What's New in Milvus 2.2.10 and 2.2.11
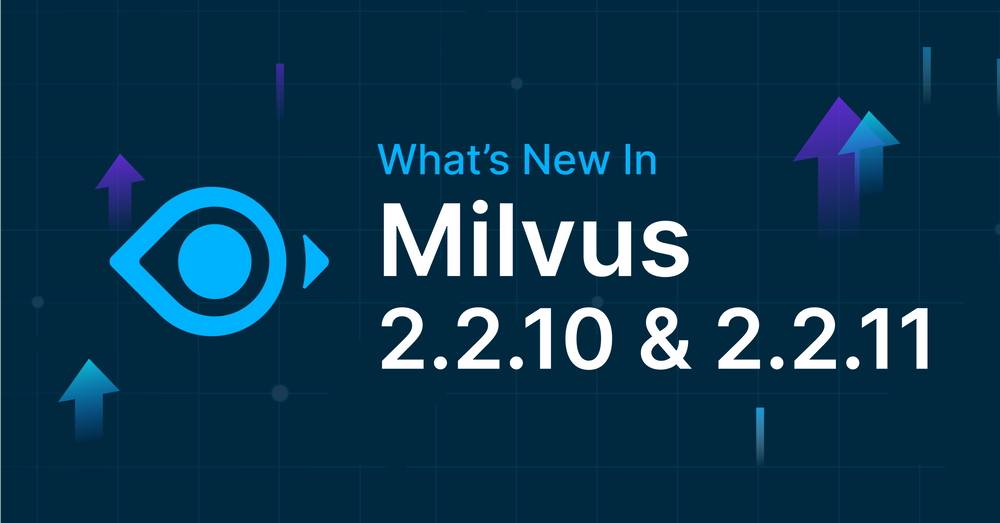
We are excited to announce the release of Milvus versions 2.2.10 and 2.2.11! These latest versions come loaded with numerous enhancements that significantly improve the product's functionality and user experience. We have incorporated feedback from our community of users and made updates to enhance the performance and user experiences further! This blog post will highlight some of the more prominent features. For a complete list of changes, please check the release notes.
📦 PyPI: https://pypi.org/project/milvus/
📚 Docs: https://milvus.io/docs
🛠️ Release Notes:
🐳 Docker Image: docker pull
🚀 Release: milvus-2.2.11
We are continuously enhancing RBAC to expand the security functionalities across different aspects of the system. In version 2.2.10, we introduced the 'FlushAll' function and Database API to the RBAC capabilities. These updates offer improved control over permissions and access to sensitive operations, ultimately enhancing the system's overall security.
Additionally, both versions, 2.2.10 and 2.2.11, introduced several optimizations and upgrades. Notably, in version 2.2.11, we optimized disk usage for RocksMq by enabling zstd compression starting from level 2. This enhancement significantly improves storage efficiency. Additionally, in version 2.2.10, we made a major improvement by replacing the CGO payload writer with a Go payload writer. This change reduced memory usage, leading to improved system performance and stability.
Please note that there are several bug fixes and performance enhancements in the Milvus 2.2.10 & 2.2.1 releases, so check out the release notes for more details.
Summary
In addition to all of the features listed above, Milvus 2.2.10 & 2.2.11 include several bug fixes and improvements. To learn more:
See the release notes for version 2.2.10 & version 2.2.11 for the complete list of changes Download Milvus and get started.
- Summary
Content
Start Free, Scale Easily
Try the fully-managed vector database built for your GenAI applications.
Try Zilliz Cloud for FreeKeep Reading
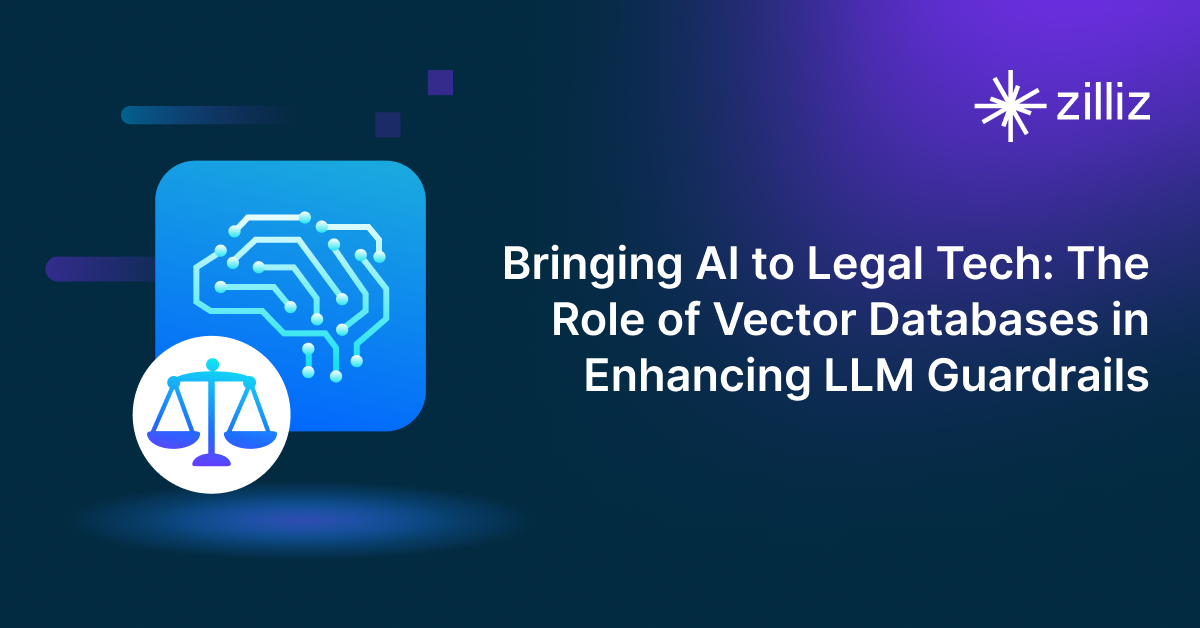
Bringing AI to Legal Tech: The Role of Vector Databases in Enhancing LLM Guardrails
Discover how vector databases enhance AI reliability in legal tech, ensuring accurate, compliant, and trustworthy AI-powered legal solutions.
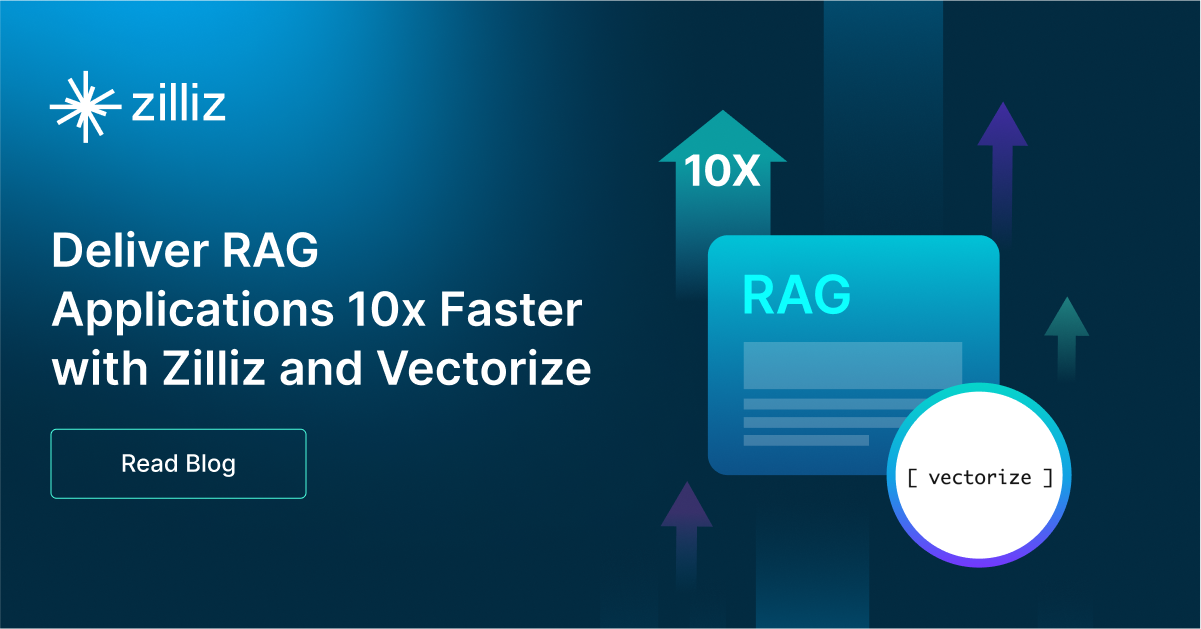
Deliver RAG Applications 10x Faster with Zilliz and Vectorize
Zilliz Cloud delivers reliable vector storage and search, while Vectorize automates your RAG pipelines and keeps your embeddings up-to-date.
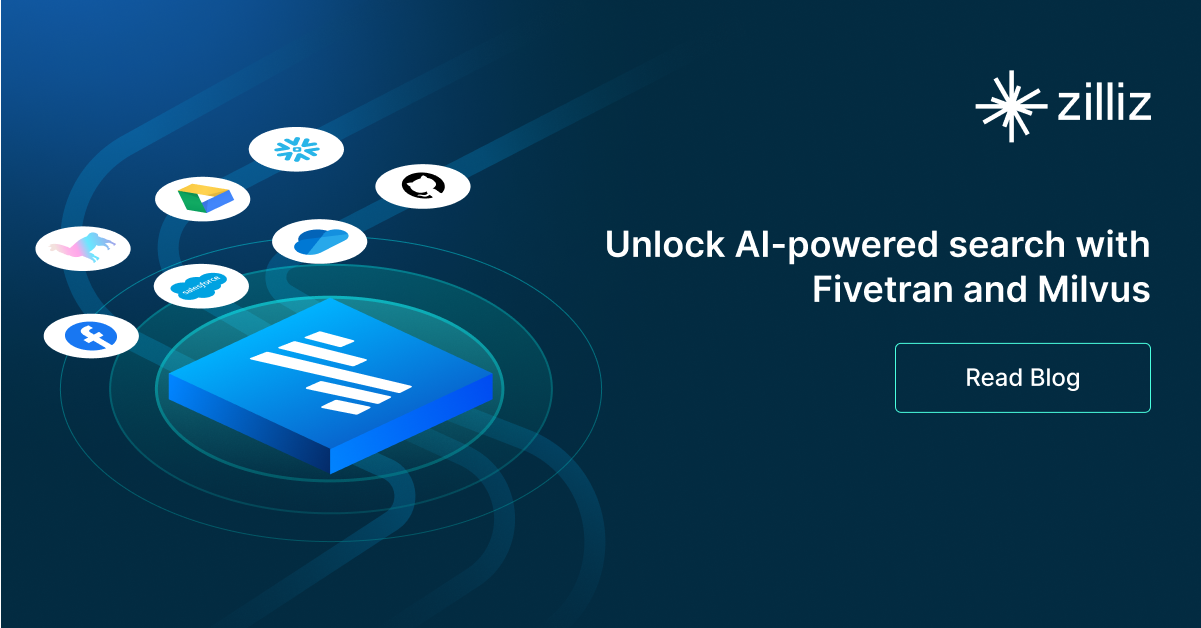
Unlock AI-powered search with Fivetran and Milvus
Fivetran supports the Milvus vector database as a destination, making it easier to onboard every data source for RAG and AI-powered search.