Building an AI-driven Car Repair Assistant with Milvus and the OpenAI LLM
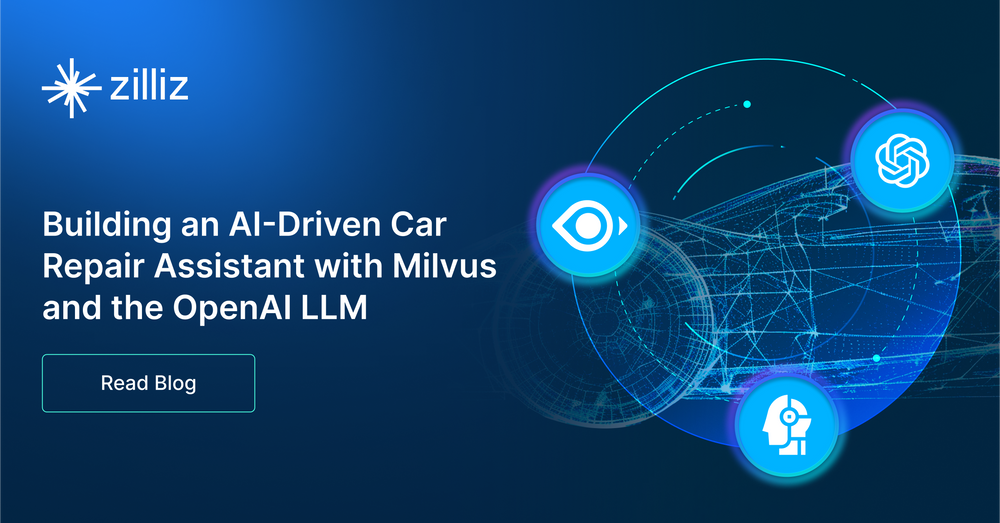
Why We Built an AI-drive Car Repair Assistant
Have you ever had a familiar sinking feeling when an unfamiliar light suddenly appears on your dashboard or an unexpected noise comes from under the hood of your car? It's a mix of apprehension, confusion, and the dread of impending inconvenience or costly repairs.
This moment, fraught with uncertainty, is where we all long for reassurance—a knowledgeable friend or family member we can instantly turn to for advice. However, not all of us are lucky to have such a person in our lives. Back in the day, a beloved "Car Talk" radio show gave a glimmer of hope that everyone could have that pair of Boston uncles who were just a phone call away, ready to tell us what to do next.
Our AI-driven car repair assistant project seeks to bring this very comfort to every car owner.
In the spirit of collaboration, dedicated coding, and problem-solving to real-world problems, we (Brandon Hoffman, Lin Liu, Mark Grube, and Danni Li) decided to explore new tools to improve our automobile assistant product during the Open Source AI Hackathon in Seattle on Jan 27, 2024. Our AI-driven car repair assistant project aims to create an interactive and reliable platform for drivers seeking automotive advice and solutions.
How We Built the Car Repair Assistant with Milvus and the OpenAI Model
During the hackathon, we used the OpenAI LLM model, with the Milvus vector database storing and retrieving car-issue-related context. Our product combines user inputs (car make, model, year, and issues) with AI capabilities to provide relevant diagnostic suggestions.
Unlike the traditional ways of seeking automotive guidance (via mechanics, car manuals, online forums, etc.), our automotive assistant product will provide accessible and enjoyable experiences for drivers of all skill levels when they need to consult on their car issues. We also see the possibility of pinpointing diagnosis and repair suggestions for professional auto mechanics. In its most advanced form, the tool could seamlessly integrate with a vehicle's systems, offering real-time feedback, maintenance updates, and tailored advice directly to the driver.
We hope this project represents more than just a nod to a bygone era of radio-based automotive camaraderie; it is a forward-looking endeavor to revolutionize car maintenance and repair in today's digital world. With Generative AI at our helm, we aim to both refine the process of identifying car issues and broaden access to expert advice, bridging the growing divide between drivers and their increasingly intricate vehicles. Check out the project on the GitHub page.