The K-Nearest Neighbors (KNN) algorithm can be used for image segmentation by classifying each pixel in an image based on its feature values. Features can include pixel intensity, color, texture, or even spatial information like pixel coordinates. To apply KNN, prepare a dataset of labeled pixels where each pixel’s features and class (segment) are known. During segmentation, each pixel in the image is assigned to the class most common among its K-nearest neighbors in the feature space. Preprocessing is critical for KNN-based segmentation. Normalize the pixel features to ensure all attributes contribute equally to distance calculations. Use a distance metric like Euclidean distance to measure similarity between pixel features. For images with complex patterns, including additional features like texture descriptors or output from convolutional layers of a neural network can improve segmentation accuracy. While KNN is simple and effective for small-scale problems, it has limitations for high-dimensional data, such as computational inefficiency and sensitivity to irrelevant features. It also struggles with boundary accuracy in complex segmentation tasks. Despite these drawbacks, KNN is a useful baseline method and is particularly suitable for teaching or prototype development before moving to more advanced algorithms like U-Net or Mask R-CNN.
How will the KNN algorithm work for image segmentation?
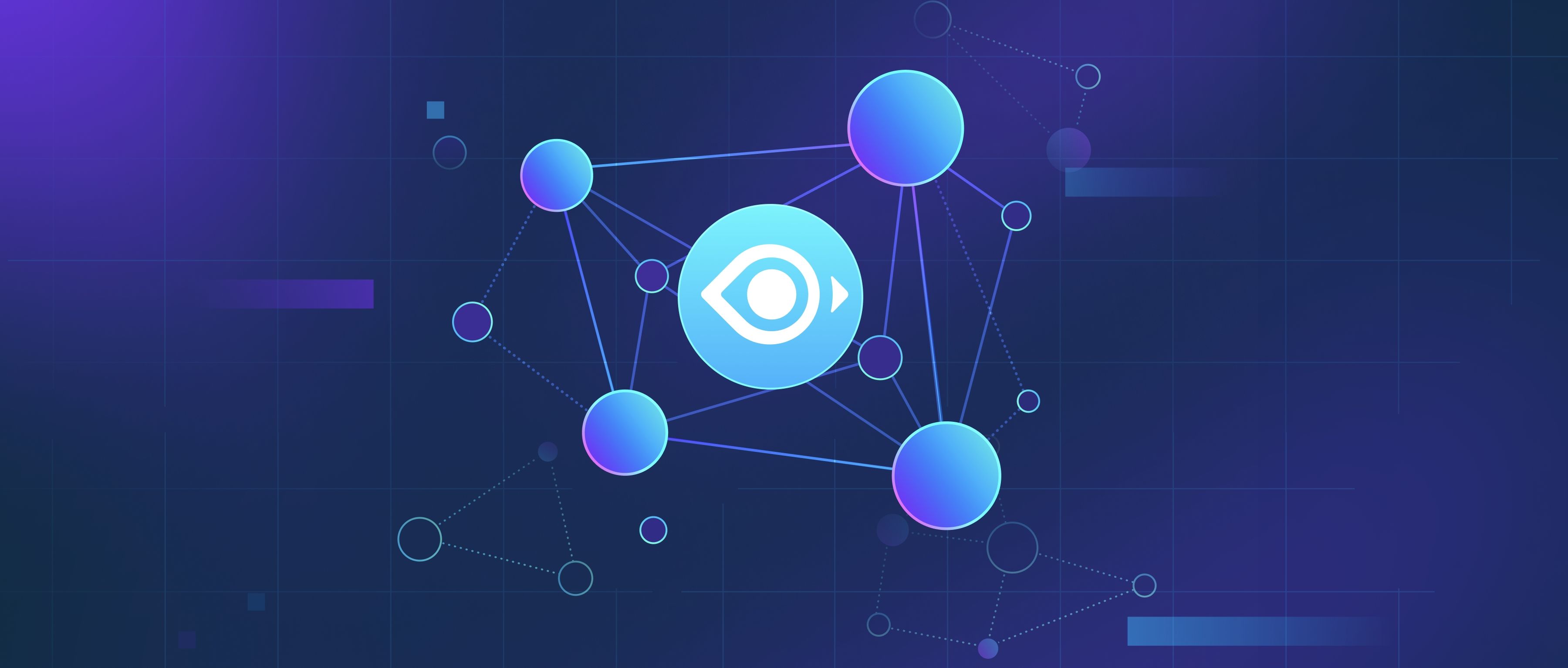
- Getting Started with Milvus
- Large Language Models (LLMs) 101
- Natural Language Processing (NLP) Advanced Guide
- The Definitive Guide to Building RAG Apps with LangChain
- Vector Database 101: Everything You Need to Know
- All learn series →
Recommended AI Learn Series
VectorDB for GenAI Apps
Zilliz Cloud is a managed vector database perfect for building GenAI applications.
Try Zilliz Cloud for FreeKeep Reading
What is the best motion tracking system for object detection?
The best motion tracking system for object detection depends on the specific requirements of the task, but several optio
What is the LangChain agent, and how does it work?
LangChain agents are components within the LangChain framework designed to assist in decision-making processes where mul
How is Apache Pulsar different from Apache Kafka?
Apache Pulsar and Apache Kafka are both popular distributed messaging systems, but they differ in their architecture and