Optimizing User Experience: BIGO Leverages Milvus for Duplicate Video Removal
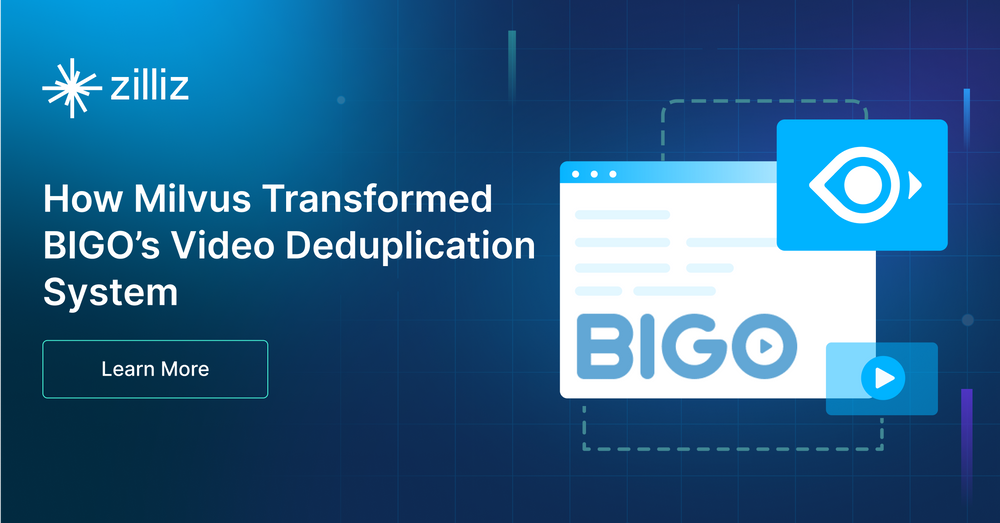
Short video-sharing platforms have become an integral part of our daily lives. Likee, a global short video platform owned by BIGO, has millions of short video uploads daily. However, with the sheer number of new videos daily, the problem of duplicate videos poses a threat to the content quality and the overall user experience. To tackle this issue, BIGO used Milvus, an open-source vector database, to transform its video deduplication system.
In this post, we will discuss the specific challenges BIGO faced, why the company chose the Milvus vector database to power its video deduplication system, and how Milvus came to the rescue.
The surge of duplicate videos leads to poor user experience
With an impressive user base exceeding 400 million, Likee witnesses millions of new video uploads daily. However, the proliferation of new content comes with its own set of challenges, particularly in the form of duplicate videos. This surge threatens to maintain high-quality content recommendations and user-friendly experiences and raises concerns about potential violations of the intellectual property rights of other creators.
In the past, Likee addressed this issue by employing FAISS, a similarity search and clustering library. While effective initially, FAISS revealed limitations when confronted with the monumental task of managing and storing massive vectors. This limitation led to sluggish query responses and constrained throughput. Therefore, the Likee team embarked on a quest for a more efficient technology capable of swiftly identifying and eliminating the burgeoning number of duplicate videos.
Milvus: a catalyst for change
Likee turned to Milvus, an open-source vector database designed to store, index, and query billion-scale embedding vectors in the quest for a more efficient solution. The impact was nothing short of revolutionary. Milvus injected lightning-fast similarity search capabilities into Likee's deduplication system, completing searches of duplicate videos in under 200 milliseconds while maintaining a high recall rate. Likee also benefited from Milvus's scalability, resulting in improved throughput of vector queries and increased working efficiency.
Tackling Likee’s duplicate videos with Milvus
The transformation of Likee's deduplication system is fascinating. Newly uploaded videos undergo a meticulous transformation: they are sliced into frames, converted into feature vectors, and then intricately matched against an extensive database housing over 700 million vectors corresponding to pre-existing content. This intricate process is a choreography of cutting-edge technologies involving storing videos in Kafka, converting videos into vector embeddings through deep learning models, indexing embeddings with Milvus, and storing recalled results in Ceph. For better video matching, video IDs corresponding to vector embeddings are managed in TiDB or Pika, two relational databases.
The architecture of Likee's deduplication system
Empowering Likee's quest for similarity search with Milvus
Milvus brings a new level of efficiency to Likee's similarity search process. Milvus recalls the top 100 vectors similar to each feature vector from a new video by conducting a batch search. The system then identifies and removes duplicate videos by comparing video IDs, retrieving the feature vectors of the remaining videos, and scoring the similarity between the retrieved and query video feature vectors.
How Milvus helps Likee’s similarity search
Towards a collaborative horizon
Milvus's success in refining Likee's video deduplication system sets the stage for broader collaborations between BIGO and Milvus. Xinyang Guo, Software Engineer at BIGO, envisions extending Milvus's prowess to content moderation, restriction, and customized video services. The synergy between BIGO and Milvus promises a mutually beneficial journey, with both entities poised for sustained growth and prosperity.
In conclusion, Milvus emerges as the driving force propelling BIGO's Likee into a new era of efficiency and user satisfaction. As the partnership evolves, the success story of Milvus in solving intricate challenges exemplifies the potential of open-source technologies to navigate and conquer the complexities of the digital landscape.
- The surge of duplicate videos leads to poor user experience
- Milvus: a catalyst for change
- Towards a collaborative horizon
Content
Start Free, Scale Easily
Try the fully-managed vector database built for your GenAI applications.
Try Zilliz Cloud for FreeKeep Reading
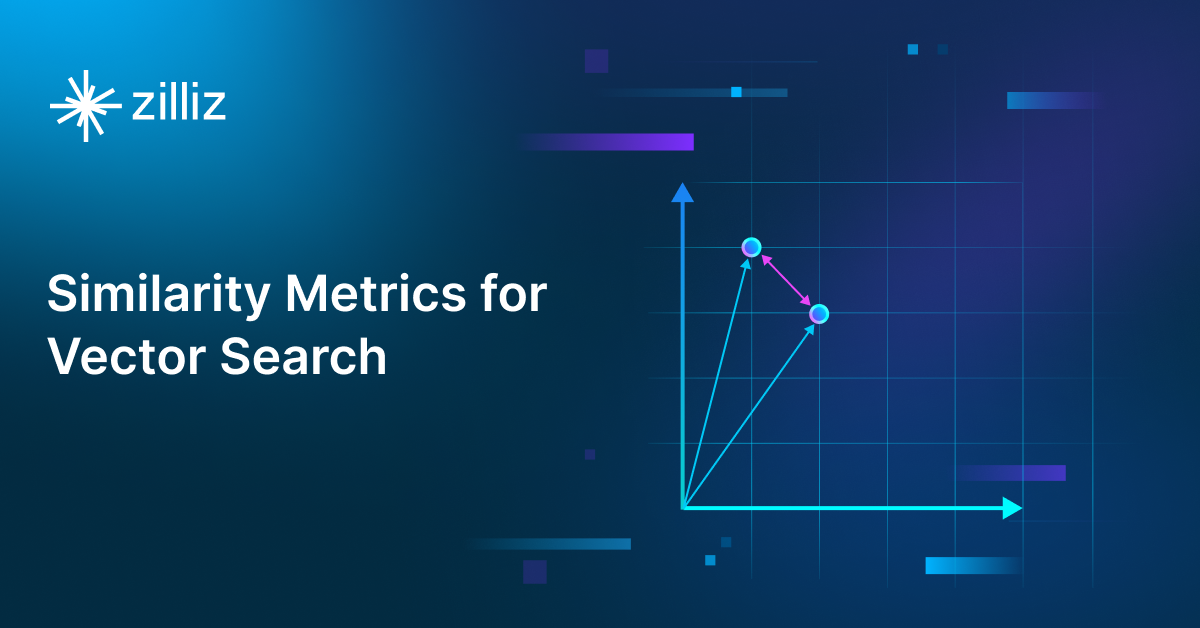
Similarity Metrics for Vector Search
Exploring five similarity metrics for vector search: L2 or Euclidean distance, cosine distance, inner product, and hamming distance.
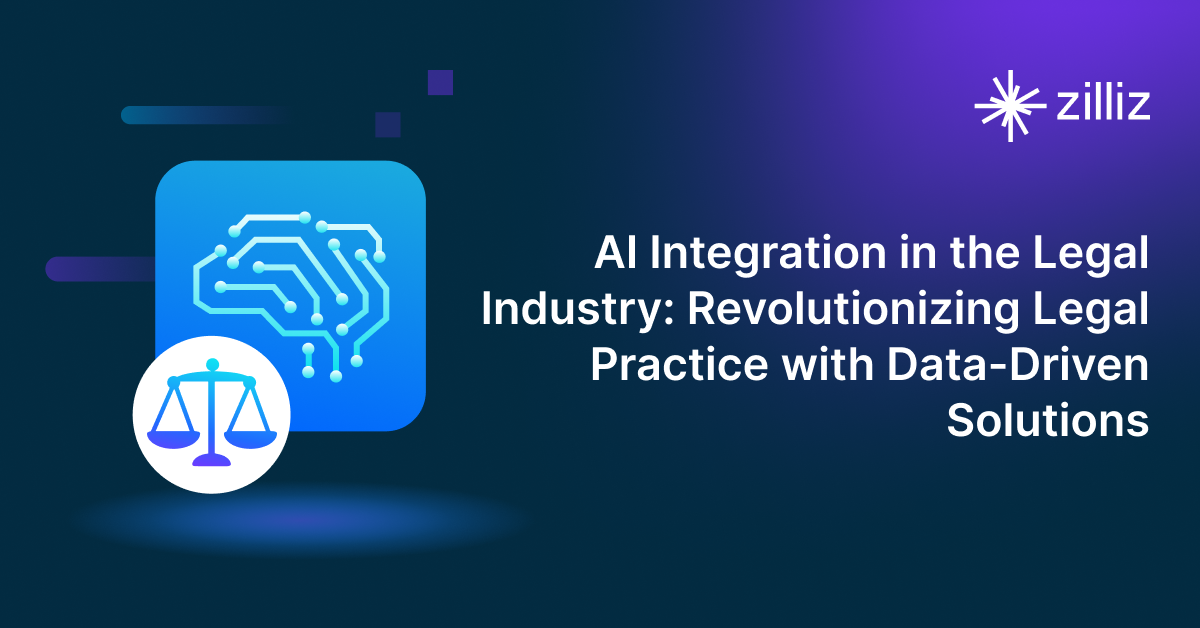
AI Integration in the Legal Industry: Revolutionizing Legal Practice with Data-Driven Solutions
Discover how AI and vector databases are revolutionizing legal work through advanced document processing, semantic search, and contract analysis capabilities.
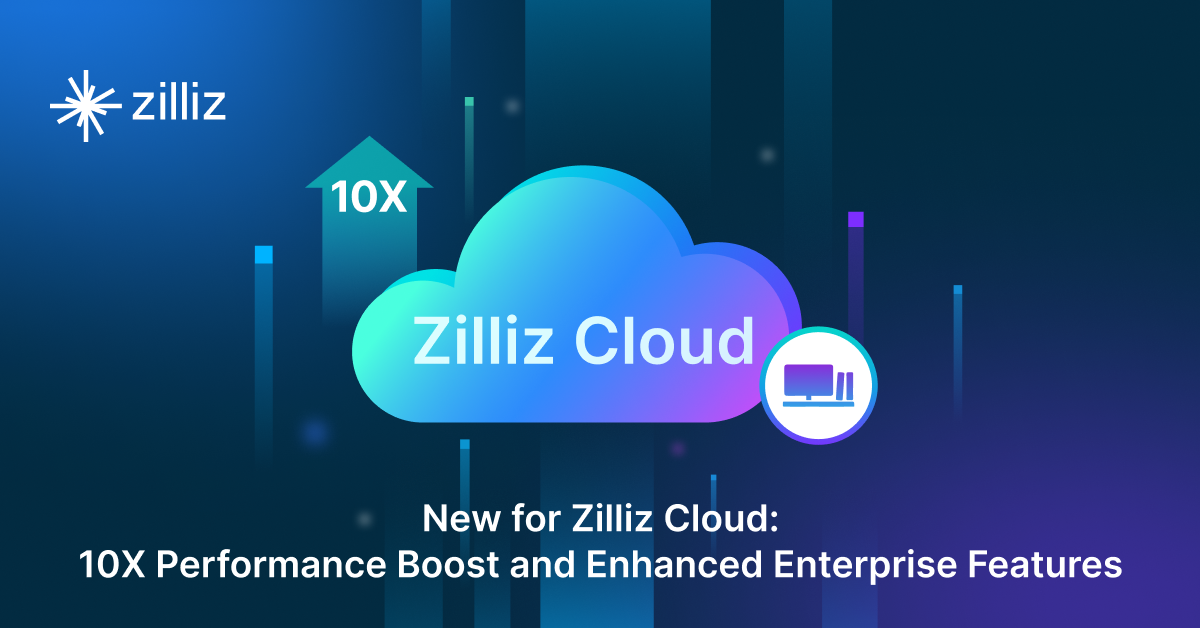
New for Zilliz Cloud: 10X Performance Boost and Enhanced Enterprise Features
A 10x faster Performance with Cardinal vector search engine, production-ready features including Multi-replica, Data Migration, Authentication, and more