Milvus Metadata Filtering: JSON and Metadata Filtering in Milvus
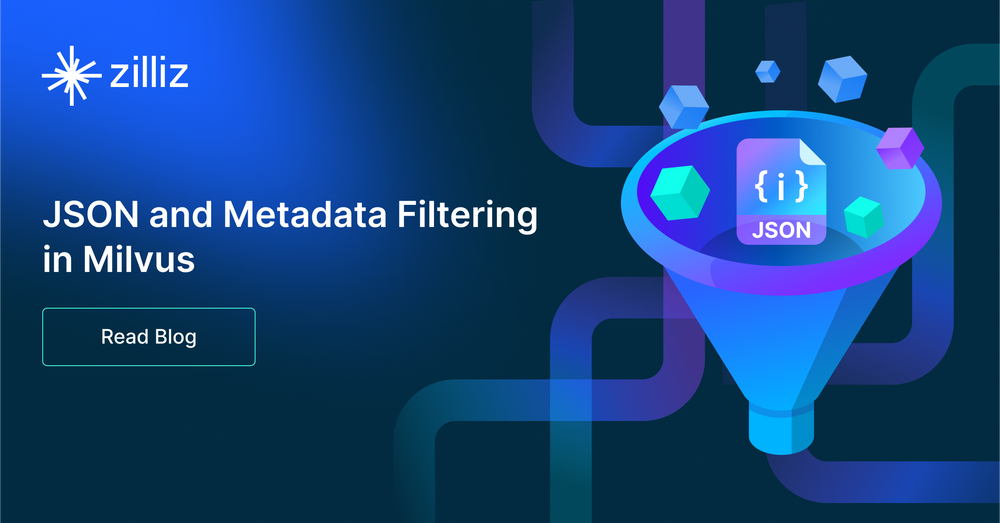
JSON, or JavaScript Object Notation, is a flexible data format for storage and transmission. It uses key-value pairs adaptively, making it perfect for NoSQL databases and API results. Because of its flexibility, it is a popular data format. Search parameters are crucial for defining the specifics of each search request, such as the vector fields used, the query vectors, and the limits on the number of results returned.
Many Milvus demos start with raw text data, such as .txt, .pdf, or .csv file types. But did you know you can upload and work with raw JSON with Milvus? See this JSON documentation page for more details.
Milvus Client is a wrapper around the Milvus collection object that uses a flexible JSON “key”:value format to allow schema-less data definitions. See the Milvus Client documentation for more information.
Schema-less Milvus Client and Zilliz’s free tier cloud is a great way to use Milvus quickly! Milvus Client is nearly as fast as defining a full schema upfront but with less error-prone coding. Compared to “enable_dynamic_field”, Milvus Client is much faster, offering a smoother approach to using less schema-definition up front. The schema-less schema is:
- id (str): Name of the primary key field.
- vector (str): Name of the vector field.
That’s it! The rest of the fields can be determined flexibly when the data is inserted into Milvus.
To make this more concrete, let’s jump into some code examples of how to use Milvus Client. The full code is in my Bootcamp github repo.
Code example - upload raw JSON data directly into Milvus
The raw data itself could be JSON format. This is useful, for example, if your data comes from a NoSQL database such as MongoDB. Below the JSON data used is the IMDB movie genre classification from Kaggle
# !pip install numpy pandas json pprint pymilvus torch sentence-transformers
# Import common libraries.
import pandas as pd
import json
# Read JSON data.
df = pd.read_json('data/tiny_parsed_data.json')
# Concatenate Title and Description into 'text' column.
df['text'] = df['title'] + ' ' + df['description']
display(df.head(2))
Start up a Zilliz cloud free trial server. You can have up to 2 collections, each up to 1 million vectors, on a free trial at a time. Code in this notebook uses fully-man
- Choose the default "Starter" option when you provision > Create collection > Give it a name > Create cluster and collection.
- On the Cluster main page, copy your
API Key
and store it locally in a .env variable ‘ZILLIZ_API_KEY’. - Also on the Cluster main page, copy the
Public Endpoint URI
.
import os
from pymilvus import connections, utility
TOKEN = os.getenv("ZILLIZ_API_KEY")
# Connect to Zilliz cloud using endpoint URI and API key TOKEN.
CLUSTER_ENDPOINT="https://in03-xxxx.api.gcp-us-west1.zillizcloud.com:443"
connections.connect(
alias='default',
token=TOKEN,
uri=CLUSTER_ENDPOINT,)
# Check if the server is ready and get collection name.
print(f"Type of server: {utility.get_server_version()}")
Choose an embedding model. Below, I’ve chosen one from HuggingFace. I’m running this on my laptop, so I have to make sure DEVICE=cpu.
import torch
from sentence_transformers import SentenceTransformer
# Initialize torch settings
torch.backends.cudnn.deterministic = True
DEVICE = torch.device('cuda:3' if torch.cuda.is_available() else 'cpu')
# Load the model from huggingface model hub.
model_name = "WhereIsAI/UAE-Large-V1"
encoder = SentenceTransformer(model_name, device=DEVICE)
# Get the model parameters and save for later.
EMBEDDING_DIM = encoder.get_sentence_embedding_dimension()
MAX_SEQ_LENGTH_IN_TOKENS = encoder.get_max_seq_length()
# View model parameters.
print(f"model_name: {model_name}")
print(f"EMBEDDING_DIM: {EMBEDDING_DIM}")
print(f"MAX_SEQ_LENGTH: {MAX_SEQ_LENGTH}")
Import MilvusClient and create the collection. Notice we don’t have to specify a schema! In addition, you can just use our system default index, called AUTOINDEX. On open source and free tier the default is HNSW. On paid Zilliz cloud, AUTOINDEX will be further optimized using proprietary indexes.
from pymilvus import MilvusClient
import pprint
# Set the Milvus collection name.
COLLECTION_NAME = "imdb_metadata"
# Use no-schema Milvus client.
mc = MilvusClient(
uri=CLUSTER_ENDPOINT,
token=TOKEN)
# Check if the collection already exists, if so drop it.
has = utility.has_collection(COLLECTION_NAME)
if has:
drop_result = utility.drop_collection(COLLECTION_NAME)
print(f"Successfully dropped collection: `{COLLECTION_NAME}`")
# Create the collection.
mc.create_collection(COLLECTION_NAME,
EMBEDDING_DIM,
consistency_level="Eventually",
auto_id=True,
overwrite=True,
# skip setting params, if using AUTOINDEX
)
print(f"Successfully created collection: `{COLLECTION_NAME}`")
pprint.pprint(mc.describe_collection(COLLECTION_NAME))
A simple chunking strategy is to keep the ‘text’ field as a single chunk unless it exceeds 512 characters in length. Below, we can see the JSON data text field was pretty short. No rows had to be split up into smaller chunks.
# Use the embedding model parameters.
chunk_size = 512
chunk_overlap = np.round(chunk_size * 0.10, 0)
# Chunk a batch of data from pandas DataFrame and inspect it.
BATCH_SIZE = 100
batch = imdb_chunk_text(BATCH_SIZE, df, chunk_size)
display(batch.head(2))
Now that we have chunks of text, vector embeddings for each chunk of text, and all the original metadata, let’s insert that data into Milvus vector database.
# Convert the DataFrame to a list of dictionaries
chunk_list = batch.to_dict(orient='records')
# Insert data into the Milvus collection.
start_time = time.time()
insert_result = mc.insert( COLLECTION_NAME, data=chunk_list, progress_bar=True )
end_time = time.time()
print(f"Milvus Client insert time for {batch.shape[0]} vectors: {end_time - start_time} seconds")
Let’s ask a question and retrieve answers from our movie data.
SAMPLE_QUESTION = "Dystopia science fiction with a robot."
# Embed the question using the same encoder.
query_embeddings = _utils.embed_query(encoder, [SAMPLE_QUESTION])
TOP_K = 2
# Run semantic vector search using your query and the vector database.
start_time = time.time()
results = mc.search(
COLLECTION_NAME,
data=query_embeddings,
output_fields=OUTPUT_FIELDS,
limit=TOP_K,
consistency_level="Eventually",
filter='film_year >= 2019', )
elapsed_time = time.time() - start_time
print(f"Milvus Client search time for {len(chunk_list)} vectors: {elapsed_time} seconds")
search time
Looping through the top 2 results, we see.
That looks pretty good. But what if we want to do some metadata filtering on the JSON array ‘Genres’?
Metadata filtering across JSON fields and JSON arrays
A new feature in Milvus 2.3 is the ability to filter raw JSON metadata. Below, I’ll show an example using metadata filtering with a field and an array.
Let’s say I wanted to see older, more retro movies and strictly the Sci-Fi genre.
SAMPLE_QUESTION = "Dystopia science fiction with a robot."
# Embed the question using the same encoder.
query_embeddings = _utils.embed_query(encoder, [SAMPLE_QUESTION])
TOP_K = 2
# Run semantic vector search using your query and the vector database.
start_time = time.time()
results = mc.search(
COLLECTION_NAME,
data=query_embeddings,
output_fields=OUTPUT_FIELDS,
limit=TOP_K,
consistency_level="Eventually",
filter='json_contains(Genres, "Sci-Fi") and film_year < 2019',
)
elapsed_time = time.time() - start_time
print(f"Milvus Client search time for {len(chunk_list)} vectors: {elapsed_time} seconds")
Looping through the top 2 results, now the movie recommendations have changed to match the filter.
Many Milvus demos start with raw text data such as .txt, .pdf, or .csv file types. This time we saw how to load JSON data directly into a Milvus vector database collection. We also saw how to use the handy metadata filtering on JSON fields and thejson_contains()
filtering on JSON array data types. Full code for this blog article is in my Bootcamp GitHub repo.
Introduction to Hybrid Search
Hybrid search is a powerful feature in Milvus that allows users to combine multiple vector fields into a single search. This feature is particularly useful in complex search scenarios where an entity can be represented by multiple, diverse vectors. By integrating the results of multiple vector searches using reranking strategies, hybrid search enables users to achieve higher accuracy and more relevant results.
In Milvus, hybrid search can be used to combine different types of data, such as text, images, and audio, into a single search query. This allows users to perform more comprehensive searches and retrieve more relevant results. For example, a hybrid search can combine text embeddings with image embeddings to find products that match both a textual description and a visual example.
To use hybrid search effectively in Milvus, it is important to understand the different vector fields and how they can be combined. By carefully selecting and combining vector fields, users can improve the accuracy and relevance of their search results. Additionally, using reranking strategies can help to further refine the search results and ensure that the most relevant results are returned.
Managing Data with Metadata
Metadata plays a crucial role in managing data in Milvus. Metadata is used to describe the structure and organization of data, making it easier to search, filter, and analyze. In Milvus, metadata is used to manage data files, including information about the data, such as the collection name, vector field, and index type.
By using metadata effectively, users can improve the performance and accuracy of their searches. Metadata allows users to organize their data in a way that makes it easy to retrieve and analyze. For example, by using metadata to tag data with relevant keywords, users can quickly filter and search for specific data points.
In addition to improving search performance, metadata can also help to ensure data integrity and consistency. By using metadata to track changes and updates to data, users can ensure that their data remains accurate and up-to-date. This is particularly important in large-scale data environments where data is constantly being updated and modified.
JSON and Metadata Filtering
JSON (JavaScript Object Notation) is a flexible data format that is widely used in NoSQL databases and API results. Milvus supports uploading and working with raw JSON data, making it easy to integrate with other systems. Metadata filtering is a powerful feature in Milvus that allows users to filter raw JSON metadata based on specific conditions.
By using metadata filtering, users can improve the accuracy of their searches and reduce the amount of irrelevant data. For example, users can filter JSON data based on specific fields or values, such as filtering movies by genre or release year. This allows users to perform more targeted searches and retrieve more relevant results.
In Milvus, metadata filtering can be used in combination with vector search to further refine search results. By applying metadata filters to vector search queries, users can ensure that only the most relevant data is returned. This can help to improve search accuracy and reduce the amount of irrelevant data that needs to be processed.
Building and Managing Indexes for Vector Search
Index building is a critical step in optimizing vector search performance in Milvus. An index is a data structure that improves the speed of data retrieval by allowing the database to quickly locate specific data. In Milvus, indexes can be built on vector fields to improve the performance of vector searches.
There are different types of indexes available in Milvus, each with its own advantages and use cases. For example, the HNSW (Hierarchical Navigable Small World) index is commonly used for high-dimensional vector searches due to its efficiency and accuracy. Other index types, such as IVF (Inverted File) and PQ (Product Quantization), offer different trade-offs between search speed and accuracy.
To build and manage indexes effectively, it is important to understand the characteristics of the data and the specific search requirements. By selecting the appropriate index type and tuning the index parameters, users can significantly improve the performance of their searches. Additionally, regularly updating and maintaining indexes can help to ensure that search performance remains optimal as the data evolves.
Optimizing Hybrid Search Performance
Hybrid search performance can be optimized by using various techniques, including index building, metadata filtering, and query optimization. By building indexes on vector fields, users can improve the performance of vector searches. Indexes allow the database to quickly locate relevant data, reducing the time required to perform searches.
Metadata filtering is another important technique for optimizing hybrid search performance. By using metadata filters, users can reduce the amount of irrelevant data that needs to be processed, improving search accuracy and speed. For example, filtering data based on specific fields or values can help to narrow down the search results and ensure that only the most relevant data is returned.
Query optimization is also crucial for improving hybrid search performance. By optimizing the structure and parameters of search queries, users can reduce the computational resources required for searches and improve performance. This can include techniques such as batching queries, using efficient data structures, and tuning query parameters.
In this section, we have discussed best practices for optimizing hybrid search performance in Milvus. By using techniques such as index building, metadata filtering, and query optimization, users can improve the accuracy and speed of their searches, ensuring that they retrieve the most relevant results.
- Code example - upload raw JSON data directly into Milvus
- Metadata filtering across JSON fields and JSON arrays
- Introduction to Hybrid Search
- Managing Data with Metadata
- JSON and Metadata Filtering
- Building and Managing Indexes for Vector Search
- Optimizing Hybrid Search Performance
Content
Start Free, Scale Easily
Try the fully-managed vector database built for your GenAI applications.
Try Zilliz Cloud for FreeKeep Reading
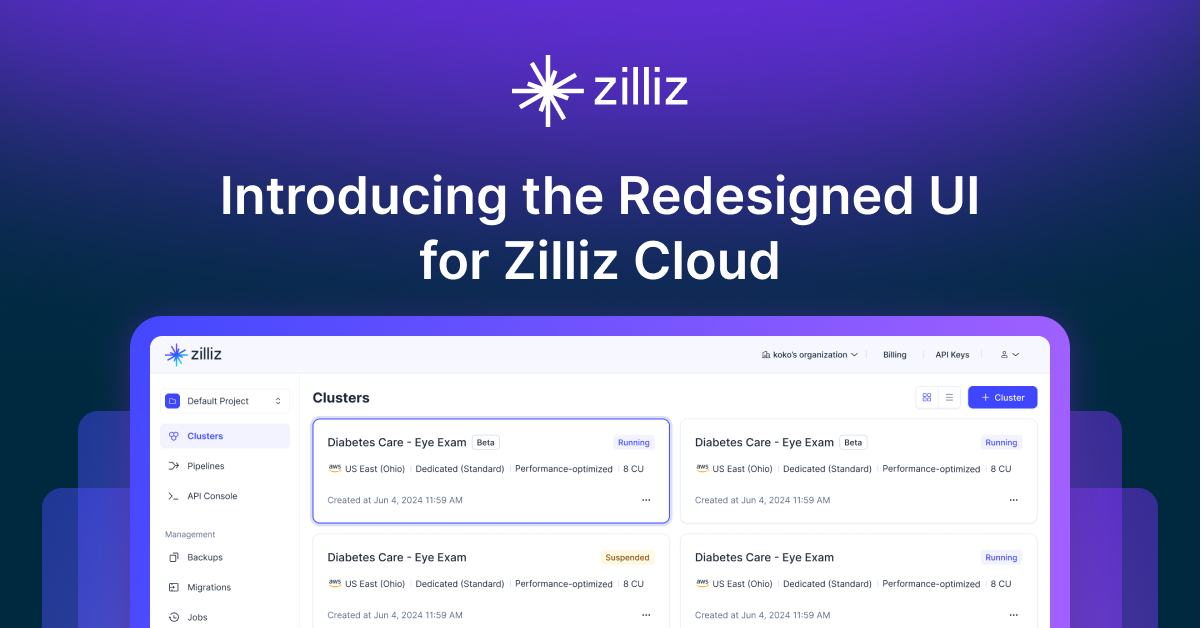
Zilliz Cloud’s Redesigned UI: A Streamlined and Intuitive User Experience
This new UI is cleaner, more intuitive, and specifically designed to streamline workflows, reduce cognitive load, and boost productivity
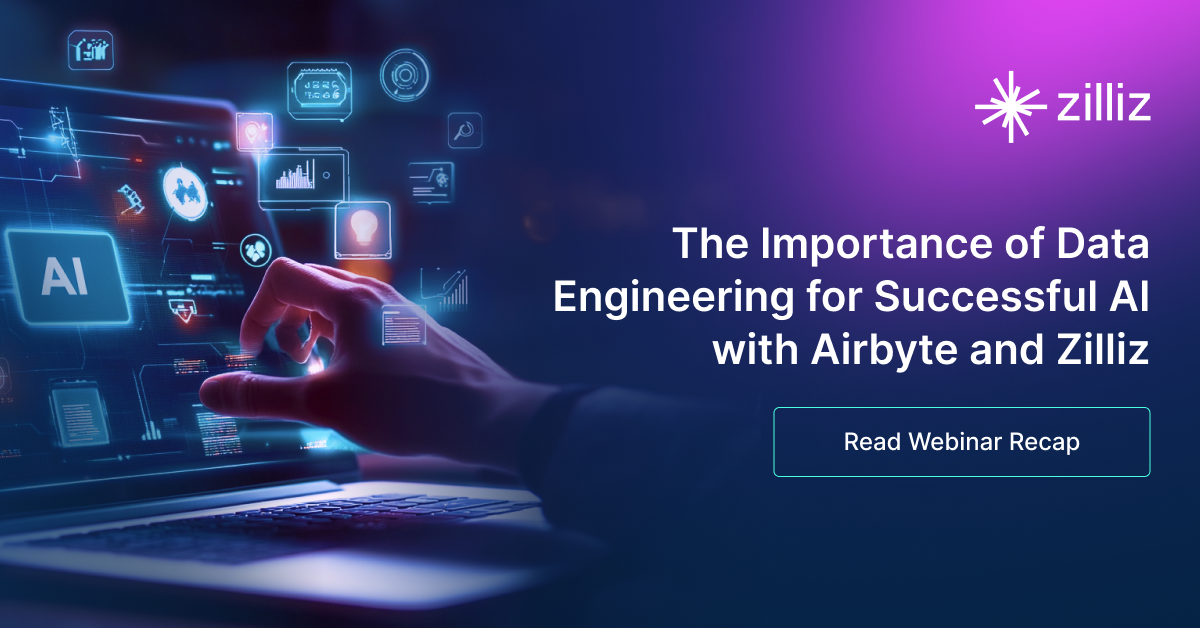
The Importance of Data Engineering for Successful AI with Airbyte and Zilliz
Learn how data engineering can resolve common challenges associated with deploying and scaling effective AI usage.
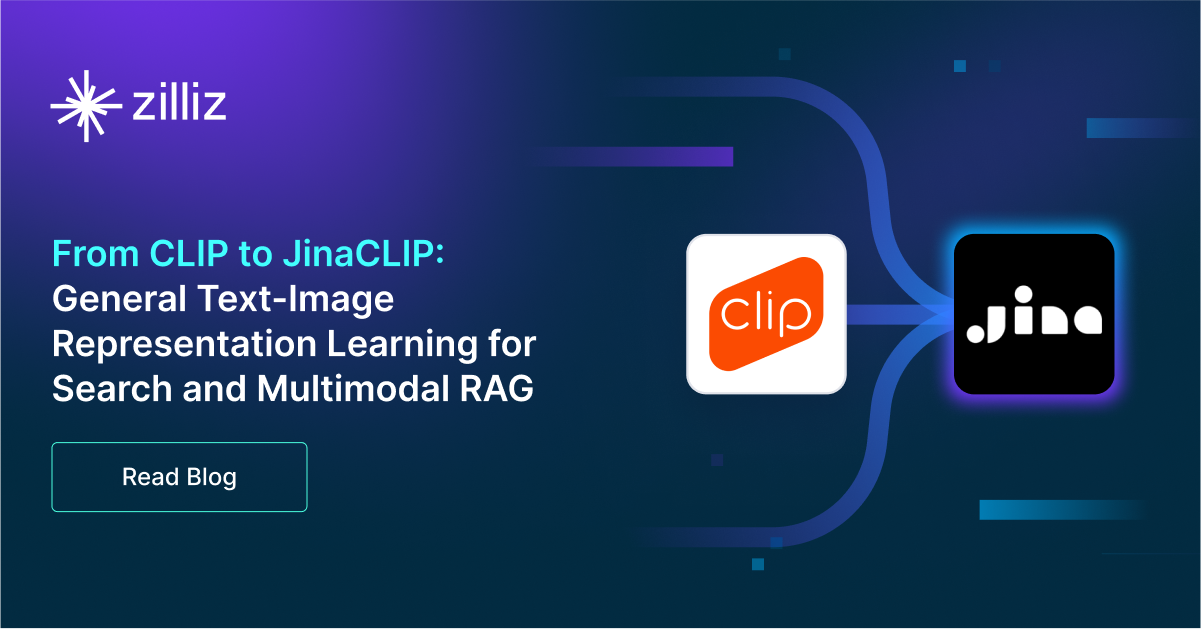
From CLIP to JinaCLIP: General Text-Image Representation Learning for Search and Multimodal RAG
In this blog, we will implement a multimodal similarity search system. This system will use JinaCLIP to generate multimodal embeddings and the Milvus vector database to store and retrieve similar embeddings given a certain query.