A moving average is a technique used to smooth time series data by calculating the average of observations over a defined window. This method helps to reduce noise and highlights underlying trends. For example, a 5-day moving average for sales data computes the average sales of the last 5 days at each point in the series. There are different types of moving averages, such as the simple moving average (SMA) and weighted moving average (WMA). SMA assigns equal weight to all observations within the window, while WMA gives more weight to recent observations, making it more responsive to changes. These techniques are used to understand trends without being distracted by short-term fluctuations. In time series modeling, the moving average concept forms the basis of the MA component in ARIMA models. Unlike descriptive moving averages, this component adjusts predictions by incorporating past forecasting errors. For example, an MA(1) model corrects the forecast using the error from the previous time step, making it an essential tool for dynamic prediction.
What is a moving average in time series?
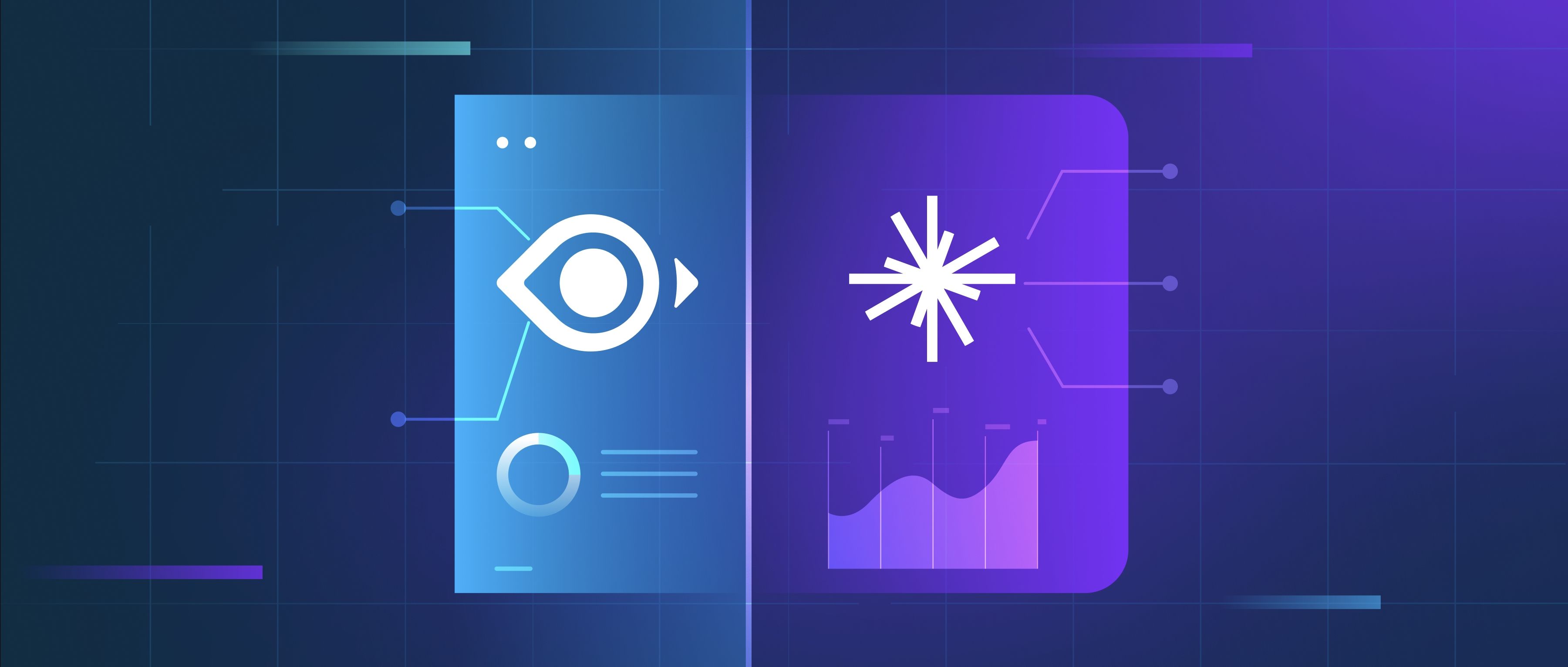
- Natural Language Processing (NLP) Basics
- The Definitive Guide to Building RAG Apps with LlamaIndex
- Getting Started with Milvus
- Retrieval Augmented Generation (RAG) 101
- Getting Started with Zilliz Cloud
- All learn series →
Recommended AI Learn Series
VectorDB for GenAI Apps
Zilliz Cloud is a managed vector database perfect for building GenAI applications.
Try Zilliz Cloud for FreeKeep Reading
What are the latest trends in self-supervised learning research?
Self-supervised learning (SSL) has seen significant advancements recently, aimed at improving model performance without
How does image preprocessing affect search results?
Image preprocessing plays a crucial role in determining the effectiveness of search results when leveraging images withi
Can anomaly detection identify rare events?
Yes, anomaly detection can identify rare events. Anomaly detection is a technique used to identify data points that sign