Vector Database Stories
From company news to technical tutorials – explore the most popular content on the Zilliz blog.
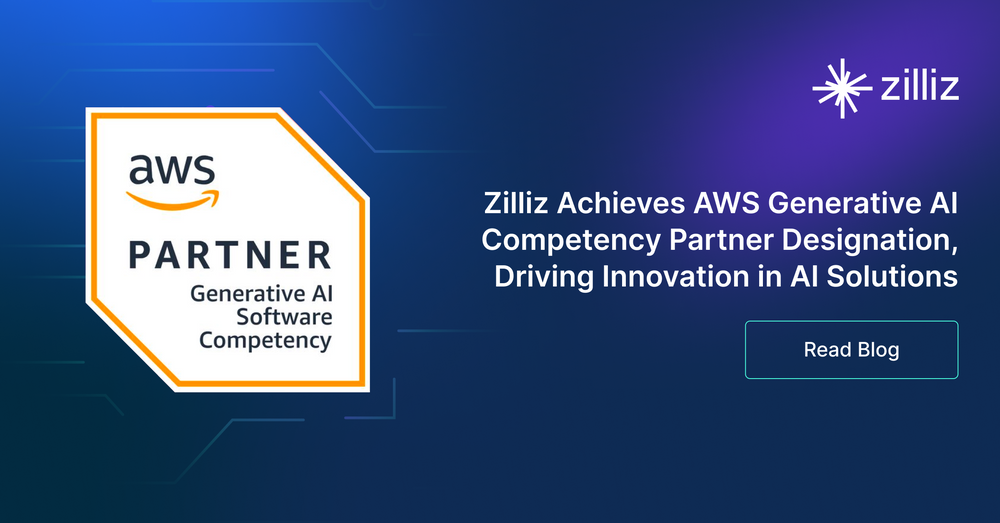
Company
Zilliz Achieves AWS Generative AI Competency Partner Designation, Driving Innovation in AI Solutions
Zilliz is proud to announce that it has achieved the AWS Generative AI Competency status, marking a significant milestone in our commitment to driving the advancement of generative AI technologies.
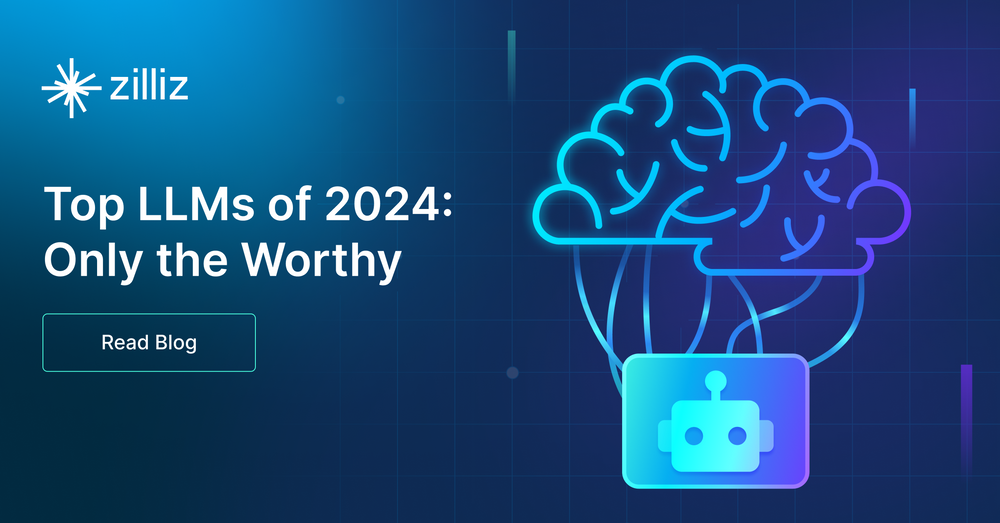
Engineering
Top LLMs of 2024: Only the Worthy
This blog introduces the six most influential large language models in 2024.
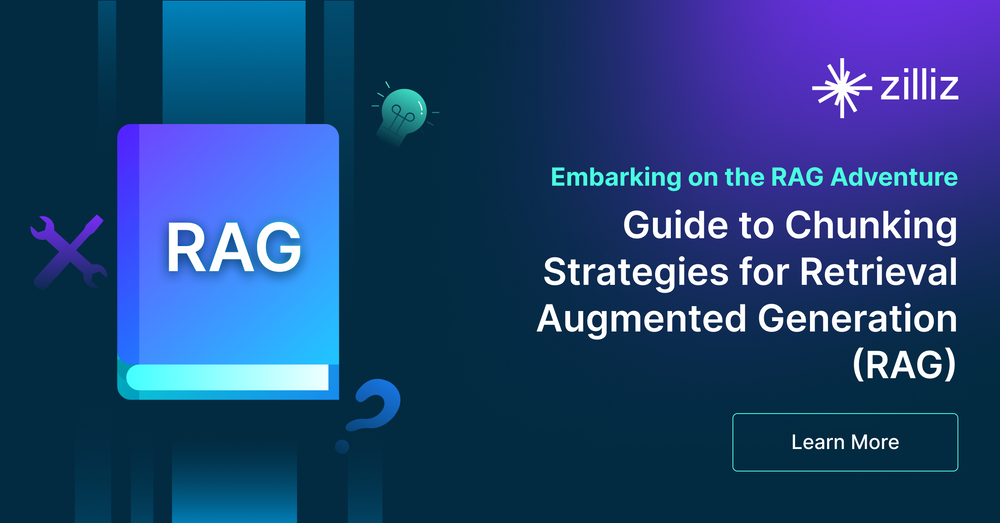
Engineering
A Guide to Chunking Strategies for Retrieval Augmented Generation (RAG)
We explored various facets of chunking strategies within Retrieval-Augmented Generation (RAG) systems in this guide.
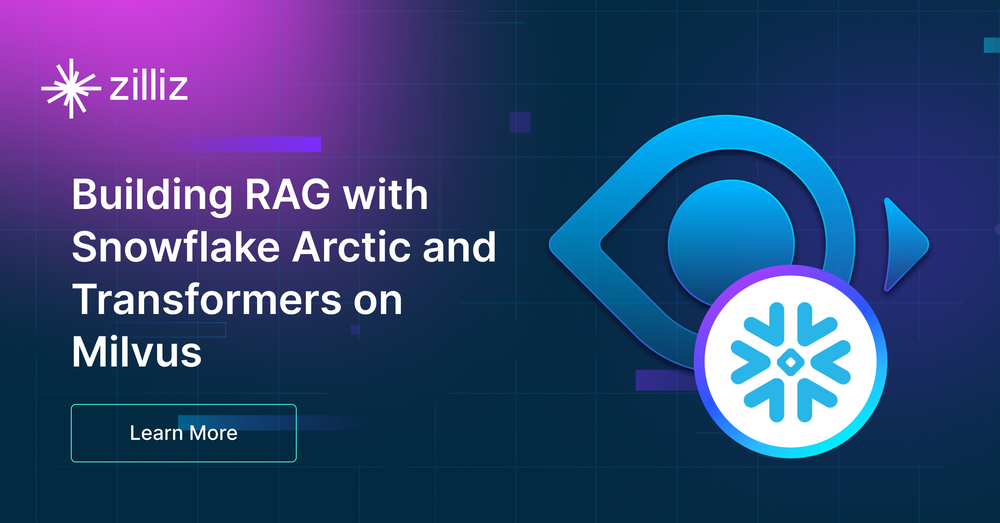
Engineering
Building RAG with Snowflake Arctic and Transformers on Milvus
This article explored the integration of Snowflake Arctic with Milvus.
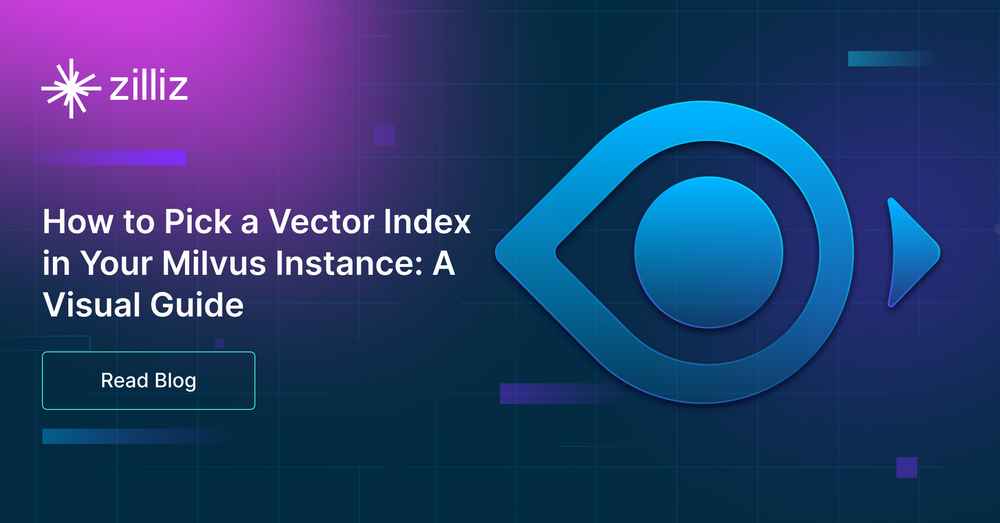
Engineering
How to Pick a Vector Index in Your Milvus Instance: A Visual Guide
In this post, we'll explore several vector indexing strategies that can be used to efficiently perform similarity search, even in scenarios where we have large amounts of data and multiple constraints to consider.
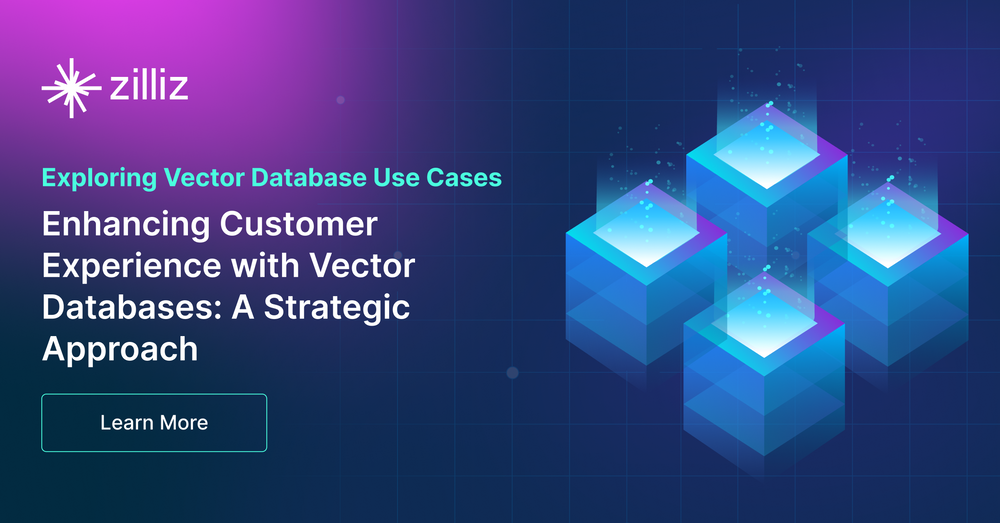
Vector Database 101
Enhancing Customer Experience with Vector Databases: A Strategic Approach
Understand how vector databases process data to enhance customer experience and drive business growth.
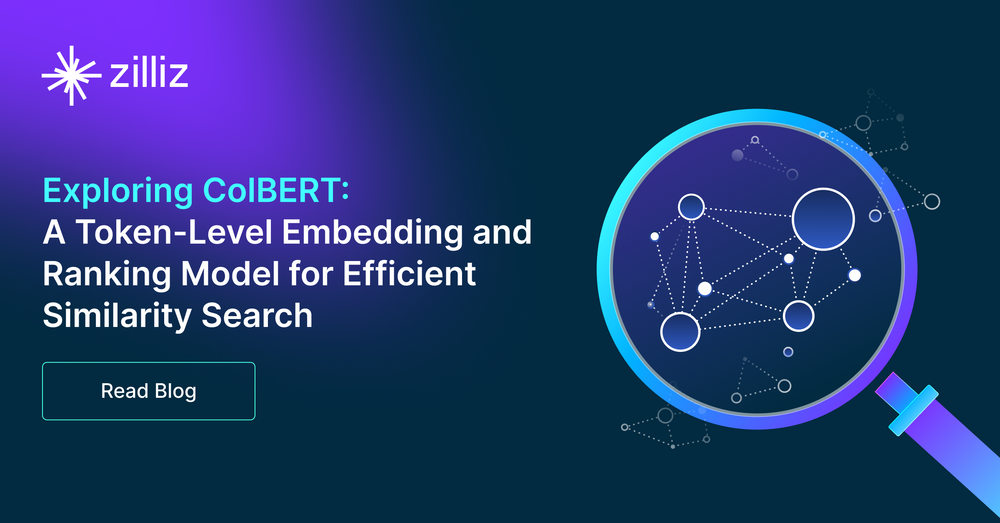
Engineering
Exploring ColBERT: A Token-Level Embedding and Ranking Model for Efficient Similarity Search
Our review of ColBERT has unveiled a novel approach to token-level embeddings and ranking, specifically engineered to optimize efficiency in similarity search tasks.
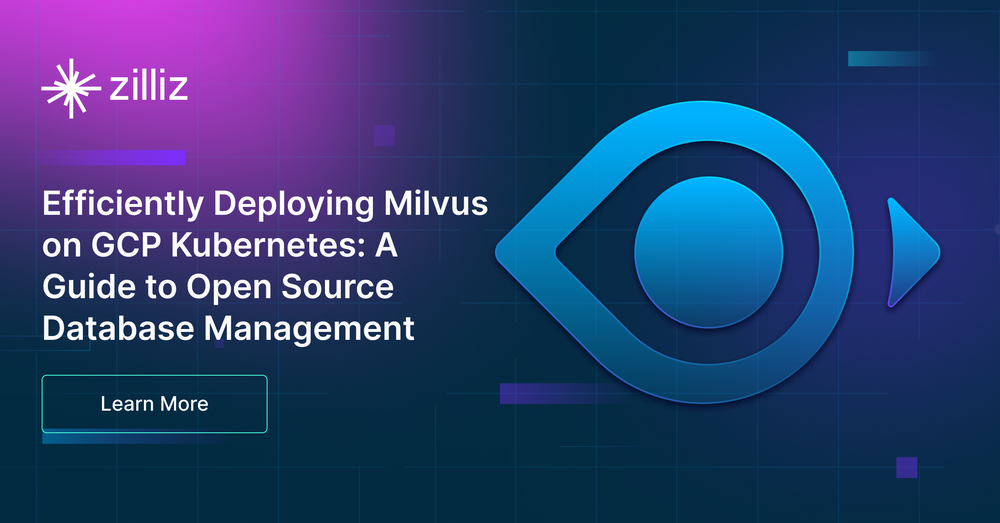
Engineering
Efficiently Deploying Milvus on GCP Kubernetes: A Guide to Open Source Database Management
Self-hosting Milvus on Kubernetes (K8s), especially in the Google Cloud Platform (GCP) environment, offers numerous benefits. Read about the benefits and how to set up the Kubernetes cluster on GCP in the blog.
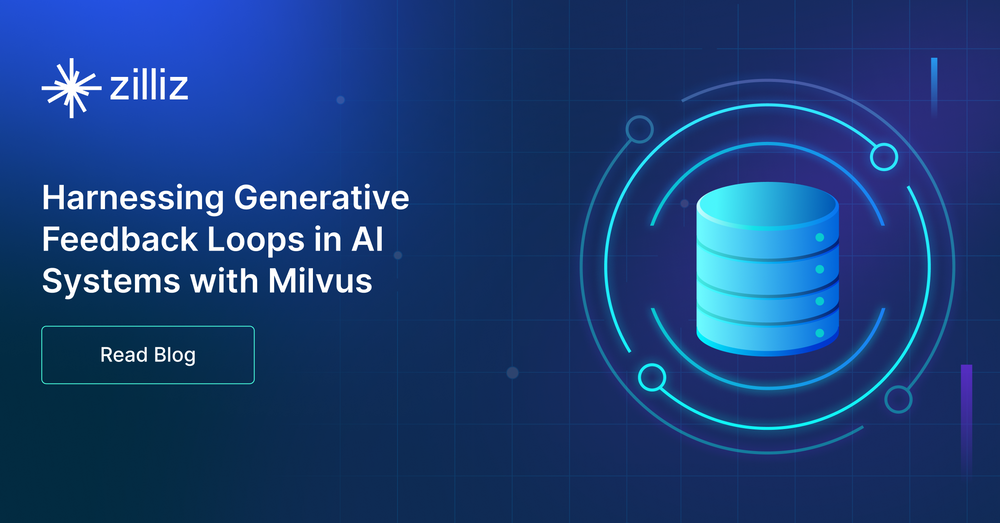
Engineering
Harnessing Generative Feedback Loops in AI Systems with Milvus
A generative feedback loop is a cyclical process in which the output generated by an AI model is fed back into the system as training data. This allows the model to learn and improve its capabilities continuously over time. This cycle repeats, allowing the AI to optimize its results progressively. Integrating Milvus with LLMs in a generative feedback loop allows us to create a dynamic system that continually learns and improves.