HumanSignal
HumanSignal Offers Faster Data Sourcing & Labeling with Milvus and AWS
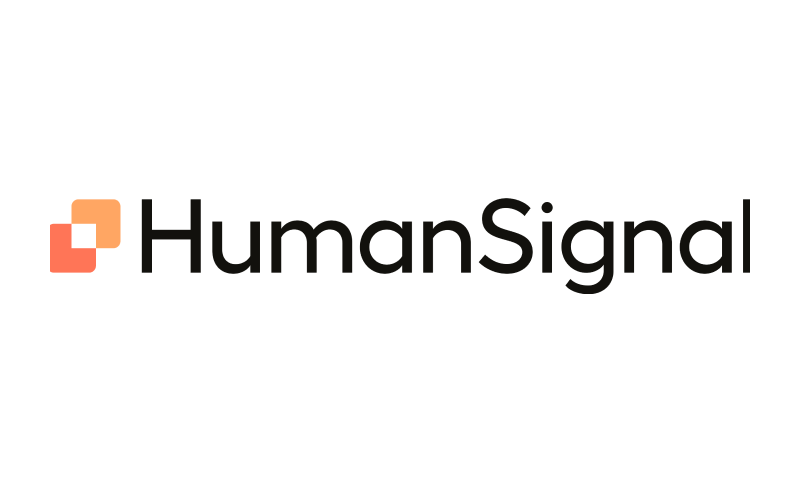
Super Low Latency
in semantic search
Enhanced Scalability
in vector data storage
Faster and More Reliable
in image indexing
Better User Experience
with a streamlined operation process
About HumanSignal
HumanSignal, formerly Heartex, empowers Machine Learning and Artificial Intelligence development through its flagship open-source data labeling platform, Label Studio. Since its inception in 2019 by a team of data scientists and engineers, HumanSignal has addressed the critical challenge of model accuracy arising from substandard training data. Label Studio was created to enable domain experts within organizations to annotate and manage training data efficiently. The platform emphasizes user-friendly interfaces, adaptability, and collaborative processes to bolster internal data labeling capabilities, thereby significantly improving model precision. As the most popular data labeling platform on GitHub, Label Studio has supported over 200,000 users in labeling upwards of 250 million data items, serving as a pivotal tool in the production ML/AI strategies of leading enterprises such as Bombora, Geberit, Outreach, Trivago, Wyze, and Zendesk, among others.
The Challenges: Building A New Way to Navigate and Label Data Lakes
A major challenge in data labeling revolves around choosing the correct pieces of data to label in the first place. Many AI projects have massive data lakes full of unstructured data, and it can be challenging to sort through the many items within the data lake to choose the ones that are the most relevant and important for inclusion in a training or ground truth dataset. Traditional methods, such as basic heuristics and SQL queries, are time-consuming and manual and usually fail to pinpoint the most impactful items needed for high-quality training sets.
Consequently, many data science teams resort to smaller, less representative data samples, which degrades the accuracy and effectiveness of ML/AI models. Furthermore, such constraints slow down the model development process, impeding progress and the ability to bring advanced AI solutions to a competitive, rapidly evolving technological environment.
Because of these challenges, HumanSignal started working on a major new Label Studio Enterprise feature designed to alleviate many of these issues - Data Discovery.
The Solutions: Enhancing Data Discovery with Milvus and AWS
In the process of building this new Data Discovery feature, HumanSignal turned to Zilliz’s open source offering Milvus because of its unique capability to support a wide array of indexing algorithms - a feature not commonly offered by other vector database vendors. This flexibility allowed HumanSignal to significantly enhance their semantic search functionality within their Data Discovery tool, transitioning through various indexing algorithms—from Hierarchical Navigable Small World (HNSW) for initial efficiency to DiskANN for optimized memory usage, and finally to IVF_SQ8 for improved performance.
The deployment of Milvus on Amazon Web Services (AWS) using the Elastic Kubernetes Service (EKS) further amplified this solution's efficacy. Utilizing Milvus's helm chart, HumanSignal seamlessly integrated this robust vector database into their cloud infrastructure, leveraging the scalability and reliability of AWS to support their large-scale data processing needs. This strategic combination streamlined the deployment process and ensured that the Data Discovery tool could efficiently manage and process vast amounts of data for Label Studio users.
The Results: A Streamlined Data Labeling and Enhanced Model Development
Integrating Milvus into HumanSignal's Data Discovery feature has been critical to achieving super-low latency in semantic search operations. This improvement has allowed HumanSignal to offer a streamlined new process for users to identify relevant data subsets for labeling, making the process much faster than traditional search methods. Furthermore, Milvus improved the speed and reliability of image indexing, a crucial area previously fraught with challenges. This advancement means that Data Discovery users can now enjoy faster and more dependable image processing, which has significantly boosted the quality and accuracy of their training sets, directly benefiting ML/AI model performance.
The Zilliz Milvus and AWS stack has been crucial to HumanSignal by providing a scalable and robust platform for vector data storage. It addressed the immediate challenges they faced while building their Data Discovery feature and has positioned HumanSignal for continued innovation and growth in AI and ML, underscoring the transformative power of combining cutting-edge technologies in AI and the Cloud.