Demystify Benchmark Result Divergence: Milvus vs. Qdrant
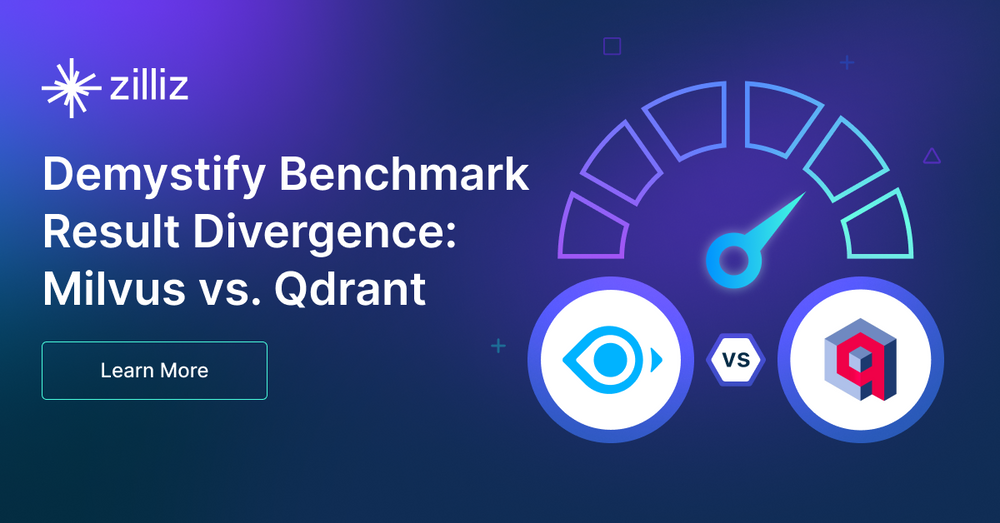
With the rising popularity of GenAI, an increasing number of vector databases have entered the market. Early last year, we introduced VectorDB Bench to provide insights into the performance of emerging vector database technologies.
Recently, developers deeply invested in this field approached us at Zilliz, seeking to understand the substantial disparities between Qdrant's benchmark results and our findings in VectorDB Bench. In particular, they needed clarification on Milvus' pool performance in the Qdrant benchmark results. In response to these inquiries, our engineering team at Zilliz initiated a comprehensive investigation to uncover the root causes of these disparities.
This blog post provides an in-depth technical analysis of the benchmark differences, with specific attention to the performance discrepancy of Milvus. Without further delay, let's delve into the details of this investigation.
Reason #1: outdated Milvus version
The disparities in the benchmark results primarily stem from the different Milvus versions used in testing. The Qdrant benchmark report, based on Milvus v2.1 and published on August 10, 2022, doesn’t fully capture the significant advancements made in later versions. Check out the raw data from this report. Since then, Milvus has undergone considerable enhancements.
In Milvus v2.2.1, we upgraded the vector engine (also known as Knowhere) and revised the parallelism strategy. Subsequent updates in v2.2.3 brought further improvements in search performance. Our white paper highlights these advancements, demonstrating that Milvus 2.2.3 is four times faster than Milvus 2.0 in query performance (latency and throughput) and scalability (billion-scale collections and multiple replicas).
Following this, v2.2.9 improved filtered search performance. In v2.2.12, we introduced better search efficiency with minimal overhead for large top-K values, enhanced write performance in partition-key-enabled or multi-partition scenarios, and optimized CPU usage for larger machines. Additionally, v2.3.2 marked a significant performance enhancement with minimized data copying during loading and better bulk insertions.
These continuous enhancements have substantially transformed Milvus' capabilities. As a result, benchmarks based on Milvus 2.1 no longer accurately reflect the technology's current performance. To better understand the latest capabilities of Milvus, developers are encouraged to refer to the VectorDB Bench, which employs Milvus 2.3 for testing.
Reason #2: improper use of Milvus
Qdrant's benchmark on Milvus performance partly results from how it only used Growing Segments. As the name suggests, Milvus optimizes with two segment types: Growing and Sealed Segments. Growing Segments are still receiving data until it reaches a predefined threshold. These segments prioritize fast data input and utilize a brute-force search strategy, which leads to slower query performance.
On the other hand, Sealed Segments no longer receive data and, therefore, have an index, resulting in significant performance gains. Milvus automatically seals Growing Segments when it reaches a predefined threshold, thus allowing this data also to see the performance gains when used with an index.
The Qdrant benchmark only focused on using Growing Segments, which naturally led to the reported slower performance. Concentrating only on Growing Segments differs from how Milvus is used in real-world applications and defeats the purpose of the segment strategy contained in Milvus.
In addition, to help users balance data freshness and search efficiency, Milvus v2.3 introduced support for the IVF-FLAT index for growing indexes. Additionally, Milvus v2.3.4 introduced the Binlog index for Growing Segments. This update enables the use of advanced indices such as IVF or Fast Scan in these segments, potentially enhancing search performance by up to tenfold. As a result, this feature improvement has made the previous Qdrant benchmark results even less relevant.
Reason #3: benchmark-driven optimization for Qdrant
Qdrant's exceptional benchmark performance against other vendors stems from its use of super-large segments for benchmarking. While this strategy delivered noteworthy results in the benchmarks, its real-world applicability is in question. In vector databases, the size of segments is crucial. Qdrant's focus on large segments boosted benchmark scores but might compromise operational flexibility in everyday use.
Effective vector databases must handle diverse workloads and evolving data requirements. Super-large segments, while effective in benchmarks, may struggle with the varied queries typical in real-world situations. They introduce complexity in management and could escalate resource demands.
While Qdrant's benchmark-driven optimizations, like super-large segments, showcase impressive performance, their practicality in dynamic, real-world environments raises concerns. This questions the overall relevance of such benchmark results in actual vector database deployments.
Closing thoughts: a path to fair and informed decisions
When it comes to vector databases, trustworthy and comprehensive benchmarking is vital. VectorDB Bench, built by Zilliz, contributes real-world performance data for developers. Acknowledging the challenge of achieving absolute impartiality in benchmarks, we share our insights to enrich the collective knowledge in this domain.
The ANN Benchmark is a valuable tool for those searching for impartial assessments, providing standardized evaluations of vector databases. As technology advances in this field, we stress the need for clear, transparent, and collaborative benchmarking efforts. Developers must access truthful and precise benchmarks or conduct their own tests against their data to make informed decisions when choosing a vector database.
- Reason #1: outdated Milvus version
- Reason #2: improper use of Milvus
- Reason #3: benchmark-driven optimization for Qdrant
- Closing thoughts: a path to fair and informed decisions
Content
Start Free, Scale Easily
Try the fully-managed vector database built for your GenAI applications.
Try Zilliz Cloud for Free