The Holt-Winters method, also known as triple exponential smoothing, is a time series forecasting technique designed to handle data with trends and seasonality. It extends simple exponential smoothing by adding components for trend and seasonality, making it suitable for datasets with consistent seasonal patterns, such as monthly sales or temperature data. The method has three components: the level, which represents the overall average; the trend, which accounts for upward or downward movement; and the seasonal component, which captures periodic fluctuations. These components are updated iteratively based on smoothing parameters, which control the weight given to recent observations. Holt-Winters is widely used because it is straightforward to implement and performs well for short- to medium-term forecasts. For example, it can predict retail sales during holiday seasons or energy consumption in different weather conditions. However, it assumes that seasonality is consistent over time and may not perform well when seasonality or trends vary significantly.
What is the Holt-Winters method, and when is it used?
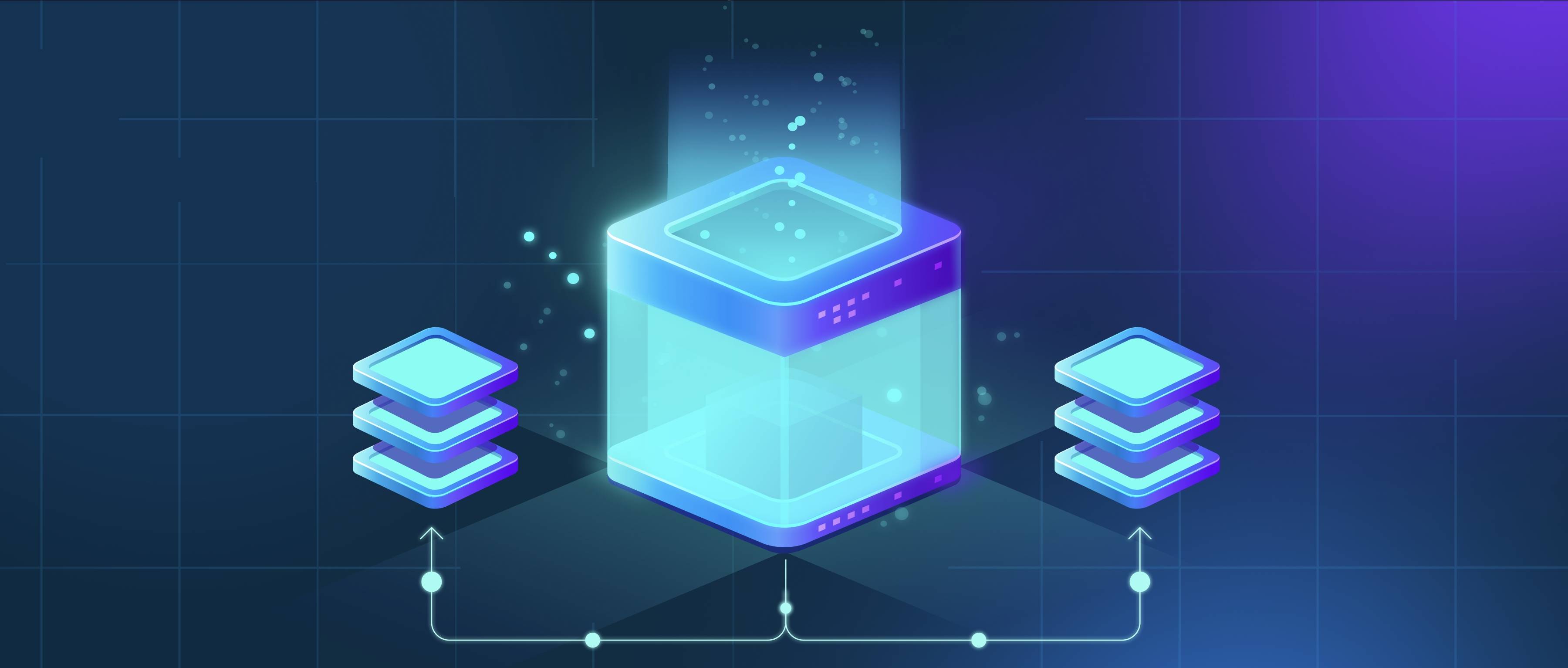
- Optimizing Your RAG Applications: Strategies and Methods
- Natural Language Processing (NLP) Basics
- How to Pick the Right Vector Database for Your Use Case
- The Definitive Guide to Building RAG Apps with LangChain
- Master Video AI
- All learn series →
Recommended AI Learn Series
VectorDB for GenAI Apps
Zilliz Cloud is a managed vector database perfect for building GenAI applications.
Try Zilliz Cloud for FreeKeep Reading
What are open datasets, and where can I find them?
Open datasets are collections of data that are freely available for anyone to use, modify, and share. These datasets are
How do you integrate ranking signals in search engines?
Integrating ranking signals in search engines involves the systematic use of various parameters that help determine the
How do agents interact in swarm intelligence?
In swarm intelligence, agents interact through simple local rules and decentralized communication, which allows them to