The AI Revolution in Marketing: How Vector Databases Are Unlocking True Personalization
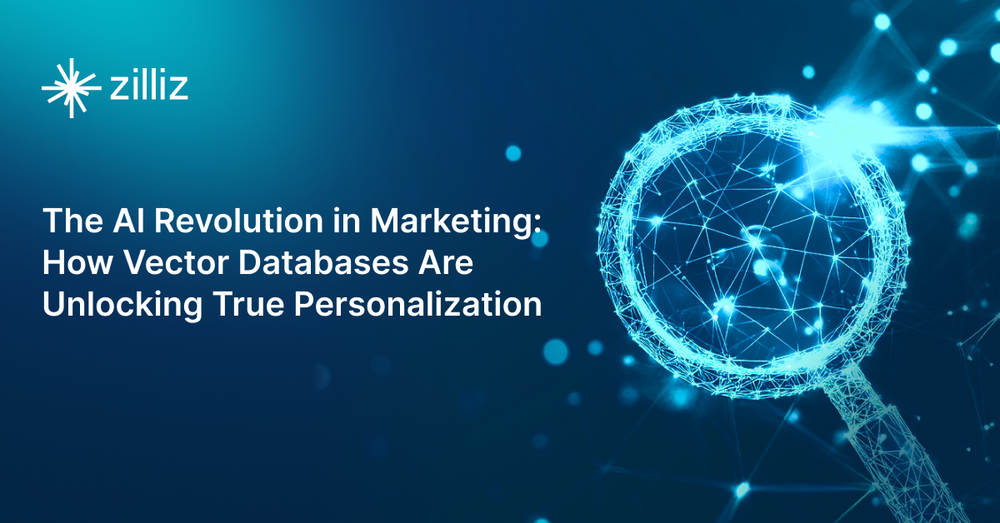
Introduction
By 2034, AI-powered marketing solutions won’t just be an advantage—they’ll be a necessity for competitive survival, according to Morningstar. This shift echoes past technological revolutions: just as word processors and spreadsheets led to longer documents and more complex decision-making rather than reducing work, the AI revolution is transforming marketing in ways that don’t simply automate tasks but expand possibilities.
The integration of AI and vector databases into marketing platforms marks a fundamental shift in how businesses engage with customers. These technologies process massive real-time data streams into actionable insights, unlocking levels of personalization and efficiency previously unimaginable. Yet, as with any technological leap, the challenge lies in harnessing AI effectively—balancing automation with creativity, personalization with originality, and efficiency with genuine human connection.
Current State & Challenges in AI Marketing Platforms
While AI has supercharged marketing capabilities, many platforms still fall short of delivering true intelligence. The problem? A widening gap between data collection and actionable insights, compounded by legacy architectures that weren’t designed for today’s AI-driven scale.
Key technical challenges include:
Data Overload: Marketing platforms ingest massive amounts of structured and unstructured data, but extracting real-time insights remains a bottleneck.
Fragmented Customer Journeys: Users interact across web, mobile, social, and email, yet stitching these touchpoints together for predictive modeling is difficult.
Personalization Limits: Rule-based segmentation lacks adaptability, while AI-driven personalization often struggles with sparse or biased data.
Manual Content Tagging & Classification: Relying on human-tagged metadata slows down asset retrieval and weakens recommendation systems.
Inefficient Search & Recommendations: Traditional keyword-based search fails to capture semantic intent, reducing relevance in content discovery and ad targeting.
For developers, the key question is: Will AI-driven marketing platforms actually improve decision-making, or just flood the system with more content?
A BCG/Harvard study found that ChatGPT improved marketers' performance by 40%. But instead of reducing workloads, this efficiency boost led to even more content production. Rather than freeing up time, AI allowed marketers to create and distribute content at unprecedented scale.
This shift can lead to two possible outcomes. On one hand, AI-powered marketing platforms enable true personalization, generating tailored emails, dynamic ad creatives, and customer-specific experience that drive engagement. Which is really great and exciting! However, on the other hand, if AI-generated content lacks originality, the sheer volume of material flooding the market risks making campaigns feel repetitive and indistinguishable. When content is trained on AI-generated data, the cycle of repetition accelerates, leading to homogenization rather than differentiation.
So how can you fix the problem of content overload and homogenization? Marketing platforms can help by using vector search to surface the most relevant, high-performing content rather than relying solely on AI to generate new material. Instead of flooding channels with repetitive, AI-generated text, marketers can retrieve and repurpose top-performing articles, case studies, or customer insights that align with their campaign goals. This allows for strategic content augmentation instead of blind regeneration.
Beyond reducing redundancy, vector search also enhances personalization. By leveraging embeddings, marketing platforms can match messaging with a user’s previous interactions, preferences, or sentiment, ensuring content is not just similar but actually relevant. This allows brands to deliver more engaging, differentiated experiences rather than contributing to an endless cycle of AI-recycled material.
Future State: AI-Powered Marketing That Actually Delivers
The next generation of AI-driven marketing platforms must go beyond simple content generation and static segmentation. To transform performance, developers need to create intelligent, scalable systems capable of adapting in real-time. Real personalization requires not just generating content but understanding the shifts in user behavior as they happen. Predictive AI is essential for this, enabling real-time recommendation engines to adjust dynamically to a customer’s actions. By continuously learning from behavior, predictive AI ensures marketing strategies stay relevant, helping brands personalize content without the constraints of static rules.
At the heart of this AI-driven personalization is vector search. Unlike traditional search, which relies on keyword matching, vector search utilizes semantic understanding to retrieve content based on customer intent. This makes recommendations and search results more intuitive and relevant. Furthermore, when paired with Retrieval-Augmented Generation (RAG), it enhances the power of large language models by integrating fresh, domain-specific knowledge rather than relying solely on outdated training data.
However, as AI-driven systems scale, infrastructure must evolve as well. Vector databases provide the necessary speed and scalability, enabling near-instant retrieval of massive datasets. Traditional relational databases simply cannot keep up with the demands of AI-powered marketing. Hybrid search, combining semantic vector search and traditional keyword search, optimizes accuracy and relevance. By incorporating metadata—such as user preferences, past interactions, and contextual details—AI is able to refine results, ensuring that search queries and recommendations are not only accurate but also deeply personalized.
While AI systems can automate many tasks, they must always work in harmony with human creativity. AI is an excellent assistive tool, enabling marketers to ideate, prototype, and test ideas more efficiently. However, creativity is still a fundamentally human trait. AI cannot replicate the nuanced thinking that allows marketers to craft unique, emotionally resonant messages. Over-relying on AI can lead to homogenized content, and research shows that excessive automation reduces the divergence of ideas by 40%. Marketers should retain control, using human-in-the-loop systems to fine-tune AI outputs, ensuring that their brand identity and message remain distinct and impactful.
The future of AI-powered marketing isn’t about replacing humans with automation; it’s about creating an ecosystem where AI enhances human creativity, making decision-making smarter and faster, while enabling real-time, scalable personalization that drives deeper engagement and measurable results.
Recommendations: Building Smarter AI-Driven Marketing Platforms
According to McKinsey’s "Connecting for Growth: A Makeover for Your Marketing Operating Model", most marketing organizations still struggle with AI adoption. Key gaps include:
Only 30% can effectively adjust marketing spend dynamically.
Only 23% have mature personalized targeting capabilities.
36% gap in evaluating automation opportunities.
30% gap in AI strategy implementation.
To close these gaps, developers need to create platforms that enable smarter, AI-driven marketing decisions in real-time.
Real-Time Analytics and AI-Driven Decision-Making
Many marketing teams still struggle with adjusting their budget dynamically based on performance. This is where real-time analytics powered by AI come in. Imagine having the ability to adjust spending on the fly, ensuring that your marketing budget is always being optimized. With AI-driven forecasting and intelligent allocation, you can bridge this gap and help marketers make the most of their resources.
But it's not just about moving away from static reports. We need to shift from rule-based analytics that provide limited insights to AI-powered platforms that deliver deeper, smarter recommendations. Currently, 64% of marketing decisions aren't driven by analytics—an opportunity for developers to step in and drive change by embedding AI-driven insights directly into marketing workflows.
Seamless Data Integration Across Silos
Another major hurdle for marketers is fragmented data. With so many touchpoints and platforms collecting customer information, the lack of a unified data strategy can hold back AI's full potential. Developers can solve this by creating real-time data pipelines that bring all this information together, providing a complete, actionable view of the customer journey. When all data sources are unified, marketers have a clear picture of their audience and can make informed decisions faster.
On top of that, integrating AI-native data processing into these pipelines is crucial. Automating the transformation and optimization of data in real-time allows for the kind of hyper-personalization that today’s marketers demand. This isn’t about managing data better—it's about creating an environment where AI can work alongside the marketing team to provide insights that are both relevant and timely.
AI/GenAI for Scalable Personalization and Optimization
Personalization is where AI truly shines. Yet, only 23% of marketers have reached the level of deep personalization they desire. Developers have an opportunity to build solutions that go beyond basic segmentation. With vector search-powered recommendation engines, you can offer more targeted content, product suggestions, and experiences for each individual customer, moving personalization from a static practice to something dynamic and meaningful.
And it doesn’t stop at targeting—AI can help streamline the creative side of marketing as well. Tools that assist with content creation are making a big impact, improving creative workflows by 39%. Instead of replacing creativity, AI tools can enhance it by providing smart suggestions, automating repetitive tasks, and allowing creative teams to focus on what they do best.
Similarly, optimizing media and ad spend is a huge opportunity for AI. With marketers increasingly relying on AI-powered media optimization, platforms can automatically adjust ad placements to ensure higher ROI. As AI handles these adjustments in real-time, marketing teams can focus on strategy and innovation, knowing that the technology is working behind the scenes to maximize effectiveness.
Architecting for Scalability and Cross-Functional Collaboration
Finally, developers must ensure that AI-driven marketing solutions can scale across regions, brands, and customer needs. This requires building flexible architectures that adapt seamlessly to different market conditions. A global marketing campaign might look very different from a regional initiative, and AI solutions should be able to pivot quickly to meet these varying demands.
Supporting external data partnerships also plays a key role. APIs and third-party integrations allow marketing teams to tap into a broader range of data sources, giving them a more complete picture of their customers. By connecting various data streams, marketers can create richer, more accurate customer profiles.
But none of this will work without strong collaboration between engineering and marketing teams. Developers should focus on building platforms that are intuitive enough for marketers to experiment with AI models without requiring deep technical expertise. The goal is to break down the barriers that currently separate technical and marketing teams, creating an environment where both sides can work together to drive innovation.
Next Steps: What Developers Can Do Today
Enhance your AI-powered marketing platform with real-time decision-making
Implement vector search + hybrid search to improve recommendations.
Use Retrieval-Augmented Generation (RAG) for fresher, domain-specific AI outputs.
Build AI-driven budget and media optimization tools.
Solve data integration challenges
Develop real-time data pipelines that unify customer interactions.
Leverage LLMs for automated content tagging and classification.
Scale AI-driven marketing solutions
Design flexible architectures that support multi-brand and multi-region marketing needs.
Ensure AI systems seamlessly integrate with external data sources and APIs.
Join the Conversation & Start Building
Explore Zilliz Cloud for scalable vector search and AI-powered recommendations.
Read our technical guide on integrating AI into marketing platforms.
Join our developer community to discuss real-world AI challenges and solutions.
- Introduction
- Current State & Challenges in AI Marketing Platforms
- Future State: AI-Powered Marketing That Actually Delivers
- Recommendations: Building Smarter AI-Driven Marketing Platforms
- Next Steps: What Developers Can Do Today
Content
Start Free, Scale Easily
Try the fully-managed vector database built for your GenAI applications.
Try Zilliz Cloud for FreeKeep Reading
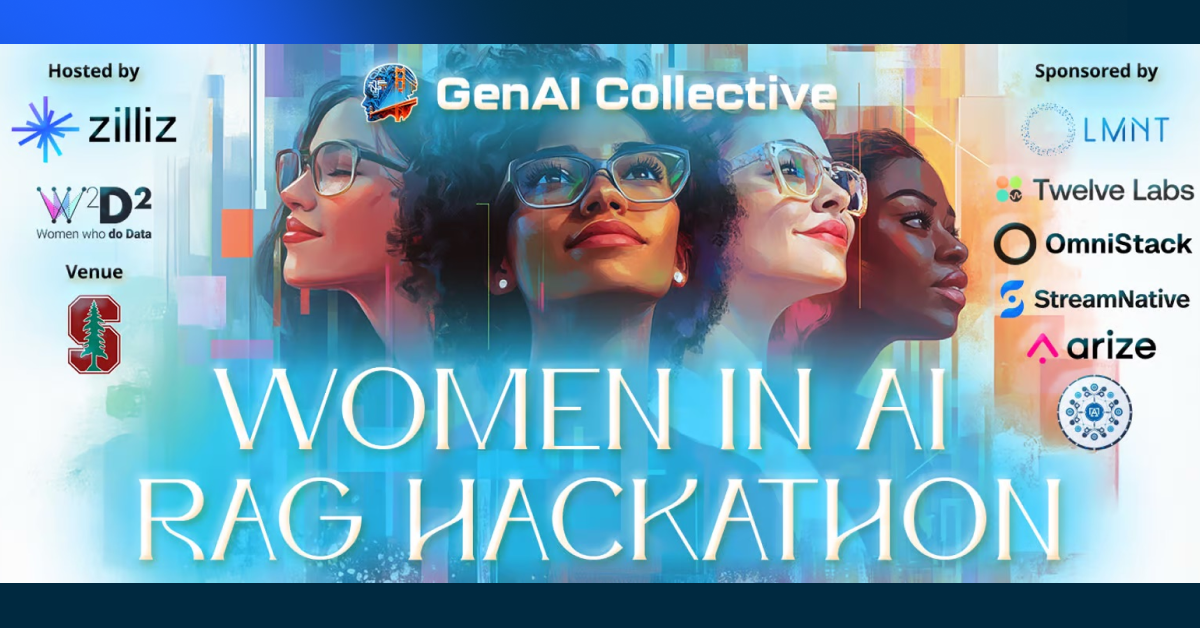
Empowering Innovation: Highlights from the Women in AI RAG Hackathon
Over the course of the day, teams built working RAG-powered applications using the Milvus vector database—many of them solving real-world problems in healthcare, legal access, sustainability, and more—all within just a few hours.
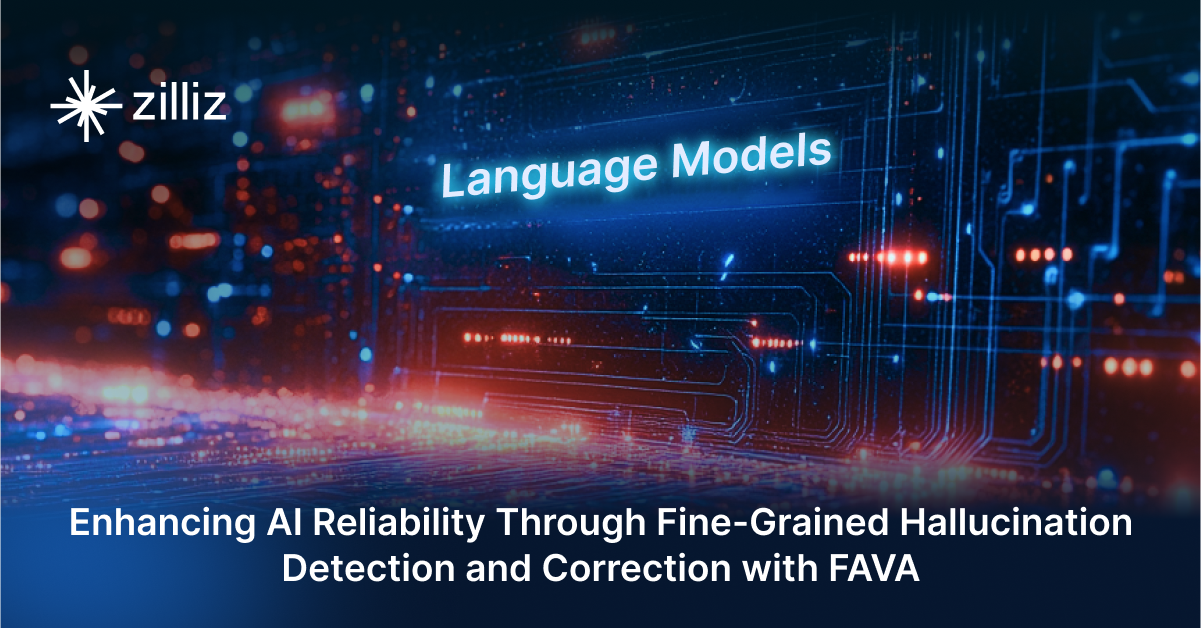
Enhancing AI Reliability Through Fine-Grained Hallucination Detection and Correction with FAVA
In this blog, we will explore the nature of hallucinations, the taxonomy that provides a framework for categorizing them, the FAVABENCH dataset designed for evaluation, and how FAVA detects and corrects errors.
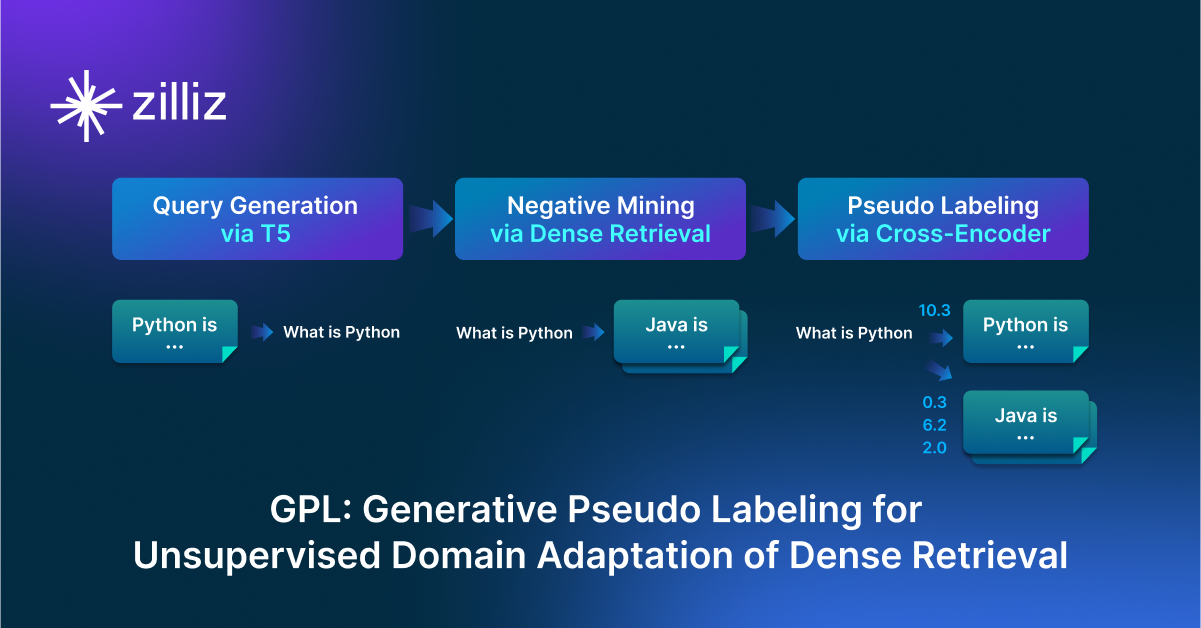
GPL: Generative Pseudo Labeling for Unsupervised Domain Adaptation of Dense Retrieval
GPL is an unsupervised domain adaptation technique for dense retrieval models that combines a query generator with pseudo-labeling.