Boosting Work Efficiency with Generative AI Use Cases
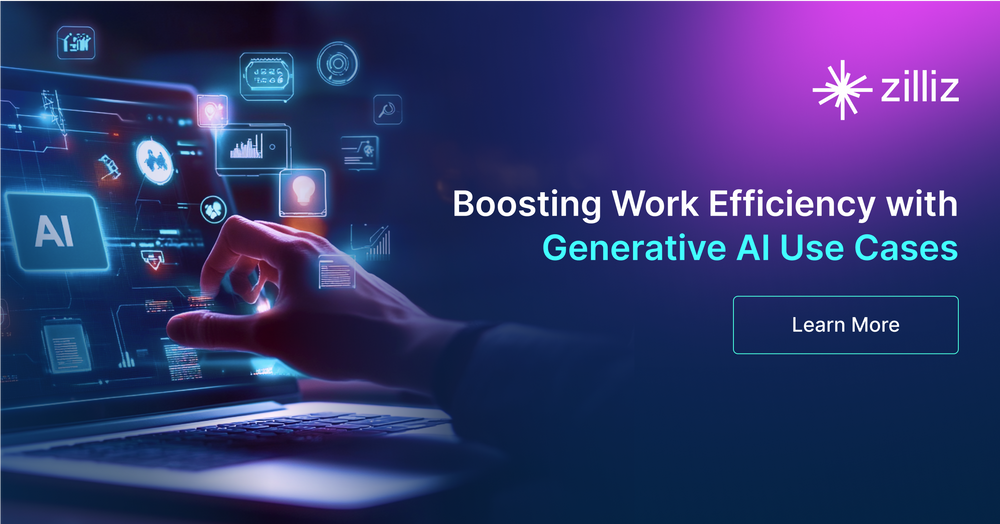
In today’s rapidly evolving business world, we must embrace cutting-edge technologies like Generative AI (GenAI) to stay competitive. From automating mundane tasks to enhancing decision-making, GenAI is transforming how industries operate. But what does this mean for your organization?
This blog will explore how Generative AI (GenAI) applications can boost work efficiency. We draw insights from a recent talk at the Unstructured Data Meetup by Lucy Park, Chief Scientific Officer at Upstage AI. If you want a deeper dive, we recommend you watch Lucy’s talk on YouTube.
Lucy Park from Upstage speaking at the May Unstructured Data Meetup at SAP Palo Alto
The Power of GenAI in Transforming Business Operations
GenAI is rapidly becoming a key driver of innovation across industries, enhancing work efficiency and enabling smarter decision-making. By automating repetitive tasks, boosting productivity, and offering deeper insights, GenAI is fundamentally reshaping business operations. Its applications are vast, from customer service automation to supply chain optimization, significantly impacting various sectors.
To fully grasp how GenAI systems achieve these efficiencies, it’s essential to understand the underlying technologies. Central to GenAI’s success are advanced tools like Large Language Models (LLMs) and multimodal models for content generation, vector databases like Milvus and Zilliz Cloud for semantic similarity search, and embedding models that transform unstructured data into vector embeddings. Retrieval Augmented Generation (RAG) further enhances generated content quality by providing contextual information with GenAI models.
A practical example of GenAI in action is how platforms like Upstage AI utilize these technologies to automate workflows and solve industry-specific challenges. For instance, Document AI leverages generative models to extract and interpret information from unstructured documents, reducing manual effort and increasing efficiency in sectors like insurance and finance. Another example is Solar LLM, a specialized language model tailored to industries like finance and healthcare. Solar LLM ensures that GenAI applications deliver precise and relevant results by focusing on industry-specific language and needs.
Upstage AI also proposed a full-stack GenAI system, which integrates structured and unstructured data to generate accurate, contextually relevant responses. This advanced architecture goes beyond traditional RAG systems that rely solely on vector databases for retrieving unstructured data through semantic similarity search. By incorporating additional components like relational databases, Upstage AI’s system processes and retrieves structured data, resulting in more precise and comprehensive outcomes.
The architecture of Upstage AI’s full-stack LLM system
The diagram above illustrates the functionality of this full-stack GenAI system:
User Query Processing: The system starts by processing the user’s query, which may include structured and unstructured data. Structured data is converted into SQL commands using the NL2SQL model, while unstructured data, such as natural language, is transformed into vector embeddings. These SQL queries and vectors are then sent to relational and vector databases to search for relevant information.
Document Processing: Domain-specific knowledge also involves structured and unstructured data. Structured data, such as business logs or website information, is stored in relational or NoSQL databases. For unstructured documents like PDFs, emails, or Slack messages, a Key Information Extractor identifies crucial information, and a Layout Analyzer, often paired with a chunking process, breaks down complex documents into manageable parts. These chunks are then converted into vector embeddings using specialized embedding models and stored in a vector database like Milvus for quick and accurate semantic similarity search.
Retriever: The system retrieves relevant structured and unstructured data from their databases.
Generative Model and Groundedness Checker: The retrieved data is fed into a generative model to construct a response. A Groundedness Checker (GC) then verifies the accuracy and relevance of the generated content.
Final Response: The system delivers a contextually accurate and relevant response to the user.
GenAI Use Cases for Boosting Work Efficiency
The transformative potential of GenAI is evident across various applications. Below are some key areas where GenAI significantly boosts work efficiency:
Process Automation
Manual data entry and document processing are time-consuming and prone to human error. Tasks like entering invoice details into financial systems, processing client onboarding forms, and updating employee records are still done manually in many organizations. GenAI tools like Document AI automate extracting and classifying information from these documents, reducing errors and freeing up time for strategic tasks like financial analysis, client relationship management, and employee development.
Customer Support Automation
GenAI has made significant strides in customer support. AI-driven chatbots and virtual assistants handle common customer queries, reducing the workload on human agents and ensuring 24/7 support. For instance, Zendesk uses AI-powered bots to automate responses to frequently asked questions, allowing customer service representatives to focus on more complex issues. Similarly, Bank of America’s virtual assistant, Erica, uses GenAI to help customers manage their accounts, answer queries about transactions, and provide financial advice, significantly enhancing the overall customer experience.
Content Creation and Personalization
GenAI plays a pivotal role in content creation and personalization by automating the production of high-quality content and tailoring it to individual preferences. For example, in marketing, tools like Copy.ai quickly generate compelling ad copy, social media posts, and email content, allowing marketers to produce engaging material at scale. Platforms like Netflix utilize GenAI to analyze user viewing habits and recommend movies and shows that match individual tastes, ensuring personalized content delivery.
Supply Chain Optimization
GenAI optimizes supply chain operations in manufacturing and logistics, ensuring products are delivered on time and at the lowest possible cost. For example, IBM's Watson Supply Chain uses GenAI to predict disruptions and recommend corrective actions. By analyzing data from various sources, Watson anticipates issues like delays or shortages and suggests alternative routes or suppliers, helping companies maintain smooth operations.
Automated News Generation
News organizations increasingly use GenAI to automate the writing of news articles, especially for real-time reporting. Platforms like The Associated Press (AP) use AI to generate articles on sports events, financial reports, and weather updates. The AI system processes structured data like game statistics or stock prices. It produces well-formed news articles almost instantly, allowing news agencies to cover more events quickly and efficiently.
Conclusion
Generative AI is redefining how businesses operate across various sectors. By automating complex tasks like content creation and personalizing user experiences at scale, GenAI empowers organizations to achieve higher efficiency and more meaningful engagement with their audiences. These intelligent systems are shaping the future of work, making GenAI an essential asset for any forward-thinking organization. For businesses looking to enhance work efficiency through advanced data storage, indexing, and retrieval processes, integrating tools like Zilliz Cloud, which offers robust vector search services across major platforms, is critical to achieving these goals.
Further Reading
Learn the following resources if you want a deeper dive into LLM evaluations.
- The Power of GenAI in Transforming Business Operations
- GenAI Use Cases for Boosting Work Efficiency
- Conclusion
- Further Reading
Content
Start Free, Scale Easily
Try the fully-managed vector database built for your GenAI applications.
Try Zilliz Cloud for FreeKeep Reading
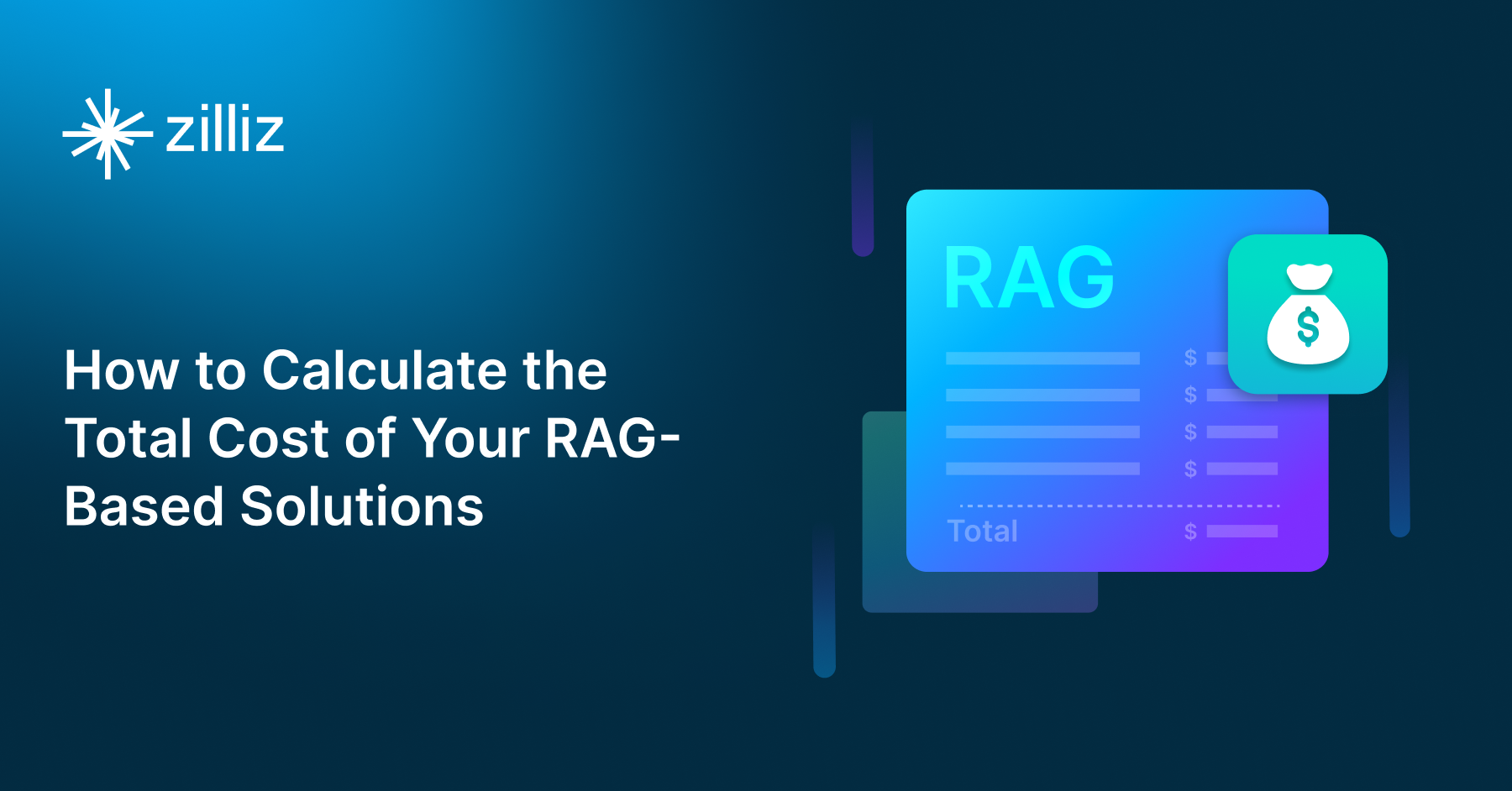
How to Calculate the Total Cost of Your RAG-Based Solutions
In this guide, we’ll break down the main components of RAG costs, show you how to calculate these expenses using the Zilliz RAG Cost Calculator, and explore strategies to manage spending efficiently.
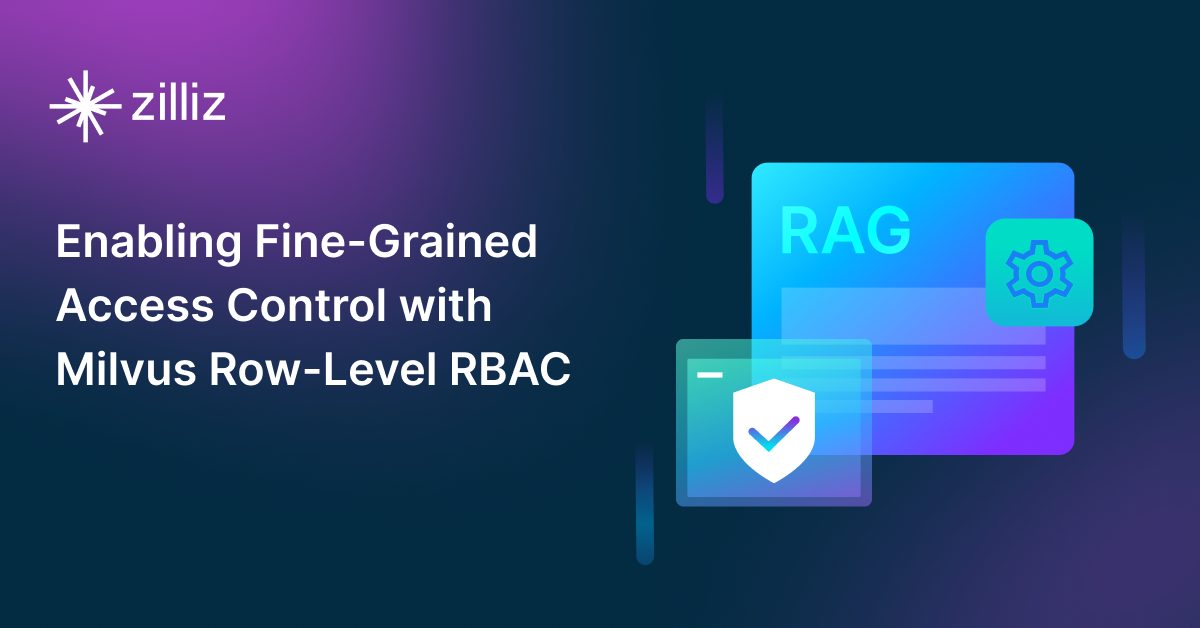
Enabling Fine-Grained Access Control with Milvus Row-Level RBAC
Milvus offers row-level RBAC (Role-Based Access Control) which is a robust solution for managing data access with precision and efficiency.
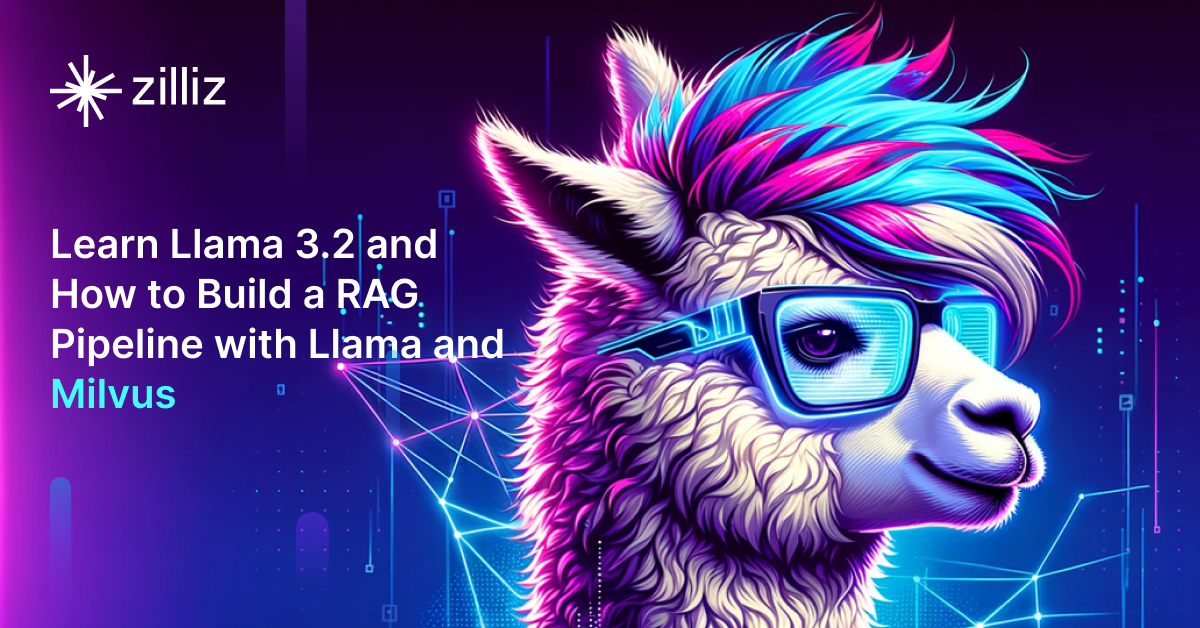
Learn Llama 3.2 and How to Build a RAG Pipeline with Llama and Milvus
introduce Llama 3.1 and 3.2 and explore how to build a RAG app with Llama 3.2 and Milvus.