A lag in time series analysis refers to the time delay between an observation in a dataset and its preceding values. It’s a fundamental concept for modeling dependencies in sequential data. For example, if you’re analyzing daily temperature, the temperature today might be related to the temperature one day ago (lag 1) or two days ago (lag 2). Lags are crucial when building models like ARIMA or autoregressive models because they help identify patterns and relationships in past data that influence current or future values. In an AR(1) model, for instance, the value at time 𝑡 t is predicted using the value at time 𝑡 − 1 t−1. The inclusion of lagged variables allows the model to account for these relationships. To analyze lag effects, tools like autocorrelation function (ACF) and partial autocorrelation function (PACF) plots are used. These plots measure how strongly a time series is correlated with its past values at different lags, providing guidance on the significance of specific lags for modeling.
What is a lag in time series analysis?
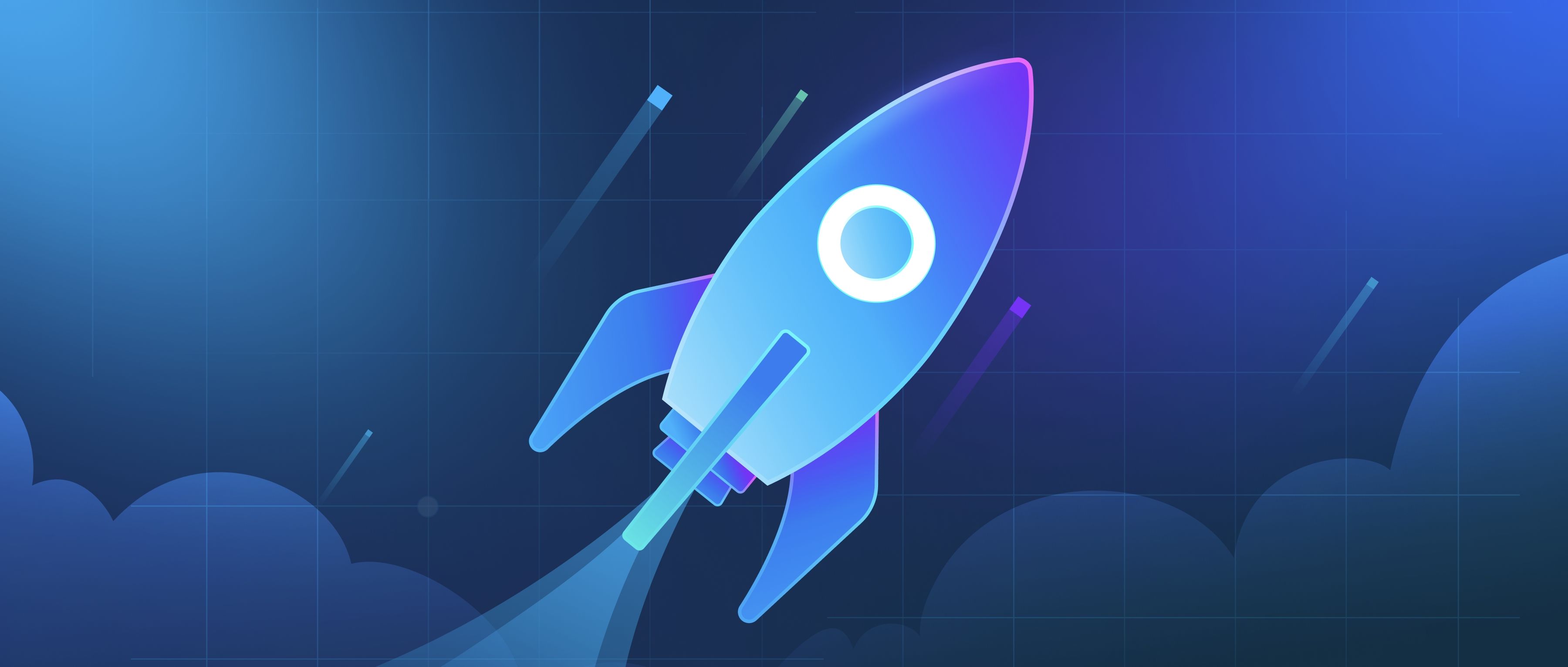
- Getting Started with Zilliz Cloud
- How to Pick the Right Vector Database for Your Use Case
- AI & Machine Learning
- The Definitive Guide to Building RAG Apps with LangChain
- Advanced Techniques in Vector Database Management
- All learn series →
Recommended AI Learn Series
VectorDB for GenAI Apps
Zilliz Cloud is a managed vector database perfect for building GenAI applications.
Try Zilliz Cloud for FreeKeep Reading
How does a typical ETL architecture look for a data warehouse?
A typical ETL (Extract, Transform, Load) architecture for a data warehouse consists of three core stages, supported by t
How do I evaluate the performance of a retriever in Haystack?
To evaluate the performance of a retriever in Haystack, you can follow a few structured steps that mainly involve assess
What is big data as a service (BDaaS)?
Big Data as a Service (BDaaS) refers to a cloud-based service model that provides businesses with tools and infrastructu