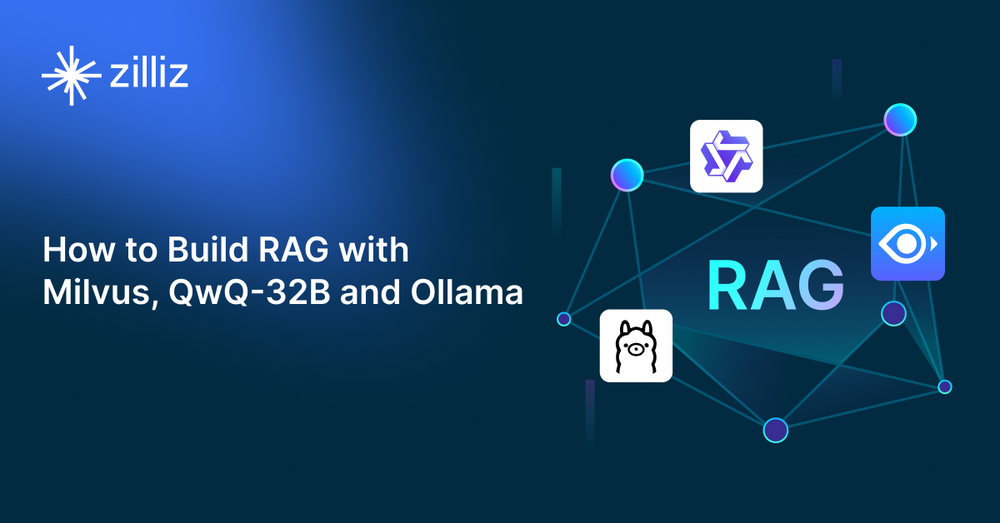
AI models are evolving fast, and Alibaba’s QwQ-32B is making a strong entrance recently. With just 32 billion parameters, this medium-sized reasoning model delivers impressive performance across mathematical reasoning, creative writing, and code generation—rivaling much larger models like DeepSeek-R1. Its efficiency and accuracy across benchmarks make it a compelling option for a wide range of AI applications.
11.jpeg
Figure 1: QwQ-32B’s performance in comparison to other leading models (Source)
Beyond its capabilities, QwQ-32B stands out for its accessibility. Unlike some massive models that require specialized hardware, it runs efficiently on consumer-grade GPUs like the RTX 4090, making it an excellent choice for developers and researchers looking for high-quality AI without enterprise-scale resources. However, as a dense model, QwQ-32B can sometimes struggle with long-text complex reasoning and may exhibit hallucinations, particularly when handling extended context windows.
To mitigate these challenges and enhance its reliability, we can integrate QwQ-32B with Retrieval-Augmented Generation (RAG). In this tutorial, we’ll walk through how to build a RAG system using QwQ-32B, Milvus (a high-performance vector database), and Ollama. By the end, you’ll have a streamlined, powerful AI pipeline that balances efficiency, accuracy, and scalability.
Before we go into details on how to build a RAG application, let's quickly go through all the technologies we'll use for this tutorial.
QwQ-32B vs. DeepSeek-R1
Both QwQ-32B and DeepSeek-R1 specialize in reasoning, but the latter adopts a Mixture-of-Experts (MoE) architecture, while QwQ-32B is a classic dense model.
- MoE models excel in knowledge-intensive scenarios (e.g., Q&A systems, information retrieval) and large-scale data processing, where different experts handle distinct data subsets to improve efficiency. However, their massive parameter count demands cloud or dedicated server resources.
- Dense models, though computationally intensive, are better suited for deep, coherent reasoning tasks (e.g., complex logical reasoning, in-depth reading comprehension) and algorithm design where real-time performance is not a must. Their compact size enables local deployment but may sometimes produce redundant and unnecessary messages.
Dense Model (QwQ-32B) | MoE Model (DeepSeek-R1) | |
---|---|---|
Advantages | Lower training complexity; straightforward process | High computational efficiency (activates partial experts during inference) |
Advantages | Coherent reasoning; full neuron engagement for contextual understanding | Scalable model capacity via expert expansion |
Disadvantages | High computational costs for training and inference | Complex training (requires gating networks and expert load balancing) |
Disadvantages | Limited scalability; prone to overfitting; high storage/deployment costs | Routing overhead (additional computation for gating decisions) |
Neither architecture is perfect. The choice should depend on task requirements, data characteristics, available computational resources, and budget constraints.
I believe we will be looking at hybrid approaches in the near future—using MoE for initial knowledge retrieval and coarse processing, and then dense models for deep reasoning and refinement, so as to achieve better performances.
Why Milvus?
Milvus is an open-source, high-performance, and highly scalable vector database that can store, index, and search billion-scale unstructured data through high-dimensional vector embeddings. It is perfect for building modern AI applications such as retrieval augmented generation (RAG), semantic search, multimodal search, and recommendation systems.
To mitigate QwQ-32B’s possible hallucinations (in fact, LLMs' possible ones), Milvus stores external or private knowledge and provides contextual information with the QwQ-32B model. This ensures that the QwQ-32B model can generate more accurate results.
Why Ollama?
Ollama is an open-source platform that simplifies the local deployment and management of large language models (LLMs). It provides a user-friendly, cloud-free experience, enabling effortless model downloads, installation, and interaction without requiring advanced technical skills. It allows users to quickly deploy models via simple command-line tools and Docker integration, and supports Modelfile management to streamline version control and model reuse.
Furthermore, Ollama offers a rich library of models—from general-purpose to domain-specific. It provides cross-platform and hardware compatibility—supporting macOS, Linux, Windows, and Docker container deployments—with automatic GPU detection and prioritization of acceleration. It also provides developer-friendly tools such as REST API and Python SDK, facilitating easy integration of models into various applications.
And it ensures data privacy and flexibility, empowering users to fine-tune, optimize, and deploy AI-driven solutions entirely on their machines.
Now, let's get started building a simple RAG pipeline with QwQ-32B as the language model, Milvus as the vector database, and Ollama as the framework.
Preparation
Dependencies and Environment
! pip install pymilvus ollama
Note: If you are using Google, to enable dependencies just installed, you may need to restart the runtime (click on the "Runtime" menu at the top of the screen, and select "Restart session" from the dropdown menu).
Prepare the data
We use the FAQ pages from the Milvus Documentation 2.4.x as the private knowledge in our RAG, which is a good data source for a simple RAG pipeline.
Download the zip file and extract documents to the folder milvus_docs
.
! wget https://github.com/milvus-io/milvus-docs/releases/download/v2.4.6-preview/milvus_docs_2.4.x_en.zip
! unzip -q milvus_docs_2.4.x_en.zip -d milvus_docs
We load all markdown files from the folder milvus_docs/en/faq
. For each document, we just simply use "# " to separate the content in the file, which can roughly separate the content of each main part of the markdown file.
from glob import glob
text_lines = []
for file_path in glob("milvus_docs/en/faq/*.md", recursive=True):
with open(file_path, "r") as file:
file_text = file.read()
text_lines += file_text.split("# ")
Prepare the LLM and Embedding Model
Ollama supports multiple models for both LLM-based tasks and embedding generation, making it easy to develop RAG applications. For this setup:
- We will use QwQ (32B) as our LLM for text generation tasks.
- For embedding generation, we will use mxbai-embed-large, a 334M-parameter model optimized for semantic similarity.
Before starting, ensure both models are pulled locally:
! ollama pull mxbai-embed-large
! ollama pull qwq
With these models ready, we can proceed to implement LLM-driven generation and embedding-based retrieval workflows.
import ollama
from ollama import Client
ollama_client = Client(host="http://localhost:11434")
def emb_text(text):
response = ollama_client.embeddings(model="mxbai-embed-large", prompt=text)
return response["embedding"]
Generate a test embedding and print its dimension and first few elements.
test_embedding = emb_text("This is a test")
embedding_dim = len(test_embedding)
print(embedding_dim)
print(test_embedding[:10])
1024
[0.23217937350273132, 0.42540550231933594, 0.19742339849472046, 0.4618139863014221, -0.46017369627952576, -0.14087969064712524, -0.18214142322540283, -0.07724273949861526, 0.40015509724617004, 0.8331164121627808]
Load data into Milvus
Create the Collection
from pymilvus import MilvusClient
milvus_client = MilvusClient(uri="./milvus_demo.db")
collection_name = "my_rag_collection"
As for the configuration of MilvusClient
parameters:
- Setting the
uri
as a local file, e.g../milvus.db
, is the most convenient method, as it automatically utilizes Milvus Lite to store all data in this file. - If you have a large scale of data, you can set up a more performant Milvus server on docker or kubernetes. In this setup, please use the server
uri
, e.g.http://localhost:19530
, as youruri
. - If you want to use Zilliz Cloud, the fully managed cloud service for Milvus, adjust the
uri
andtoken
, which correspond to the Public Endpoint and Api key in Zilliz Cloud.
Check if the collection already exists and drop it if it does.
if milvus_client.has_collection(collection_name):
milvus_client.drop_collection(collection_name)
Create a new collection with specified parameters.
If we don't specify any field information, Milvus will automatically create a default id
field for primary key, and a vector
field to store the vector data. A reserved JSON field is used to store non-schema-defined fields and their values.
milvus_client.create_collection(
collection_name=collection_name,
dimension=embedding_dim,
metric_type="IP", # Inner product distance
consistency_level="Strong", # Strong consistency level
)
Insert data
Iterate through the text lines, create embeddings, and then insert the data into Milvus.
Here is a new field text
, which is a non-defined field in the collection schema. It will be automatically added to the reserved JSON dynamic field, which can be treated as a normal field at a high level.
from tqdm import tqdm
data = []
for i, line in enumerate(tqdm(text_lines, desc="Creating embeddings")):
data.append({"id": i, "vector": emb_text(line), "text": line})
milvus_client.insert(collection_name=collection_name, data=data)
Creating embeddings: 100%|████████████████████████████████████████████████████████████████████████████████████████████████████████| 72/72 [00:06<00:00, 11.86it/s]
{'insert_count': 72, 'ids': [0, 1, 2, 3, 4, 5, 6, 7, 8, 9, 10, 11, 12, 13, 14, 15, 16, 17, 18, 19, 20, 21, 22, 23, 24, 25, 26, 27, 28, 29, 30, 31, 32, 33, 34, 35, 36, 37, 38, 39, 40, 41, 42, 43, 44, 45, 46, 47, 48, 49, 50, 51, 52, 53, 54, 55, 56, 57, 58, 59, 60, 61, 62, 63, 64, 65, 66, 67, 68, 69, 70, 71], 'cost': 0}
Build a RAG Pipeline
Retrieve data for a query
Let's specify a frequent question about Milvus.
question = "How is data stored in milvus?"
Search for the question in the collection and retrieve the semantic top-3 matches.
search_res = milvus_client.search(
collection_name=collection_name,
data=[
emb_text(question)
], # Use the `emb_text` function to convert the question to an embedding vector
limit=3, # Return top 3 results
search_params={"metric_type": "IP", "params": {}}, # Inner product distance
output_fields=["text"], # Return the text field
)
Let's take a look at the search results of the query.
import json
retrieved_lines_with_distances = [
(res["entity"]["text"], res["distance"]) for res in search_res[0]
]
print(json.dumps(retrieved_lines_with_distances, indent=4))
[
[
" Where does Milvus store data?\n\nMilvus deals with two types of data, inserted data and metadata. \n\nInserted data, including vector data, scalar data, and collection-specific schema, are stored in persistent storage as incremental log. Milvus supports multiple object storage backends, including [MinIO](https://min.io/), [AWS S3](https://aws.amazon.com/s3/?nc1=h_ls), [Google Cloud Storage](https://cloud.google.com/storage?hl=en#object-storage-for-companies-of-all-sizes) (GCS), [Azure Blob Storage](https://azure.microsoft.com/en-us/products/storage/blobs), [Alibaba Cloud OSS](https://www.alibabacloud.com/product/object-storage-service), and [Tencent Cloud Object Storage](https://www.tencentcloud.com/products/cos) (COS).\n\nMetadata are generated within Milvus. Each Milvus module has its own metadata that are stored in etcd.\n\n###",
231.9922637939453
],
[
"How does Milvus flush data?\n\nMilvus returns success when inserted data are loaded to the message queue. However, the data are not yet flushed to the disk. Then Milvus' data node writes the data in the message queue to persistent storage as incremental logs. If `flush()` is called, the data node is forced to write all data in the message queue to persistent storage immediately.\n\n###",
226.54090881347656
],
[
"What is the maximum dataset size Milvus can handle?\n\n \nTheoretically, the maximum dataset size Milvus can handle is determined by the hardware it is run on, specifically system memory and storage:\n\n- Milvus loads all specified collections and partitions into memory before running queries. Therefore, memory size determines the maximum amount of data Milvus can query.\n- When new entities and and collection-related schema (currently only MinIO is supported for data persistence) are added to Milvus, system storage determines the maximum allowable size of inserted data.\n\n###",
210.63682556152344
]
]
Use LLM to get a RAG response
Convert the retrieved documents into a string format.
context = "\n".join(
[line_with_distance[0] for line_with_distance in retrieved_lines_with_distances]
)
Define system and user prompts for LLM. This prompt is assembled with the retrieved documents from Milvus.
SYSTEM_PROMPT = """
Human: You are an AI assistant. You are able to find answers to the questions from the contextual passage snippets provided.
"""
USER_PROMPT = f"""
Use the following pieces of information enclosed in <context> tags to provide an answer to the question enclosed in <question> tags.
<context>
{context}
</context>
<question>
{question}
</question>
"""
Use the QwQ (32B)
model provided by Ollama to generate a response based on the prompts.
from ollama import chat
from ollama import ChatResponse
response: ChatResponse = ollama_client.chat(
model="qwq",
messages=[
{"role": "system", "content": SYSTEM_PROMPT},
{"role": "user", "content": USER_PROMPT},
],
)
print(response["message"]["content"])
<think>
Okay, I need to answer the question "How is data stored in Milvus?" using the provided context. Let me start by reading through the context carefully.
First, looking at the first context section titled "Where does Milvus store data?". It mentions that Milvus handles two types of data: inserted data and metadata.
Inserted data includes vectors, scalar data, and collection schema, stored as incremental logs in persistent storage. The supported backends are listed like MinIO, S3, GCS, etc. So I should note that inserted data goes to these object storages via incremental logs.
Then metadata is stored in etcd for each Milvus module. That's straightforward—metadata in etcd.
The second context about flushing data explains that data first goes to the message queue and then to persistent storage when flushed. But this might be more about the process rather than where it's stored, so maybe mention that insertion is into the message queue first before being written out.
Third section on maximum dataset size mentions hardware constraints: memory for queryable data and storage for persistence. This could relate to how data is managed in terms of memory vs disk. The user asked "how is data stored," which might include both where (like object stores, etcd) and the process (message queue then persistent logs).
Putting it all together, the answer should mention:
- Two types: inserted data and metadata.
For inserted data:
- Stored as incremental logs in persistent storage using backends like MinIO, S3 etc.
- Initially loaded into message queues, then written to disk. The flush() forces immediate writing if needed.
Metadata:
- Stored in etcd for each module.
Also, the hardware part says that memory limits queryable data size and storage (like the object stores) limit total dataset size. So maybe mention these factors as part of how data is stored, considering capacity constraints.
I should structure this clearly, perhaps bullet points or sections on inserted vs metadata, then processes like flushing, and finally limitations based on hardware.
</think>
Data in Milvus is stored in two main ways:
1. **Inserted Data**:
- Includes vector data, scalar data, and collection schema.
- Stored as **incremental logs** in **persistent storage**, supported by object storage backends such as MinIO, AWS S3, Google Cloud Storage (GCS), Azure Blob Storage, Alibaba Cloud OSS, and Tencent Cloud Object Storage (COS).
- Initially loaded into a **message queue** when inserted. Milvus returns success upon insertion into the queue but does not immediately write to disk. A background process later writes this data to persistent storage. The `flush()` method can force immediate writing of all queued data to storage.
2. **Metadata**:
- Generated internally by Milvus modules (e.g., collection configurations, partitions).
- Stored in **etcd**, a distributed key-value store.
**Hardware Considerations**:
- **Memory**: The amount of data Milvus can query is limited by system memory since it loads specified collections/partitions into memory for queries.
- **Storage Capacity**: The maximum dataset size is constrained by the underlying storage backend (e.g., object storage), which stores all inserted data and schema incrementally.
Great! We have successfully built a RAG pipeline with Milvus, QWQ-32B and Ollama.
Conclusion
By integrating these technologies, we can build a RAG system that leverages Milvus for efficient data storage and retrieval and QwQ-32B's reasoning capabilities to generate accurate and contextually relevant responses. Ollama streamlines the deployment process, allowing for a seamless and efficient setup. This combination is particularly beneficial for applications requiring real-time information retrieval and generation, such as AI-assisted tutoring, logic-based problem-solving, and more.
We hope by following this tutorial, you can create RAG systems tailored to your needs and be truly beneficial from your own creations.
Start Free, Scale Easily
Try the fully-managed vector database built for your GenAI applications.
Try Zilliz Cloud for FreeKeep Reading
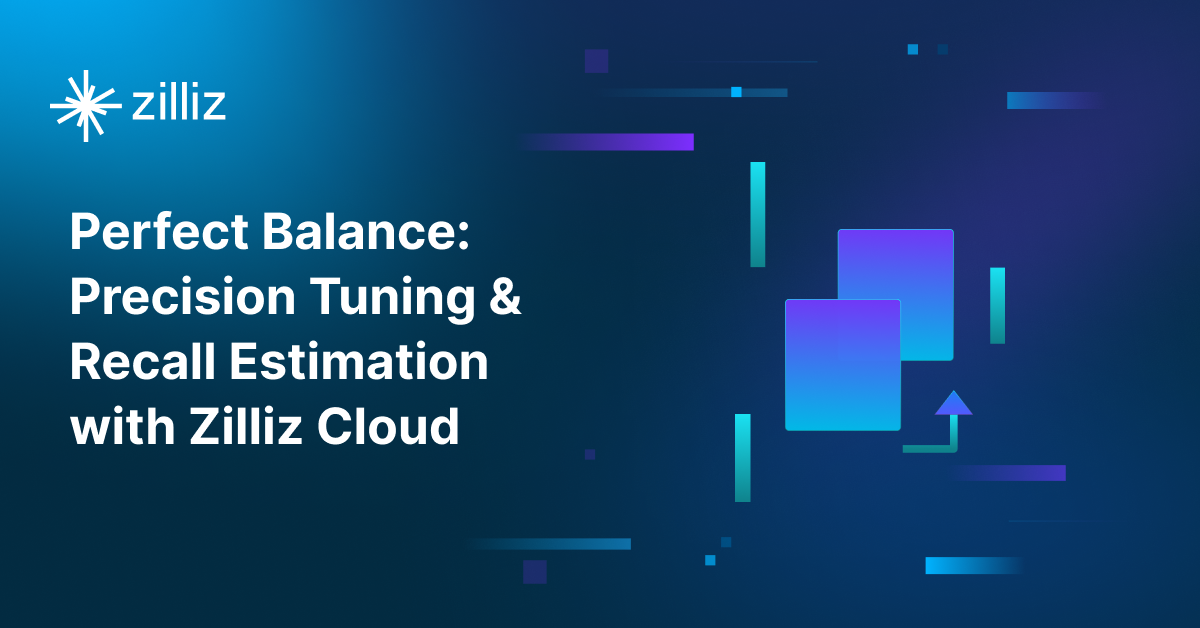
Balancing Precision and Performance: How Zilliz Cloud's New Parameters Help You Optimize Vector Search
Optimize vector search with Zilliz Cloud’s level and recall features to tune accuracy, balance performance, and power AI applications.
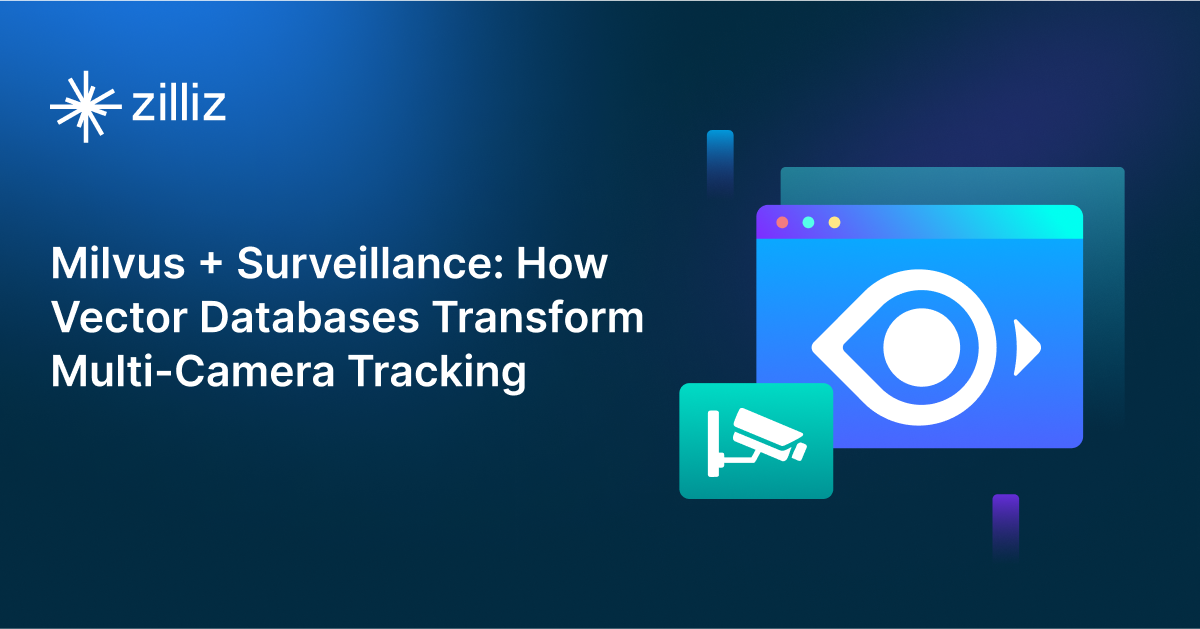
Milvus/Zilliz + Surveillance: How Vector Databases Transform Multi-Camera Tracking
See how Milvus vector database enhances multi-camera tracking with similarity-based matching for better surveillance in retail, warehouses and transport hubs.
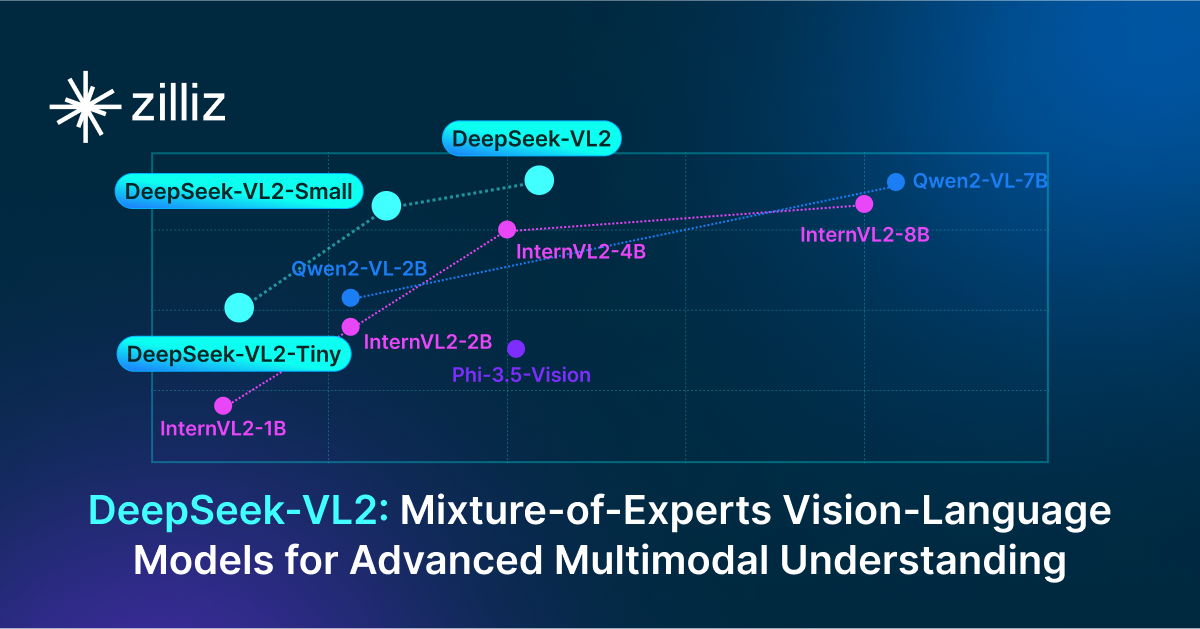
DeepSeek-VL2: Mixture-of-Experts Vision-Language Models for Advanced Multimodal Understanding
Explore DeepSeek-VL2, the open-source MoE vision-language model. Discover its architecture, efficient training pipeline, and top-tier performance.