Shopee Revolutionizes Its Multimedia Business with Milvus
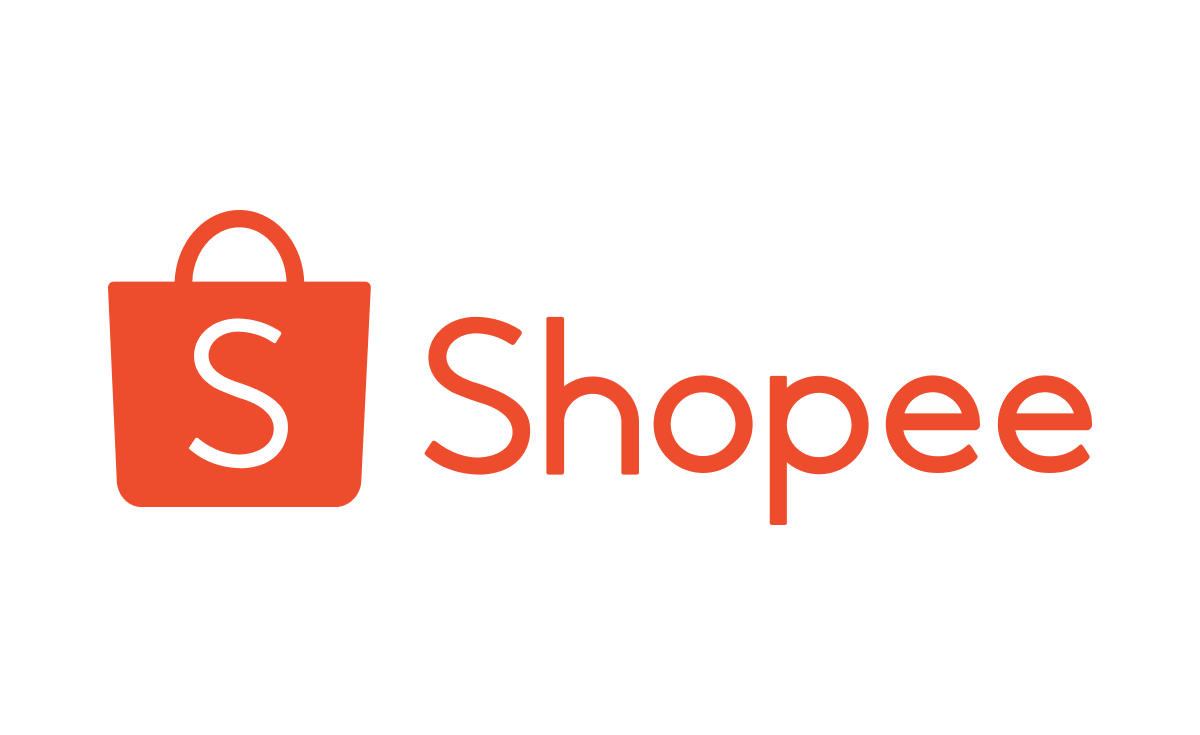
> 100M
embedding vector storage and searching
Seamlessly integration
with various internal systems and tech stacks
Enhanced real-time data retrieval
with reduced latency and increased system availability
Milvus has dramatically facilitated the MMU team in building various business systems and effectively supports our rapid business growth. Thanks to the Milvus team for developing such a fantastic vector database with stable vector search capabilities and rich functionalities.
The MMU team
About Shopee
Shopee is a leading e-commerce platform in Southeast Asia and Latin America, bridging the gap between buyers and sellers across diverse products. With its user-friendly interface, secure payment options, and extensive product range, Shopee provides millions of regional users with a seamless online shopping experience, making it their top choice.
Shopee has launched its Multimedia Understanding (MMU) business to compete with short video giants like TikTok and prevent them from biting into its e-commerce market share. As part of the MMU business, Shopee has rolled out its short video services, including a TikTok-like feature called Shopee video and a short video application.
The Challenge: Lack of A Robust Vector Search Engine for Vast Volumes of Unstructured Data
In Shopee's burgeoning multimedia venture, the influx of vast amounts of unstructured data, comprising videos, images, audio, and text, posed a significant challenge and proved daunting for traditional databases. To effectively derive actionable insights from this data, Shopee's team employed embedding tools to transform unstructured data into embedding vectors, but still urgently needed a robust vector database system for storing those vectors and swiftly searching through them.
Shopee's various internal systems, including video recall systems, video deduplication systems, and video recommendations, further complicated the scenario. These systems were meticulously crafted to manage and enhance Shopee's multimedia business. These internal systems were built with different technologies and relied heavily on vector search capabilities. Therefore, Shopee required a robust vector search engine that seamlessly fits into these systems and various technological stacks.
The Solution: Building a Vector Search Engine Using Milvus
The MMU team rigorously explored various open-source vector search engines for a robust solution. After extensive research, Milvus emerged as the perfect fit. Milvus can handle billions of vectors and quickly scale out as data volume rises. Milvus' cloud-native architecture seamlessly integrated with Shopee's internal ecosystem, enabling the rapid setup of vector retrieval systems from scratch. Its feature-rich offerings, including distributed processing, GPU support, incremental updates, and scalar support, comprehensively addressed Shopee's multifaceted requirements. After careful consideration, the team selected Milvus as the foundation for their vector search engine to construct their vector search systems from scratch.
A Search Engine Built with Milvus 1.x: Efficient But With High Latency As Data Scales
Shopee’s MUU team initially implemented Milvus 1.x, employing a distributed solution using Milvus 1.1 and Mishards. This efficient solution could address Shopee's pain points of storing and searching through vast amounts of vectors. However, challenges arose as Shopee's business grew with rapidly increasing data and requests. Mishards' default sharding strategy occasionally led to uneven segment distribution among read-only nodes, causing latency. The solution came through deploying multiple sets of Mishards clusters, sharing databases, and S3 buckets.
Milvus 2.x: A Game-Changer Bringing Enhanced Scalability And Reduced Latency
While the search engine built with Milvus 1.x was effective, this approach incurred significant deployment and maintenance costs, prompting the team to explore more efficient deployment methods.
With the introduction of Milvus 2.x, Shopee's systems underwent a transformative shift. Milvus 2.x’s enhanced stability, scalability, and multi-replica capability proved revolutionary. These improvements bolstered real-time retrieval services, ensuring low latency and high availability. Milvus 2.x's cloud-native architecture introduced low-cost logging and monitoring features, ushering in an era of user-friendly and more efficient solutions for Shopee.
Milvus 2.x architecture
Milvus Empowering Various Business Systems
Shopee's real-time search capabilities have reached new heights with the integration of Milvus. The video recall system is a prime example of this improvement. Milvus has seamlessly incorporated instant video recall into Shopee's video recommendation systems, which has enhanced the user experience for millions of people globally. Milvus has also made offline data retrieval, which is crucial for copyright video matching and video deduplication, much more efficient. Milvus is instrumental in recognizing original content and identifying duplicate videos, ensuring the content remains fresh and original while enhancing user satisfaction.
Video Recall System: Improving Video Recommendation
Shopee's video recall system uses Milvus as a cornerstone in the process of recommending videos. When a user searches for a video, the business requests access to Milvus to retrieve the most similar Top-K candidates. These results undergo refinement through post-ranking algorithms before being returned to the user.
Video Recall System Architecture Using Milvus 1.x
Initially, Shopee used Milvus 1.x versions to build the video recall system. However, as the system scaled, it faced latency challenges. To address this issue, Shopee introduced a caching mechanism to store Top-K and backend updates. Upgrading to Milvus 2.x has simplified the system's architecture and operations, enabling direct Top-K recall capabilities through Milvus' robust distributed interfaces and enhancing system performance.
Video Recall System Architecture Using Milvus 2.x
Copyright Match System: Better User Experience and System Integrity
Shopee's short video services have become increasingly popular, resulting in a large number of videos being created and uploaded to its platform. To maintain an excellent user experience and protect the copyrights of video creators, Shopee has implemented a copyright match system using Milvus. All released video features are transformed into vectors and stored in Milvus, and every newly uploaded video is matched with those held in Milvus by using similarity searches.
Copyright Match System Architecture
The method comprises four essential modules: pre-processing, feature extraction, results sorting, and rescan. These modules work together to accurately identify duplicate or stolen content, ensuring the integrity and reliability of the system.
Video Deduplication System: Enhancing User Value
The video deduplication system is designed to eliminate redundant content from Shopee's video platform. Like Shopee's copyright match system, the deduplication system uses Milvus to store embedding vectors transformed from video features. The system efficiently identifies and eliminates duplicate videos by searching for Top-K results in Milvus that are most similar to a specific part. Apart from the Top-K similarity search, the system involves other processing techniques such as batch data searching, post-ranking, clustering, and fingerprint assignment. In the end, Milvus stores all these results, providing valuable insights to various business units.
Video Deduplication System Architecture
The Road Ahead
Shopee's collaboration with Milvus is a testament to innovation's power in shaping the future of e-commerce. Milvus empowered Shopee's multimedia business, equipping them with the tools necessary to unravel the complexities of multimedia understanding. Looking ahead, Shopee envisions Milvus evolving to meet increasingly sophisticated AI demands. With Milvus as a steadfast partner, Shopee anticipates a future where multimedia understanding seamlessly integrates with user experience, paving new paths in e-commerce.
This post is written by the Shopee MMU team and is edited and posted here with permission.
- About Shopee
- The Challenge: Lack of A Robust Vector Search Engine for Vast Volumes of Unstructured Data
- The Solution: Building a Vector Search Engine Using Milvus
- A Search Engine Built with Milvus 1.x: Efficient But With High Latency As Data Scales
- Milvus 2.x: A Game-Changer Bringing Enhanced Scalability And Reduced Latency
- Milvus Empowering Various Business Systems
- The Road Ahead
Content
Industry
E-commerce