Introduction to MemGPT and Its Integration with Milvus
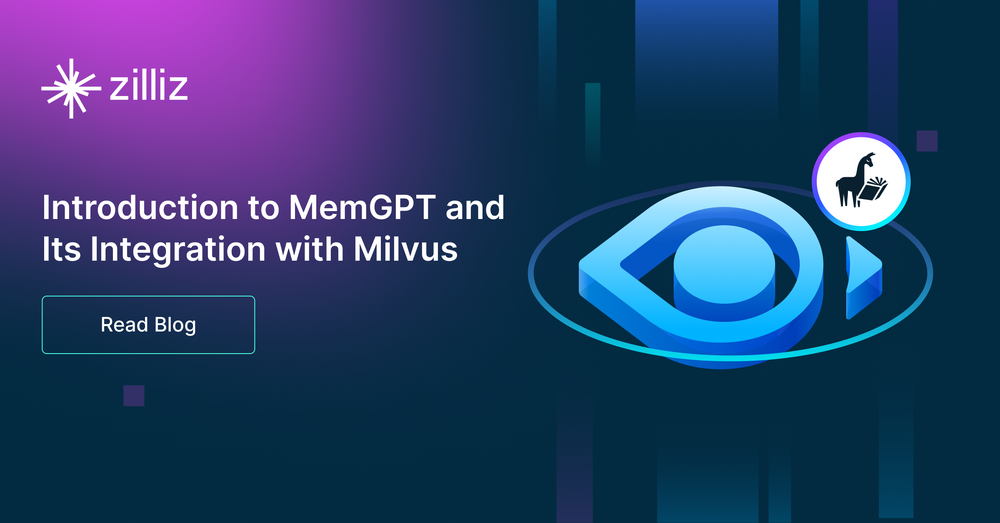
During our unstructured data meetup in May, we were joined by Charles Packer, a PhD student at UC Berkeley and Co-Founder of MemoryGPT (MemGPT). Charles highlighted a fundamental bottleneck in the current large language models (LLMs): limited memory, and explained how MemGPT aimed to solve this problem by taking inspiration from OS design.
This blog will cover the key concepts discussed in the talk and explain the ‘virtual extended memory’ introduced by MemGPT. However, if you wish to absorb the knowledge firsthand, you can revisit the entire talk on YouTube.
Limited Memory in Present LLMs
Charles opened his talk by introducing memory as context for an LLM and highlighting its importance: “For LLMs, memory is everything.” An LLM’s context window defines how much information it can retain during a conversation. This retention ability allows the language model to recall information from prior queries, making it a powerful tool for developing various chatbot applications.
However, the context window is quite limited for most mainstream LLMs. A few examples include 32k tokens for GPT4 and 128k for GPT4-Turbo. The limited memory means that these chatbot applications tend to forget older conversations after a certain number of queries, which can lead to a disappointing user experience. Charles highlighted this issue as a major bottleneck for LLM applications, further stating that LLMs are reduced to being search engines rather than assistants.
The relations between search engines, LLMs, and AI assistants
The closest solutions to the memory problem are retrieval augmented generation (RAG) and long-context LLMs like Gemini. But while these offer some improvement, RAG isn’t true memory, and long-context LLMs increase computational costs manifold. Moreover, the sub-par performance of long-context models like DevinAI proves that limited contexts are not the problem; instead, it is the context pollution caused by token mismanagement.
MemGPT: From Search Engines to Assistants
MemGPT introduces a virtually extended context window inspired by the memory pagination implemented in computer systems. In computers, key information is stored in memory (RAM) for faster access, but when the RAM fills up, the remaining data is stored in persistent storage (ROM). The operating system (OS) swaps information between the RAM and the ROM depending on what it needs to access.
MemGPT follows the same concept by creating an extended memory system. It divides the LLM context into two parts.
Main Context: This is the underlying LLM's context window. It has a limited bandwidth and stores the most relevant information that can be readily accessed.
External Context: The external context is created on persistent storage (ROM) and has an infinite window. It stores key ideas and information as the conversation continues and the main context fills up.
MemGPT takes charge of managing the information flow between the two contexts. It dynamically updates the memory based on the current context, efficiently swapping information between the main and the external memory stores. This control and efficiency are key to the system's performance.
This approach positions MemGPT as a crucial component in applications that demand long-context memory. For instance, personal assistant chatbots, which need to retain key user life details, can benefit significantly. Personal relationships and work details are stored in the external context and retrieved as the ongoing conversation necessitates. This approach offers users a truly 'personal' experience, enhancing the system's relevance and usefulness.
Structure of MemGPT
Charles explained the key differences between MemGPT’s architectural design and a standard LLM. First is the difference in context handling, which we have discussed in detail above. Second is the way it handles inputs and outputs. Its inputs and outputs are structured as JSON, treating them as events and function calls rather than plain text.
Standard LLM Setup
How MemGPT works
It can accept documents, user queries, and system alerts as input, triggering an event in the LLM. The event is parsed and transferred to the LLM, which utilizes the extended context to process the requirement and perform actions like a function call. This architectural design allows it to act like an agent trained to perform specific tasks. For example, when an email is received, it can trigger an event, and the LLM will parse the email to extract and highlight key details.
MemGPT as a Service
Charles concluded the sessions by explaining how MemGPT can be deployed on a private server for long-term use. Every interaction and query is maintained in a stateful database that can be accessed even after the system is closed.
Since the MemGPT agent lives on a server, it is accessed via the REST application programming interface (API). The agent can be accessed from anywhere via the internet, making it easy to integrate with commercial applications.
Integrating MemGPT with Milvus Vector Database
Milvus is an open-source vector database for billion-scale vector storage and retrieval. It is also one of the most important technologies for building retrieval augmented generation (RAG) applications.
Milvus has integrated with MemGPT, making it easier for developers to build AI agents with connections to external data sources.
In the following example, we'll use MemGPT to chat with a custom data source stored in Milvus.
Configuration
Install the required dependencies.
pip install 'pymemgpt[milvus]'
Configure Milvus connection via the following command:
memgpt configure
...
? Select storage backend for archival data: milvus
? Enter the Milvus connection URI (Default: ~/.memgpt/milvus.db): ~/.memgpt/milvus.db
You just set the URI to the local file path, e.g., ~/.memgpt/milvus.db
, which will automatically invoke the local Milvus service instance through Milvus Lite, a lightweight version of Milvus for quick prototyping.
Important Note: If you have a larger amount of data, such as more than a million documents, we recommend setting up a more performant Milvus server on Docker or Kubernetes. In such cases, your URI should be the server URI, e.g., <http://localhost:19530>
.
Creating an external data source
In this step, we must create a data source to feed external data into a MemGPT chatbot. We will use MemGPT’s research paper as an example data source.
To download this paper, we'll use the curl
command. You can also just download the PDF from your browser.
curl -L -o memgpt_research_paper.pdf https://arxiv.org/pdf/2310.08560.pdf
Now, we have downloaded the paper. Then we must create a MemGPT data source using memgpt load
:
memgpt load directory --name memgpt_research_paper --input-files=memgpt_research_paper.pdf
Loading files: 100%|███████████████████████████████████| 1/1 [00:00<00:00, 3.94file/s]
Loaded 74 passages and 13 documents from memgpt_research_paper
Attaching the data source to a MemGPT agent
Now, we’ve created our data source. We can attach it to a MemGPT chabot at any time.
Let's create a new chatbot using the memgpt_doc
persona (you can use any persona you want):
memgpt run --persona memgpt_doc
When chatting with the agent, we can "attach" the data source to the agent's archival memory:
? Would you like to select an existing agent? No
🧬 Creating new agent...
-> 🤖 Using persona profile: 'sam_pov'
-> 🧑 Using human profile: 'basic'
🎉 Created new agent 'PoliteButterfly' (id=d26e1981-ff36-4095-97a0-61a1601dfb5d)
Hit enter to begin (will request first MemGPT message)
💭 Interesting, I've got a first-time user. Time to present myself and get to understand the user's needs. I wonder what brings Chad here today.
🤖 Greetings Chad! I'm MemGPT. How may I assist you today?
> Enter your message: /attach
? Select data source memgpt_research_paper
100%|███████████████████████████████████| 1/1 [00:00<00:00, 4.81it/s]
Testing out our new chatbot
We have loaded the data into the chatbot's memory. Now, we can start to ask questions.
> Enter your message: The paper I loaded into your archival memory is called MemGPT. Can you tell me more about it?
💭 It seems Chad would like to know more about the paper titled 'MemGPT' that's stored in my archival memory. It's time to search the archival memory for details and background data to provide a comprehensive
understanding of the paper. Let's remember to page through results until I've gathered enough information to answer Chad's enquiry.
⚡🧠 [function] searching memory with archival_memory_search
💭 Based on the found results, MemGPT is an AI system designed for tasks like extended conversations and document analysis. It efficiently manages different tiers of storage to provide extended context within
the LLM’s limited cap. Also, noteworthy from my findings is that MemGPT performs impressively in deep memory retrieval (DMR) and document QA tasks. Let's prepare a comprehensive response for Chad.
🤖 Certainly, Chad. MemGPT, or MemoryGPT, is a system specially designed for tasks like extended conversations and document analysis which are traditionally hindered by the limited context windows of modern
Large Language Models (LLMs). It uses a technique inspired by hierarchical memory systems in traditional operating systems, called virtual context management. This technique allows for an effective provision
of extended context within an LLM’s limited context window by intelligently managing different storage tiers, much like paging between physical memory and disk. Notably, MemGPT outperforms the fixed-context
baselines in deep memory retrieval and it's able to analyze large documents with precision.
Final Thoughts
The brief talk from Charles Packer was a great dive into the concepts of MemGPT. The session discussed traditional LLMs' memory and context limitations and presented them as bottlenecks for LLM applications.
MemGPT introduces the concept of extended virtual memory by creating an external context stored on disk storage. The concept is inspired by how computer OS manages memory by swapping information between RAM and ROM. The MemGPT agent can store vital information on an external database and access the information based on the present context. The agent opens up new avenues for long-context application development.
Integrating the Milvus vector database and MemGPT has taken one step further in streamlining the development of AI Agents with connections to external data sources. In this post, we also shared an example demonstrating how to use this integration to build a chatbot with external memories.
- Limited Memory in Present LLMs
- MemGPT: From Search Engines to Assistants
- Structure of MemGPT
- MemGPT as a Service
- Integrating MemGPT with Milvus Vector Database
- Final Thoughts
Content
Start Free, Scale Easily
Try the fully-managed vector database built for your GenAI applications.
Try Zilliz Cloud for FreeKeep Reading
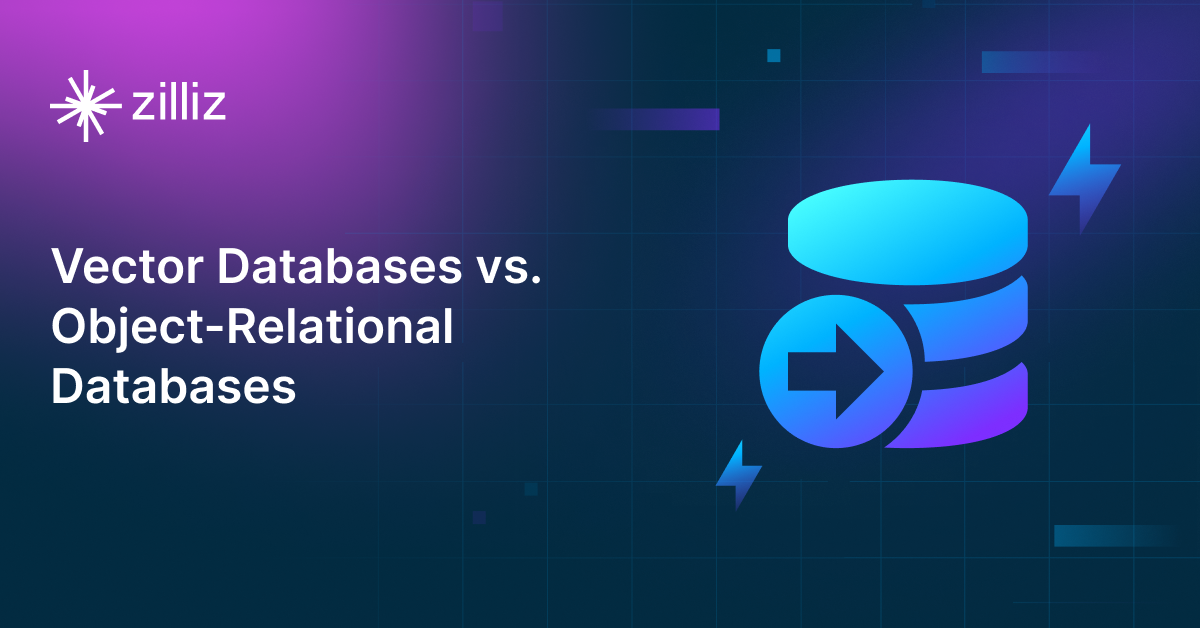
Vector Databases vs. Object-Relational Databases
Use a vector database for AI-powered similarity search; use an object-relational database for complex data modeling with both relational integrity and object-oriented features.
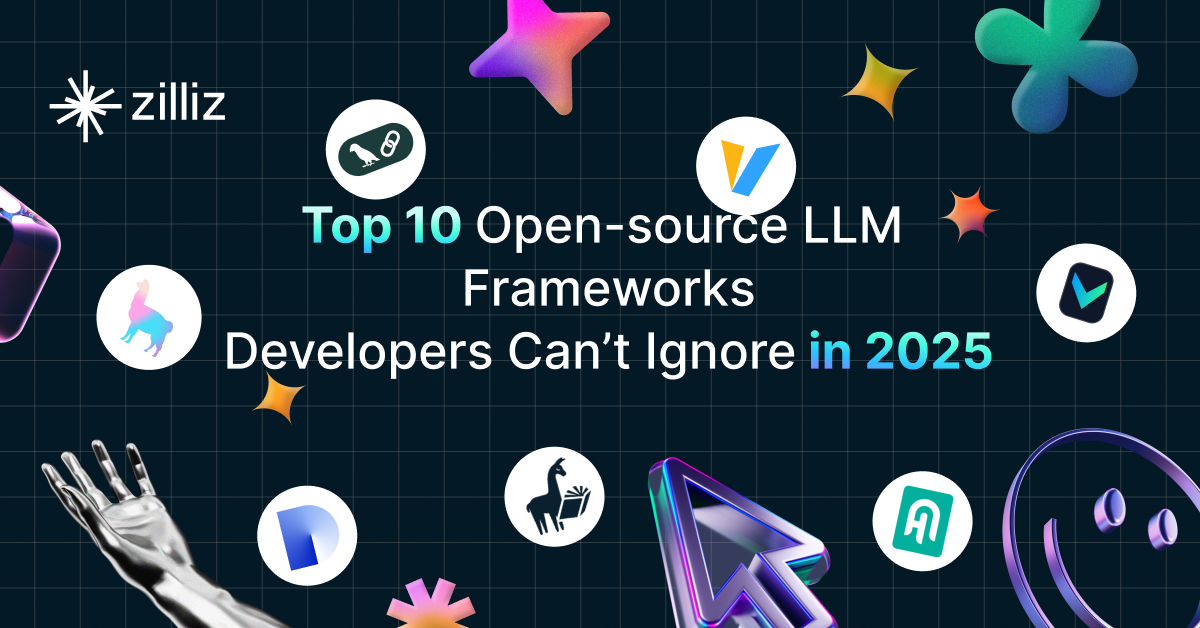
10 Open-Source LLM Frameworks Developers Can’t Ignore in 2025
LLM frameworks simplify workflows, enhance performance, and integrate seamlessly with existing systems, helping developers unlock the full potential of LLMs with less effort.
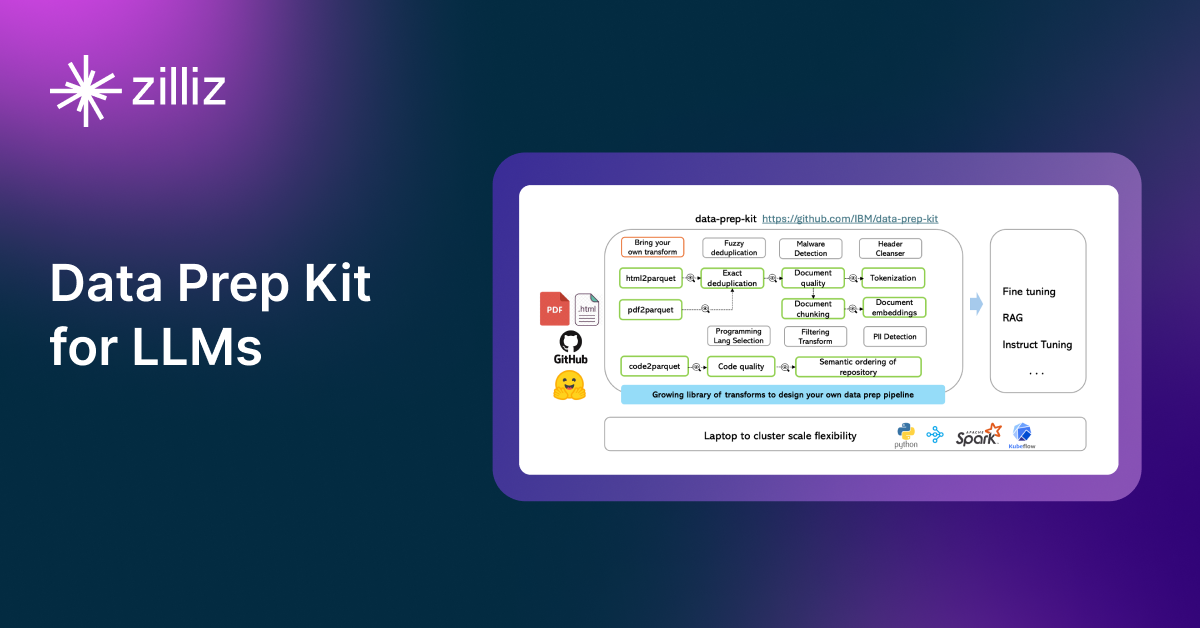
Introducing IBM Data Prep Kit for Streamlined LLM Workflows
The Data Prep Kit (DPK) is an open-source toolkit by IBM Research designed to streamline unstructured data preparation for building AI applications.