How Sohu Enhances Personalized News Recommendation with Milvus
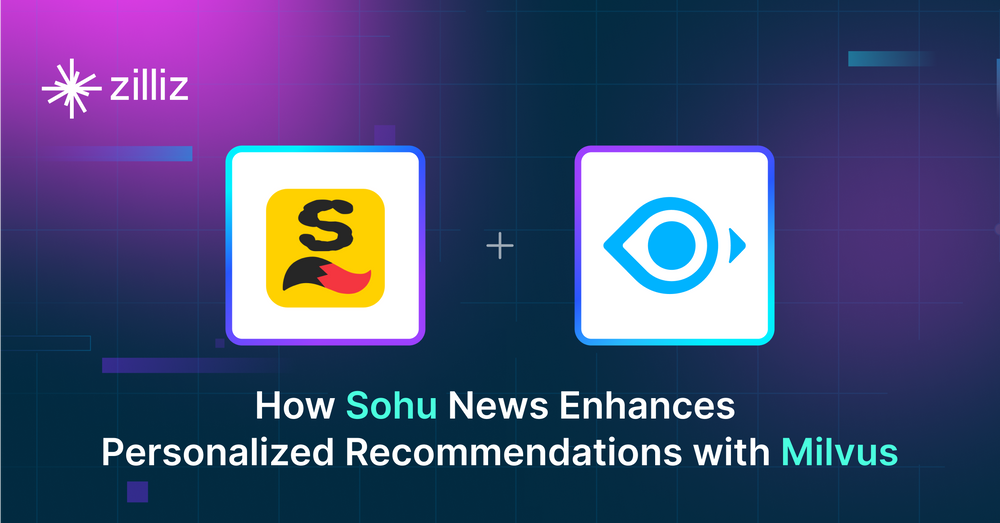
In the fast-paced world of Internet services, staying ahead of user expectations is crucial. Sohu, a NASDAQ-listed company, recognized this need and reformed its news recommendation system through a strategic collaboration with Milvus, an open-source vector database. This blog explores the challenges faced by Sohu News (a primary arm of Sohu), the innovative solutions implemented, and the transformative impact Milvus had on Sohu News’ recommendation system.
Sohu’s News Delivery Headaches
Despite acknowledging the importance of staying ahead, Sohu News found itself constrained by an outdated and inefficient legacy vector search stack in its recommender system. This outdated stack impeded rapid vector retrieval and struggled to scale seamlessly with the expanding volume of news data, resulting in a failure to deliver real-time, personalized news to users. Complicating matters further was the classification of short-text news articles, known for their limited information. The existing system grappled with accurately categorizing these concise snippets, leading to frequent misclassifications.
Recognizing the need for a robust solution to handle large datasets, provide accurate recommendations, and enhance the classification of short-text news, the Sohu News team started finding an innovative approach to position them as news delivery leaders.
The Milvus Vector Database Comes to the Rescue
Milvus, known for its lightning-fast performance and high recall rate, proved to be the ideal solution for handling massive amounts of unstructured data. With support for various indices, including FLAT, HNSW, and ScaNN, Milvus offered the flexibility to balance accuracy, performance, and cost. After careful evaluation, the Sohu News team chose Milvus to build the vector search engine for its recommendation system.
Milvus Integration with Sohu’s News Recommendation System
Sohu News smoothly incorporates Milvus into its recommender system and employs a dual-tower structure within the Milvus-powered vector search engine. Each tower represents the semantic vectors of users' preferences and news articles.
News articles were transformed into vectors using the BERT-as-service model and stored in the Milvus vector database. Simultaneously, user profiles, comprising labeled tags and keywords from browsing history, search queries, and interests, were also converted into vectors. Then, Milvus calculates the cosine similarity between user and article vectors and generates Top-K results in a recommendation pool, prioritizing and delivering articles based on estimated click-through rates (CTR).
Resolving Short News Misclassifications with Milvus
Short-text news articles contain limited information, so the system pre-classifies them before conducting vector semantic searches. Milvus is crucial in identifying and rectifying misclassified short news, enhancing classification accuracy. The process involves converting long and brief news articles into vectors using the BERT-as-service embedding model, storing them in Milvus, and calculating the cosine similarity between the two types of vectors. Next, Milvus returns the top 20 long news articles with the highest cosine similarity.
The subsequent analysis examines the categories of these 20 long news articles most semantically similar to the queried short news. Suppose more than 18 of these articles share a consistent category but deviate from the category assigned to the queried short news. In that case, it signals a potential error in the brief news category classification. The team promptly corrects these errors, resulting in a classification accuracy rate exceeding 95% and highlighting the effectiveness of Milvus in this process.
Transformative Impact on Sohu News Recommendation System
Sohu's collaboration with Milvus yielded impressive results. The recommendation system achieved a 10x faster vector retrieval speed and significantly improved recommendation accuracy. Milvus's support for mainstream indices and efficient memory consumption aligned seamlessly with Sohu's operational needs, ensuring a more personalized and engaging user experience.
Conclusion
The collaboration between Sohu and Milvus is a testament to the transformative power of advanced vector search technology. By addressing the challenges of vector retrieval speed, recommendation accuracy, and short-text news classification, Milvus has propelled Sohu News into a new era of innovation, offering users a more personalized and engaging news experience.
Start Free, Scale Easily
Try the fully-managed vector database built for your GenAI applications.
Try Zilliz Cloud for Free