Evaluating Safety & Alignment of LLM in Specific Domains
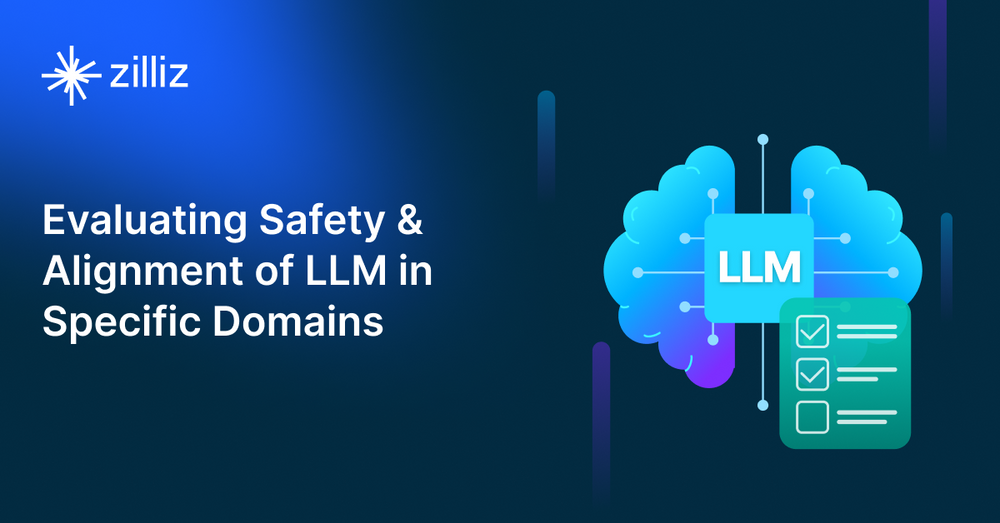
Recent advancements in AI have given rise to sophisticated Large Language Models (LLMs) with potentially transformative impacts across high-stakes domains such as healthcare, financial services, and legal industries. Although these models provide significant advantages, their use in critical decision-making requires thorough evaluation to guarantee safety, accuracy, and ethical standards. Serious concerns surrounding these models' accuracy, security, and fairness must be addressed before fully embracing AI in such sensitive environments.
At a recent Unstructured Data Meetup, Zhuo Li, CEO at of Hydrox AI, spoke about the current landscape of safety evaluations for LLMs and shared insights into an ongoing evaluation framework project in collaboration with AI Alliance. This project aims to develop comprehensive tools and methods to ensure LLMs' safe and responsible deployment in sensitive environments.
Zhuo Li speaking at Sep Unstructured Data Meetup with the AI Alliance
In this blog, we’ll go into the key points discussed during the event and explore how companies like Hydrox AI and AI Alliance are tackling the critical challenges of AI safety and evaluation.
Why Safety Evaluation is Key?
Zhuo Li emphasized the importance of safety evaluations for industries dealing with sensitive information. While many LLMs can handle general safety issues, they often fall short in real-world scenarios faced by healthcare, banking, or education professionals. An LLM may perform well in general tasks but struggle with sensitive data like medical records or financial statements.
Safety evaluations for LLMs must consider factors such as accuracy, legal regulations, and ethical responsibilities. These evaluations should be ongoing, as new challenges and vulnerabilities arise continuously. Regular testing and improvements are essential to adapt to the changing landscape.
Furthermore, the implications of inaccurate or biased AI outputs can be critical in high-stakes environments. For instance, in healthcare, a wrong diagnosis suggested by an LLM could lead to inappropriate treatments, endangering patient lives. Similarly, in finance, inaccurate risk assessments generated by AI could lead to significant financial losses for companies and individuals. Therefore, developing robust evaluation methodologies becomes imperative to safeguard stakeholders' interests.
Current Challenges in AI Evaluation
One of the biggest issues in evaluating AI models is unreliable benchmarks. While several evaluation tools are available, many aren't updated often enough or don't cover the specific needs of industries dealing with sensitive data. As a result, companies might make decisions based on outdated or incomplete information when choosing which AI model to use.
Besides lacking up-to-date benchmarks, there is also a shortage of tools to help people fully understand the risks involved and take the right steps to address them. This gap is what companies like Hydrox, in partnership with IBM, are aiming to fill within the AI Alliance. They are working on a comprehensive evaluation framework for LLMs, currently with an initial version, that is tailored to high-stakes domains. The goal is to ensure these models meet strict standards for safety, legal compliance, and ethical use, which will help minimize errors and risks.
The key objectives of this framework include:
Evaluating LLMs in critical sectors like healthcare, finance, and legal services.
Developing an evaluation framework backed by domain experts and real-world applications.
Proof of Concept (PoC) to assess the safety, scalability, and risks of LLMs.
Industry-first recommendations on integrating generative AI while considering safety, technical requirements, and regulations.
Focusing on existing pre-trained models and specific tasks identified by experts.
The initial version of this framework is called EPASS (Evaluation Platform for AI Safety and Security), and already covers several attack methods, such as "artprompt" and "gptfuzzer," and analyzes content categories like sexual content and misinformation.
Real-World Case Studies: Healthcare and Education
Zhuo Li shared some case studies showing how this evaluation framework can be used in sensitive areas like healthcare and education.
LLMs can assist doctors in healthcare by summarizing patient notes or analyzing medical images, significantly improving efficiency. However, given the sensitivity of healthcare data, AI systems must ensure compliance with laws like HIPAA. The framework helps ensure doctors receive clear outputs by checking AI models for biases in diagnosis or treatment suggestions.
For example, using LLMs to suggest treatment options based on historical patient data can speed up clinical decision-making. However, it's crucial that these suggestions are based on accurate and unbiased data to avoid potential harm to patients. This is where safety evaluations become essential—not just in ensuring compliance but also in maintaining trust among patients, who must feel confident that their data is handled securely and that AI-driven recommendations are reliable.
In education, AI can be used for teaching, grading, and assessment decisions. However, it is vital to protect student data, comply with privacy regulations like FERPA, and ensure the accuracy of AI recommendations. Regular audits of these models can help identify and eliminate biases, fostering trust in AI systems among students and teachers.
AI can also assist in personalized learning, adapting educational content to fit individual student needs. Yet, the models must be regularly evaluated to prevent reinforcing existing biases or inaccuracies in grading, which could affect students' performance. A well-structured evaluation framework can enhance the effectiveness of personalized learning by ensuring that the algorithms adapt appropriately to students' learning styles and backgrounds.
EPASS (Evaluation Platform for AI Safety and Security)
At the end of his talk, Zhuo Li introduced EPASS, a platform developed over the past ten months. Currently, the platform supports general use cases and various attack methods, with regular updates and the addition of more domain-specific use cases.
EPASS VIDEO
Figure 1 shows the current models dashboard, which displays the ranking of each model across four areas:
Safety: Risks that promote danger such as crime and hate speech.
Privacy: Risks related to data breaching such as data sharing and membership interference.
Security: Behavioral risks of the model such as roleplay and prompt injection.
Integrity: Risks related to model ethics such as copyright and fraud.
Figure 1: Models Dashboard (100 = most safe, 0 = least safe)
These models are regularly evaluated and updated, and a comparison of all models is illustrated in Figures 2 and 3. These figures show the overall score based on the areas described above and the scores based on the different attack methods.
Figure 2: Models Leaderboard (Overall Evaluation)
Among the current 19 attack methods available, the following can be highlighted:
Analyzing-based Jailbreak (ABJ): This method takes advantage of LLMs’ ability to analyze and reason, revealing their weaknesses when faced with analytical tasks.
ArtPrompt: This exploits LLMs' difficulty in understanding ASCII art.
DrAttack: This method breaks down prompts and rebuilds them using synonym searches and in-context learning.
Developer: This induces jailbreaks by using prompts that mimic developer mode
Users can evaluate models on the platform and get a score on the above areas and attack methods for their specific use case. These allow regular updates and improvements on the models and provide insights on strengths and weaknesses of each one.
Figure 3: Models Leaderboard (Attacks Methods)
Additionally, for each model, users can explore more in-depth the up-to-date overall scores (Figure 4) and scores in the specific areas, such as safety (Figure 5), privacy (Figure 6), security (Figure 7), and integrity (Figure 8). For example, the safety score is evaluated based on issues like misinformation, ethics, crime, and violence. In the privacy area, factors such as data collection, deletion, and sharing are crucial. For security, the focus is on prompt injection and API vulnerabilities. Finally, in terms of integrity, concerns like spam, fraud, and copyright violations are included.
Figure 4: Overall Score
Figure 5: Safety Score
Figure 6: Privacy Score
Figure 7: Security Score
Figure 8: Integrity Score
It is also possible to use the platform playground to validate prompts based on the above criteria. If an attack is detected by the model, the specific issue will be highlighted with a short description as seen in Figure 9.
This detailed breakdown of evaluations provided in the EPASS platform not only informs users about the potential vulnerabilities of LLMs but also empowers them to take proactive measures in securing their applications. By understanding the various ways models can fail, or can be manipulated, stakeholders can make informed decisions about implementing safeguards and monitoring mechanisms.
Moreover, ongoing collaborations, such as those facilitated by AI Alliance, along with awareness initiatives for users and developers, are crucial in the evolving landscape of AI deployment. As LLMs become increasingly integrated into daily operations, it is essential for teams to grasp both the capabilities and limitations of these models. Collaborative platforms like EPASS, which address AI ethics, safety, and technical limitations, can foster a culture of responsible AI use. This collective approach not only helps prevent potential pitfalls but also enhances overall safety, ensuring that AI technologies are applied effectively and ethically in high-stakes environments.
Figure 9: Integrity Score
Conclusion
As AI continues to evolve, the demand for safety evaluations will only increase. Companies like Hydrox AI and AI Alliance are leading the effort to ensure LLMs can operate in high-stakes domains without compromising accuracy, security, or ethics. This is not just about functionality but about ensuring safe and responsible use.
Zhuo Li’s presentation highlighted the need for ongoing collaboration between AI developers, domain experts, and companies. The future of AI in sectors like healthcare, finance, and education relies on our ability to continuously evaluate and enhance these models.
While AI holds the potential to revolutionize high-stakes domains, its implementation must be thoughtful and careful. By creating comprehensive evaluation frameworks and tools, Hydrox and its partners are establishing a foundation for a safer, more responsible AI-driven future.
Moreover, as these technologies continue to develop, it is crucial for stakeholders—including policymakers, industry leaders, and researchers—to engage in discussions about the ethical implications of AI. Establishing best practices, guidelines, and regulatory frameworks will help mitigate risks and promote transparency in AI deployment. As we navigate this rapidly changing landscape, fostering a culture of responsibility and accountability in AI development will be key to ensuring that the benefits of LLMs are realized while minimizing potential harms.
- Conclusion
Content
Start Free, Scale Easily
Try the fully-managed vector database built for your GenAI applications.
Try Zilliz Cloud for FreeKeep Reading
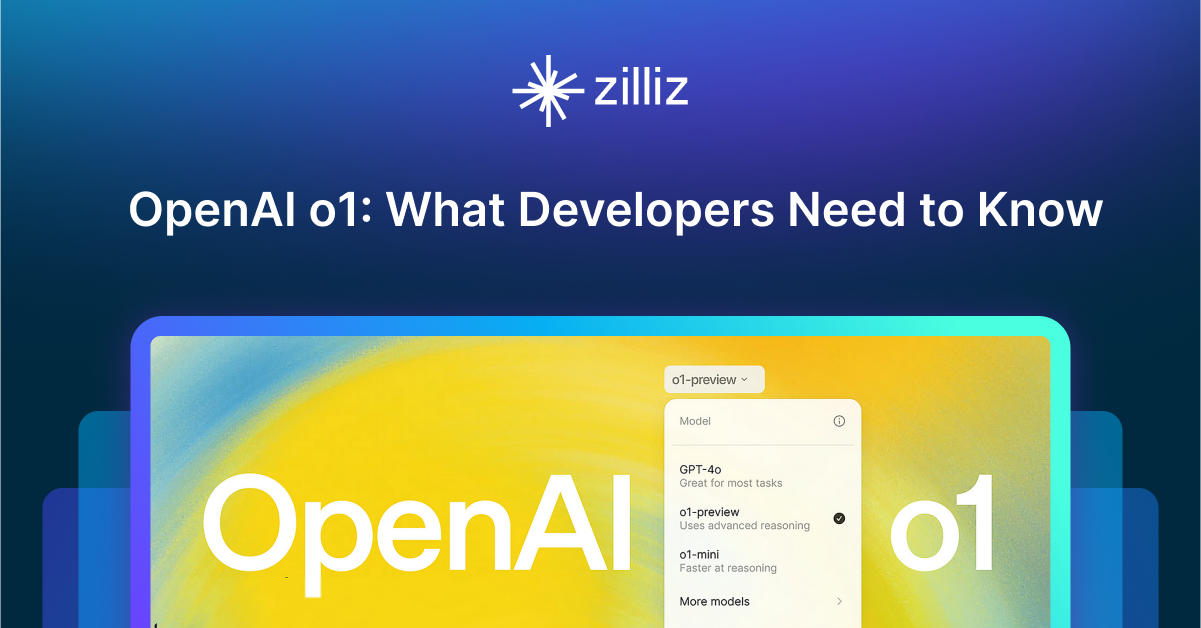
OpenAI o1: What Developers Need to Know
In this article, we will talk about the o1 series from a developer's perspective, exploring how these models can be implemented for sophisticated use cases.
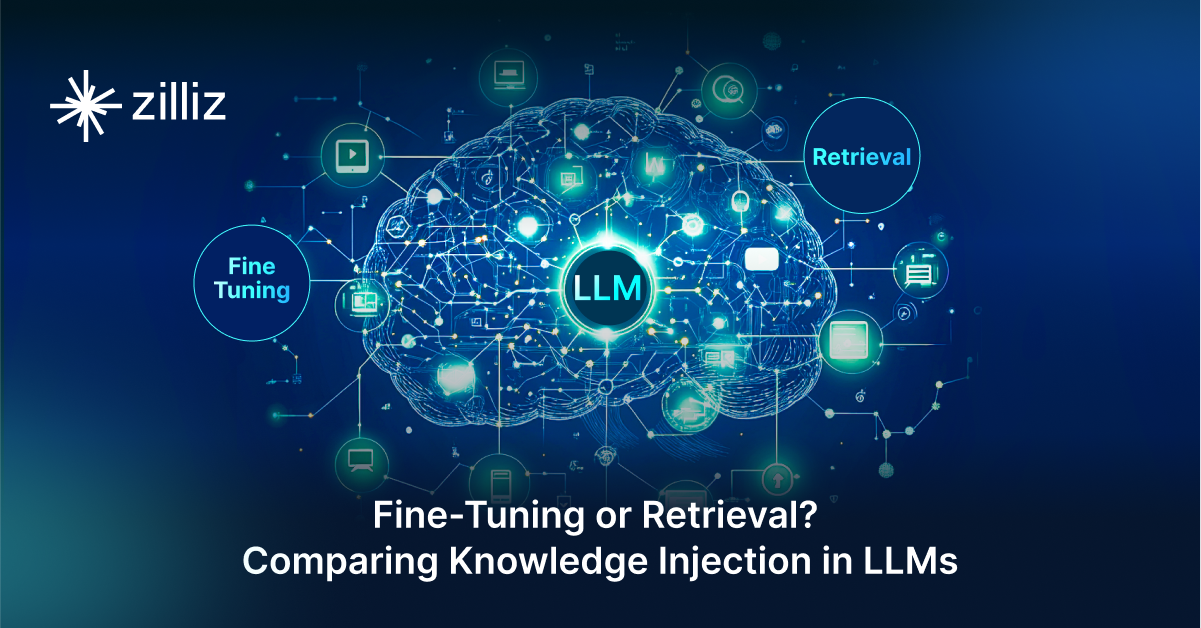
Knowledge Injection in LLMs: Fine-Tuning and RAG
Explore knowledge injection techniques like fine-tuning and RAG. Compare their effectiveness in improving accuracy, knowledge retention, and task performance.
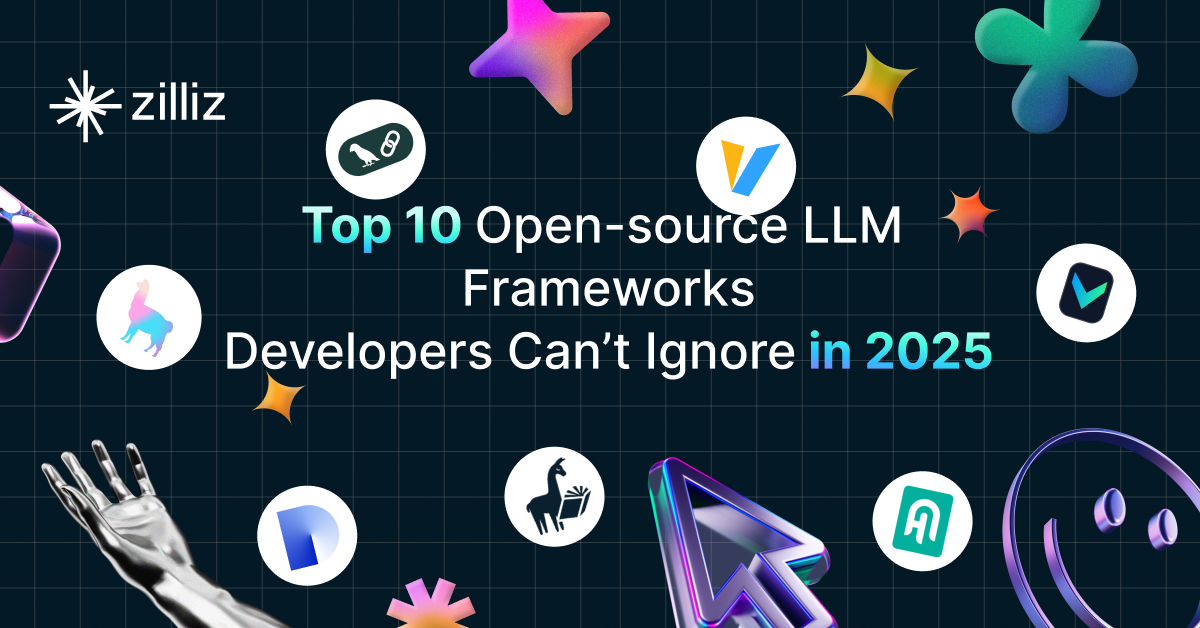
10 Open-Source LLM Frameworks Developers Can’t Ignore in 2025
LLM frameworks simplify workflows, enhance performance, and integrate seamlessly with existing systems, helping developers unlock the full potential of LLMs with less effort.