Understanding Digital Twins
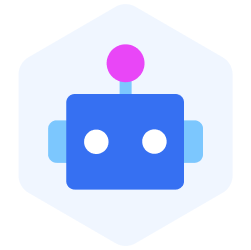
Understanding Digital Twins
What if you could replicate an entire system in a digital environment and run multiple simulations to see how it responds to varying conditions?
This approach would allow businesses to understand how their operations would change due to sudden economic shocks, developers to know how an application would behave due to an upsurge in demand, and engineers to see the impact of tweaking a system’s specifications and configurations. These are just a few possibilities that can occur through digital twins - a virtual replica of any process or system in a digital environment.
This page will explain digital twins, how they work, their benefits and challenges, and a few use cases to help you understand how you can use digital twins to optimize your operations.
What is a Digital Twin?
A digital twin is a virtual model representing a physical system or process that helps users understand its behavior in various situations. The model gets real-time data from multiple sources associated with the system’s physical environment.
Figure- Digital Twin Aviation .png
Figure: Digital Twin Aviation
Machine learning (ML) and artificial intelligence (AI) algorithms analyze the data to predict a system’s future states and help users identify areas for improvement. Detailed visualizations are critical to simulating digital twins to evaluate a system’s behavior in different environmental conditions.
How Does Digital Twin Work?
Digital twins work by connecting a virtual environment with a physical counterpart using real-time data. The following list highlights the critical components of a practical digital twin to help you understand how the technology works.
Figure- Components of a Digital Twin.png
Figure: Components of a Digital Twin
Physical System: A digital twin maps a real-world object or process in a digital environment. For instance, a digital twin of a manufacturing plant can represent a virtual model of the entire factor floor with all the workflows associated with product assembly.
Data Collection: Sensors and Internet-of-Things (IoT) devices placed near the system capture relevant data regarding the system’s behavior. For instance, the sensor can study the time it takes for a component to move from a storage facility to the assembly line.
Data Analysis and Visualization: AI and ML algorithms can help analyze the data to reveal hidden patterns and highlight problems. Interactive dashboards with multiple controls to filter data according to specific criteria can further enhance the analysis. For example, algorithms can analyze sensor data collected from a manufacturing plant to understand how the manufacturing system works. Managers can filter specific analyses based on operational hours, maintenance history, and machine type to delve deeper into particular aspects of the workflow and identify improvement areas.
Simulation: Users can tweak the system’s configuration within the virtual environment to understand the impact. For instance, a manufacturer can study the effect of removing a specific object from the factory floor on the time it takes for a component to reach the assembly line.
Types of Digital Twins
As digitization dominates every field of business, new types of digital twins are emerging. The list below mentions a few popular types of digital twins in industrial use cases.
Figure- Types of Digital Twins.png
Figure: Types of Digital Twins
Product Twin: A product twin is a virtual copy of a product or object. Depending on its complexity and magnification level, a product twin can represent a concept design, a specific component, a group of parts, or an entire product. For instance, a product twin may represent an electric vehicle model and allow engineers to study how the vehicle’s speed changes according to different conditions such as battery type, fuel quality, weather, etc.
System Twin: A system twin is a digital model of two or more physical objects or assets interacting with one another. The representation helps you understand how the objects depend on each other and highlights potential improvements to optimize their interactions. An example of a system twin can be a robotic arm assembling a product on a conveyor belt. Experts can replicate the system in a virtual environment to study the impact of varying workloads on the arm’s performance.
Process Twin: A process twin represents how a group of systems work together to achieve a particular objective. For instance, a digital twin of a manufacturing plant consists of multiple systems that assemble a product.
Digital Twin vs Simulation
Understanding the difference between a simulation and a digital twin is often confusing. Although the two may appear identical, they have a few significant differences.
Simulation replicates a specific system to study certain behaviors in a static environment. It helps a user understand the impact of pre-defined scenarios on a system’s performance. The process includes changing specific parameters within a particular time frame and analyzing the changes.
The digital twin is more dynamic and synchronized with the real world, constantly updating a system’s state in real-time. This means a user can test how a system will behave more deeply by changing conditions in the virtual environment and predict future behavior.
The list below summarizes the differences between the two concepts based on a few factors:
Data and Real-time Integration: Simulations generate results using historical or hypothetical data, while a digital twin uses real-time data from sensors and IoT devices to constantly update a system’s state.
Feedback Loop: Simulations are one-way processes in which a model gives results based on specific parameter values. If the results reveal any issues, the user must manually change the system’s configurations. In contrast, a digital twin can send live commands to a physical system and adjust its behavior automatically.
Flexibility: Simulations are more rigid as they only model specific scenarios. A digital twin, however, is more versatile as it can adapt to changing conditions, offering more insights into a system’s operations.
Benefits and Challenges of a Digital Twin
With a greater need for certainty in a changing industrial ecosystem, digital twins significantly benefit businesses operating in dynamic environments. However, the technology is still evolving and has a few challenges.
The following sections explain these benefits and challenges to help you implement digital twins more efficiently.
Benefits
Better Product Development: Digital twins can help research and development teams design better products. They can use real-time data and simulation with AI-based algorithms to gain in-depth insights into a product’s performance.
Cost Optimization: System twins allow businesses to identify areas for improvement by studying the system’s behavior in multiple scenarios. The approach lets them reduce a system’s operational costs, increase its throughput, and make it more resilient to sudden external shocks.
Predictive Maintenance: Integrating digital twins with AI technology can help businesses identify failure points before they occur. Intelligent algorithms can use real-time data to predict potential issues and generate alerts to notify relevant team members.
Challenges
Change Management: Digital twins require teams to move beyond research and development and work with senior management to quickly implement changes necessary to improve a system, product, or process. Organizations can use a phased approach, where initially, a digital twin mirrors a part of the physical world at a smaller scale. Once the value of a digital twin becomes clear, the development team can involve senior management to expand the technology.
Data Management: Digital twins collect extensive data about a system, and managing such large data volumes is challenging. Developers must implement robust integration and pre-processing pipelines to ingest and clean real-time data for analysis. They can also use cloud platforms to store data in warehouses or lakes with efficient governance protocols to maintain data integrity.
Computing Power: Mimicking an entire system or process in a virtual environment requires extensive processing power. The problem gets more severe as a digital twin processes data in real time to constantly update its state. Addressing the issue requires companies to upgrade their IT infrastructure considerably with the latest hardware, which includes powerful GPUs, edge devices, and a distributed architecture for parallel processing.
Use Cases of Digital Twins
As highlighted earlier, digital twins can deliver significant value in multiple industrial use cases by offering organizations more visibility into their products, systems, and processes. Although the technology has diverse applications, the sections below highlight three areas where it is driving impact.
Manufacturing
Digital twins can help manufacturers optimize their products and processes more efficiently through monitoring, simulation, and remote control. The list below highlights a few areas where digital twins can deliver results.
Product Design: Manufacturers can create digital replicas of concept designs and implement different changes to develop a robust prototype. The virtual environment provides a cost-effective way to run experiments without increasing production costs.
Process Design: With a process twin, manufacturers can monitor their systems' performance under different conditions without changing the physical environment.
Supply Chain Management: Digital twins for supply chain workflows can help manufacturers monitor their fleets more effectively and identify optimal routes for faster delivery.
Agriculture
Digital twins can help the agriculture industry produce high-quality food in more significant quantities by removing uncertainties associated with weather conditions, supply and demand, and resource constraints.
Weather Modeling: A digital twin of a farm can allow farmers to understand how yields will change according to different weather conditions. This can empower farmers to better estimate the presence and impact of pollution, humidity, and temperature.
Forecasting: Farming is a dynamic field where soil conditions, climate, and seasonality can affect the quality and quantity of produce. A digital twin can mitigate the uncertainties associated with these factors and allow farmers to implement robust controls to make their farms resilient to natural shocks.
Resource Optimization: Optimal resource allocation to specific tasks such as seeding, irrigation, and harvesting is necessary to ensure food security. A digital twin can analyze and predict the resources required in particular areas for better resource management.
Healthcare
The healthcare industry is increasingly adopting digital twin technology to develop drugs, improve patient care, and conduct better diagnoses.
\
Drug Development: Creating effective drugs requires extensive clinical trials and analyzing complex data. A digital twin of a human or a particular organ can help scientists try the drug in multiple scenarios and study the impact more efficiently.
Better Patient Care: Digital twins can help create models of patients using their characteristics and understand how the replica will respond to different treatments. A doctor can quickly track the virtual patient's vitals and design effective therapies.
Advanced Diagnosis: Researchers can create digital replicas of a patient’s single cell or genome to understand the root cause of a particular disease. The process will help them design better surgical procedures to cure complex diseases.
FAQs of Digital Twins
- How do digital twins work in real-time?
Digital twins use IoT sensors to collect real-time data and analyze it using AI and ML algorithms to update a system’s state and predict future behaviors.
- What industries benefit most from digital twins?
Manufacturing, healthcare, and agriculture are a few industries that significantly benefit from digital twin technology.
- What are the costs associated with implementing a digital twin?
Initial costs include implementing data processing and integration pipelines, upgrading IT infrastructure to ensure high computing power, and investing in sensors and edge devices to collect real-time data.
- How does Digital Twin technology improve product design?
Digital twins allow engineers to develop virtual prototypes and test design changes to study the impact on the product’s performance. This reduces development costs and helps engineers collect extensive data for further analysis.
- Can Digital Twins help with predictive maintenance?
Yes, Digital Twins can constantly monitor a system’s health and generate alerts in case of potential issues. AI algorithms can predict failures before they occur, allowing teams to resolve problems proactively.
Related Resources
Digital twins may require organizations to collect and manage extensive unstructured data. Robust databases that allow users to query and extract valuable insights from such datasets become necessary.
Vector databases are one approach to managing unstructured data. The resources below will help you learn more about how vector databases work.
- What is a Digital Twin?
- How Does Digital Twin Work?
- Types of Digital Twins
- Digital Twin vs Simulation
- Benefits and Challenges of a Digital Twin
- Use Cases of Digital Twins
- FAQs of Digital Twins
- Related Resources
Content
Start Free, Scale Easily
Try the fully-managed vector database built for your GenAI applications.
Try Zilliz Cloud for Free