- Events
How MindStudio Crafted a No-Code Pathway for RAG App Builders
Webinar
How MindStudio Crafted a No-Code Pathway for RAG App Builders
Join the Webinar
Loading...
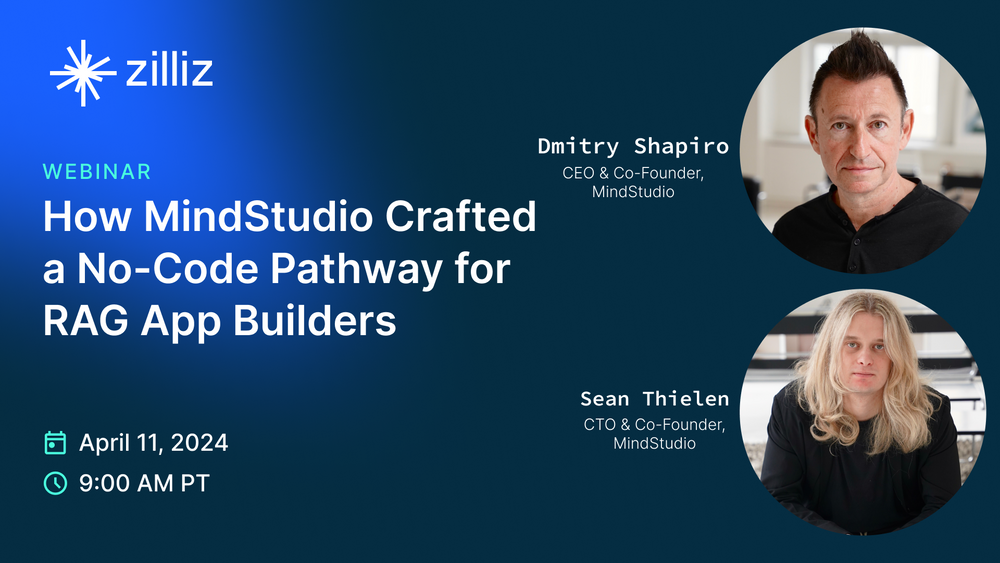
About the Session
Join Dmitry Shapiro, CEO & Co-founder, and Sean Thielen, CTO & Co-founder of MindStudio to explore the technical workings behind MindStudio: a no-code platform transforming how custom AI-powered applications are built across different sectors. We'll dive into how they made Retrieval Augmented Generation (RAG) accessible to all without any coding required.
What you'll learn:
- How MindStudio enables users to create GenAI apps without the need to manage or worry about vector databases
- The Role of Zilliz Cloud in boosting MindStudio's platform performance and security
- How MindStudio implemented Zilliz Cloud, what they envision for the platform moving forward, and the lessons learned throughout their journey
With that today, I'm really excited to introduce, uh,to this session how MindStudio crafted no-code Pathwayfor Rag App Builder and our guest speaker, Dimitri and Sean. Yeah. And Dimitri is the CEO of my studio. Uh, and Sean is his CTO. Uh, they view the MindStudio together.
How about you just introduce yourself a little bit?Absolutely. Yeah. Hey, uh, super excited to join you hereand, and chat with everyone. Yeah. So my name is Dmitri.
Um, Sean and I met, uh,10 years ago when I was working at Google,and Sean was finishing up studies at a university. And, uh, seven years ago, I left Googleand joined Sean to start, uh, mind Studio. Uh, the company's called Go Meta, uh,but the product's called Mind Studio. Uh, before this, uh, as I mentioned,I spent four years at Google. Uh, for twoand a half of those years, I ran product on three machinelearning teams that were sort of crunching all of thisimplicitly collective data.
Uh, Google has about, uh, you know, people, usersand, uh, helping to do a number of things. One is drive the adoption of, at that time Google Plus,which was a focus for Google. And so getting people onboarded to that, doing ranking on,uh, people you may know, people you may like, feed rankingand, and things of that sort. Uh, prior to that, I was the Chief Technology officerof MySpace Music. Uh, prior to that,I built two other venture backed companies.
One was a, a major competitor to YouTube called vo. Uh, raised $70 million there in venture capital. Uh, before that I built a, uh,enterprise software cybersecurity companycalled Iconix Systems. Uh, raised $34 million for that. We had over 2 million deployed seats in enterprises.
And in the mid nineties, 95 to 99,I built the web team at Fujitsu, you know,giant Japanese company. Um, and Seanand I, uh, again, have worked together for, uh, 10 years,seven years, full-time, sevenand a half years on, um, uh, on this company here. Sean, do you have anything you wanted to add?I think Dmitri mostly covered it. My, my background isMuch less Cool storied. That's awesome.
Uh,then tell us a little bit about my studioand what the business does. Mm-Hmm. Yeah, so we live in obviously a very exciting timewhere we all now have access to these, you know, new modelsthat we can, uh, we can access directlyand use, uh, and whatever. A year and a half ago when Chachi PT came out,everybody got excited about the fact that you can just sortof prompt it to do stuff and outcomes, you know, output. And some people might call that, you know, sortof work product where it can, you know, write thingsand analyze things and do all that.
Uh, but it became very clearto us pretty much instantly when we started playing aroundwith, with Chat GPTand obviously all the other, um, you know, language modelsand diffusion models and things like that, that, likewhile at it's cool to be able to just like sortof from a command line interface, prompt themand get 'em to do something, uh, the kind of the real power,uh, uh, you know, practical uses for them needto be something sort of more powerful than,than pe you know, people sort of writing prompts to it. And so we set off, uh, about a year and a half ago, a yearand a quarter ago, uh, to build Mind Studio. And so, uh, mind Studio is a platform, uh, that allowsanyone, but we're focused on enterprises, you know,organizations to, um, leverage any modelor model agnostic. So any language modelor image model code, video, whatever, any model, anythingthat be, can be called via APIand create, uh, basically, uh, you know,sophisticated multi-step workflows, uh, that allow, uh,enterprises to build, you know, automations,process automations, um, uh,to build custom business applications that are AI powered,to build specialized AI assistance for,you know, their employees. And we provide sort of the, the, the entire solution where,um, we provide the Snow Code builder.
I'll give you a, a quick demo towards the endof this webinar of how that works. And you can always go to, uh, you know, mind studio. ai/learnand, and find lots of tutorials on that or, or on YouTube. Uh, so very quickly you can learn to use this builderand create these workflows. We provide all the things that are requiredfor enterprises to adopt this.
So, you know, granular user management, logging, archiving,compliance, things like that. Um, we allow them to connect to any of their,you know, data sources. Um, and, uh, obviously this is also where, where, uh,CIS comes in, uh, uh, as part of that, uh,and, uh, obviously we allow 'em to integratewith their entire tech stack that they already have. So basically the typical approach enterprises take is they,you know, discover us organically. By the way, 86% of our growth is organic.
Uh, there's now over 40,000 of these AI applicationsthat have been created with Mind Studio and deployedand, you know, giant enterprises, government agencies,thousands of SMBs. Um, and, uh, I said 86% is organic. We don't have a single sales person in the company. Uh, we're a self-service, uh, uh, platform. Uh, and so typically how it happens is they discover usand again, learn to use it by watching a YouTube tutorialand then sort of look at their processesand start to realize that a bunch of thingsthat they've been doing, you know, manually with humans,they can just automate those.
And we make it really easyand fast for them to create these ais. You know, the typical AI build is anywhere from like 15minutes to a couple of hours for one person,and you can build like really, really powerful automationsthat like, again, take humans out of the equationfor certain parts of the workflow. And so that's the first thing you do. Once you've done that, you kind of look at the rest,the remaining thing that humans still needto be involved in, and,and start to realize that,that those employees could use really custom new custombusiness applications to help them do their work moreefficiently rather than sort of using these solutionsthat they were using before all the automation happened. You know, like, like deep heavy CRM systemsfor most organizations, they use only a few featuresof these CRM systems,but they've sort of been forced to adoptthese giant systems.
In order to do that, well now they can actually rip outoftentimes those CRM systemsand just build custom business applicationsfor these remaining employees that have some functions that,that are CRM e but don't require these heavy CRM systems. And so automate everything that can be automated for thingsthat can't completely be automated. You still need employees create custom tools for them. And then for everything else, sort of like ad hoc on demand,once in a while, I need to talk to a modeland do something, uh, that's not repetitive, sort of, uh,instead of using sort of directly allowing employees to goto, you know, Chachi PT or Clotor any of these things, uh, buildfor them very rapidly these specialized conversationalassistance that, uh,behind the scenes still leverage Open AIand drop Google Meta Mistralor their own private models, you know,some large organizationsor once they're very sort of privacy, um, uh, uh, uh, uh,and, and, and compliance and,and, uh, regulatory impacted, uh, tend to, you know, buildand run inference on premise or in private cloud. We allow them to connect to that as well.
So they might be using these things,but these conversational specialized assistance perform muchbetter than just generic chat GPTbecause they're aware of the enterprise in which they,they sit and, and sort of the constraints of the enterpriseand, and the sensitivities of it and,and the internal data, uh, they are awareof the job function of the person that is interactingwith them because again, they've sort of been, been put intothat state of context so they can understand that, andtherefore they require like radically less prompting and,and, and produce much better, you know, output. And again, we make all of this really easy to dofor enterprises to do it. And, you know, that's why since launch in Septemberof this last year, uh, we now have over 40,000 of these, uh,ais that have been deployed. I, I hope that made sense. Yeah, that sounds awesome.
Um, but what I learned is, um, they're easier,you make the productthe more complicated it's added at the backend. So I would love to hear, uh, which kindof a tech technical challenge you face, um,when you build this, um, amazing application. Yeah, I think, um, it kind of dovetails nicely withwhat Dmitri was just saying, which is that the AI is verynoisy as a space right now. There's, there's so many cool things happening all the time. Every day you wake up and you hear about some new model,but all of a sudden is better at getting into medical schoolthan the last model.
Or, you know, here's a new vector database,or here's a new, you know, thing that does something else,or here's a new style of applicationor a new way to, you know, program agents to do things,and all of a sudden that's all the rage. So I think the biggest kindof technical challenge we faced actually hasn't beenat the code level, hasn't been at the implementation level,it's been more about kindof looking at this amazing landscape of interesting thingsthat are coming outand kind of first picking which ones are the onesthat we think are valuable that we want to bet onthat provide actual real value to end users,especially non-technical end usersand enterprises, like, which are the toolsthat are coming out, are thingsthat people can actually use rather than, you know,interesting demos that two weeks laterafter we do all the implementation work,no one's gonna be caring about anymore. And then taking that and transforming that kind ofnerd coded, um, productlanguage into something that normal people know how to use. So, like most of our end usershave never even heard the term factor database before. Like, they certainly don't know what Zills isor are trying to make comparisons based on, on that.
Like, they don't understand the inner workings of this. They might have heard the term, you know, rag thrownaround somewhere and thought, oh, I need thatbecause that's popular. And so that's kind of the level of individualthat we're trying to basically play translatorto in taking these amazing core technologies and innovationsand bringing them to ordinary peopleand letting them figure out what it isthat they can do with them. Which is why, for example, like someof the language you see reflected in our interfacesdoesn't map one-to-one to what's happening on the backend. Like we talk about data sources, um,we don't really talk about vectorization that muchaside from like in, you know,some little progress bars here and there.
But we're trying to basically take these conceptsand uplevel them into things that ordinary people can,can understand, and oftentimes, you know,compose multiple things in the backendof our system into some new product that from a, you know,non-technical end user's perspective, they're gonna look atand be like, oh, this is something new. But actually it's, you know, a mix of vector databasesand LLM calls and other typesof orchestration on the backend. Uh, Dmitri, is there anything you wanna add to that maybe?Yeah, I think that's spot on. Uh, yeah, let me, let me contextualize a little bit more. So again, our focus is bringing AI enablementto enterprises, to organizations.
And by the way, when I use the word enterprise,I don't necessarily mean large organizations. There's thousands of smalland medium sized businesses that, you know, have builtAI using Mind Studio and, and use them. So any kind of organization, uh, and,and today these organizations find themselves,and again, we get to sit at this like really interestingvantage point because, you know, thousandsof organizations are coming to us. Uh, some of all of it is self-serve,but some of them reach out to us and, and,and sort of we, we ask 'em questions, how did you find us?Like, what were you looking for? What were your pain points?Things like that. So we get sort of a lot of signal of like,what's happening out there and,and there's just a tremendous amount of excitement, uh,of like, wow, this thing is amazing.
I can like prompt it to do stuff. And, and then confusion like, what do I do with it?Do I need to hire a bunch of data scientists?And I also read that like, there's now a shortage of HH 100,so I gotta figure out how to procure thoseand build a data center and all of that. Or do I need to go and do like an RFP and,and go figure out which, you know,large language model we should standardize on?Or should I go and implement like someof these like vertical SaaS tools, you know,like writer Jasper copy,and then there's others for, you know, marketingand there's others for sales and others for operations,and how do I make those decisions?So like, there's this great demandthat I should be doing something with ai,otherwise my competitors using AI are gonna kill me. But on the other hand, I don't know what to do with it. And so we show upand we say, look, don't worry, it's actually really simple.
You, you can learn to use this product in an afternoon. And then you, no matter who you are,whether you're a developer in the companyor you are a project manager or an HR manageror a marketing manager, these are like the types of rolesthat we see Discover Mind, studio, and,and begin to implement these things in their organization. Um, and, uh, and so we make it accessible and, and apply itand, and allow people to solve real problemsand not sort of take on this sort of, uh, um, perspectiveof like, the way you do AI is you wait for the next modelto come out and you wait for like a GI, whatthat's gonna be GPT eight and GPT eight. All you'll be able to do is say,please make my enterprise better and push send,and it will build your business planand refactor everything. Go raise money.
And you say,make it a trillion dollar company, and it goesand sells it for you. Like some people sort of think that this is the path toactually leveraging ai, and wesay to them, look, that's silly. You can do incredible things with it now. And, and, and again, we're seeing that happening across,I think every industry already. You know, with these 40,000 AI deployed, uh,every size company, uh,almost every country represented now,I think not every country.
I I would also add to that, um, that I think,and this gets back to the technical challenges, isthat we provide an answer to people who have anxiety around,oh, well, if I standardize on this one tool chain today,or this one model today, you know,what happens in a week when all of a sudden that's outdated?Do I need to refactor my entire business?Like, an analogy that comes to mind would be like,if you were got traveled back in time 50 yearsand had to teach people photographyand you knew that the iPhone was coming, it wouldn't be herefor a while, but like, what would you teach them?Like, you wouldn't tryand teach them about crazy future in the weeds things. You would teach them the basics of composition and lighting. And, you know, the reason that we're doing all of thisto begin with, and I think Mind Studio provides a similarlevel of abstraction with AI application development,which is don't worry about the model. The model's gonna keep getting better and better and better. It's gonna get faster, it's gonna get less expensive,you know, data sources and data retrievaland all of that is gonna get betterand easier and more contextual.
So what you should be focusing on,unless you wanna go get a PhD in machine learningand go play in that field, that's awesome. But if you're just an ordinary business person, the thingthat you should be focusing on is how do I identifyprocesses and articulate them in kind of natural languageand in these sort of structured ways that I can then useto communicate with AI broadly. And as AI gets better, you know,that fundamental thing will be refined,but that fundamental thing is still, like,it's an exercise in learning how tolook at the work you're doingor the work that the people around you are doing,and define it in discreet waysand communicate it in waysthat machines are able to take advantage of. Mm-Hmm. And obviously with, with ai, uh, the roleof the software developer, um, uh, you know, evolves,uh, obviously it evolves outside of anything we're doingwith like copilots and,and you know, you know, models that, thatthat can write code, um, uh,and it also evolves in the enterprise.
So in enterprise, it used to be you either sortof build your own, and that means you needto either hire some development shop to do it,or you have your own developers, you know, build and,and maintain, you know, products and roadmaps and such. Um, or you, uh, adopt a no-code, low-code platform,uh, sort of the last generation of these platforms. And, and on one hand that enables yournon-developer employees, business usersto build applications. On the other hand, like if anybody's used, you know,these low-code platforms, uh, no-code, low-code platforms,uh, you realize that the amount of work requiredto actually build thingsthat are meaningful is oftentimes much more work than simplyhaving written a code. And so on one hand, you have a bottleneck of developersthat are required to build stuff.
On the other hand, your business users, you know, haveto do a tremendous amount of workand learn to use these very sophisticated tools. And because, again, in the old world of development, you hadto sort of like, uh, uh, uh, think aboutand cover all, you know, uh, edge cases, let's say, and,and sort of enumerate them and, and, and,and, you know, build rules for them. We live in a fuzzy world now with AIwhere you can generally tell it your, you know,sensibilities and, and it can make a lot of assumptions,and then you can sort of fine tune thatand give it some additional constraints. So just a very different way of,of building these applications. And that makes, again,building them radically more accessible.
Many more people can learn to do it. It makes building them, you know, ludicrously faster. Again, sort of 15 minutes to a couple of hours. You're not gonna build anything meaningfulwith a no-code platform in a couple hours. Uh, but here you do, uh,and by the way, these things can be deployed, you know,when, when you build them in, in our, you know, web IDE and,and test them and preview 'em,and then, you know, you push publish, uh, they get packagedas, as web applications, right?So they're, they're, they're, you know, uh, uh,accessible via a URL and,and they can sort of be in two flavors.
One is they can have human interfaces, so for, let's say,employees to use, so like a regular web app. So have input output in, in the form of, you know, imagesand text and media and forms. Uh, or they can be headless, you know, specifically meantto be driven via, you know,some other process programmatically, API calls, uh,and obviously those are the ones that are primarily usedfor, uh, automation. And so oftentimes we see people, uh, you know,triggering them either again, sort of from their own custom,uh, software, uh, and, and, and calling our APIsor using things like Zapieror make. com, these things that allow you to create sortof these event listeners, uh, triggers.
They typically call them and then, you know,call some process, in this case our APIendpoint and start that off. And so you can build componentsand we make that accessible to non-technical peoplethat don't even know what API means,but they understand what it needs to do. And so they can build that, and then they use Zapier and,and en route it to it,and all of a sudden you've got, you know, uh, uh,software development for the modern day. That sounds awesome. Um,actually we do have like a, a question.
Let's, let's try to, uh, pick that up first. So for data analytics, there was a sayto four state describe Diagnos project prescribewith the ADV event of j and I. Do you see this involving or changing?Sorry, I, you broke up on my end. I I couldn't quite hear itIn the, in the q and a tab. Dmitri, if you wanna open it.
Okay. Uhhuh. So say the fourth stage for data analytics,describe diagnostic predict and prescribed. Do you see this will change with the gene ai?I don't, I think this is a, a good example of kind ofwhat we've been talking around, which is likegenerative AIand a little bit what Dmitri was describing with its abilityto accept kind of buzziness and imprecise. This actually allows us to focus on these foundations where,you know, the, like, it's great if you sit in adata analytics courseand they tell you, oh, there's fourstages to data analytics.
Describe diagnose, predict, describe,and then you spend 90% of your daysitting in a Jupyter Notebook trying to debug, you know,three lines of code. And you don't actually get to focus on, on those kindof foundational high level conceptual abstractionsthat actually make the work valuablebecause you're so mired in, in trying to connect everythingand, and get it to work. Precisely. So I think, yeah, moving forward,and I think this applies to a lot of, um,advances we're seeing in software developmentand what Dimitri was speaking to with the evolutionof these no-code or low-code builders. Um, the, we actually have spacefor a renewed focus onwhat problem is it we're solving,and why are we solving it, rather thanthe world we live in now, which is like, okay, great,we can do that, but then we needto actually devote the majorityof our time toward doing the actual implementationwork and diving in the weeds.
Now that paradigm is a bit inverted where we getto spend most of our time describing the problemand figuring things out and figuring out theright way to articulate it. And then the implementation detailis more hand wavy in that sense. By the way, I apologize. I found the tab,I was looking at the chat tab and QA tab. I'm like, where is this question? Sorry about that.
That's awesome. Um,then let's switch in the gear a little bitto talk about a Vector database. Uh, I actually have heard you guys had a different thoughtsinitially, uh, instead of like, uh, uh, choosingor fully managed vector database that you are thinking aboutto build something yourself. Um, so I would love to hear what exactly happened, uh,what do you made you feel, oh,actually I need a fully managed vector database over there. I think 'cause the, the benefits outweighed the costrequired to build and enroll it ourselves.
I mean, that was, I think probably the biggest thingthat went into our choice to use Zeus was justthat you guys have been around for a long timeand very stable and no nonsense and fleshed out. And yeah, it's been, um, a, a very,very positive experience so far, especiallybecause we are building out kind of this, um, you know,multi-tenant solution where on a singlecluster we are serving a lot of different clients, um,data and we're, we're loadingand unloading dynamically based on usagepatterns of our users. And so having kind of accessto the lower level database versus some of these kindof newer trendier services where you don't haveas much visibility intothat was actually really important to us. So I think it, it's unfortunately not a, the answer isthat that Zillow was, was boring and stable,and that was why we chose you, which is unfortunately,I think not, not as fun of an answer as it could be. But I think it's actually a really important answer.
And I think it, it speaks to the, you know, valuesthat we have as a company when choosing technologies to, to,you know, make a bet onHmm. As a data infrastructure company, we love to be boring. Yeah, yeah. That, that's, that's actually the greatestcompliment, you know, there can lo it at anyone is,is boring, means you're not trying hardto impress you just do, you just work. And, and that that's our, that's our, uh, approach as well.
We, we try to be boring old infrastructurethat just lets people do amazing things. Mm-Hmm. Yeah. And I think if you're delivering real valueto people as, as you guys clearly are, then you don't needto dress it up with all the other stuff. Mm-Hmm.
Um, so do you, do you wanna take a,talk a little bit more about a multitenancy?We heard this a lot from the community, uh,especially loss of Virginia app. They want to build this kind of multitenancy way,but they face like a lots of, uh, challenges. So I would love to hear a little bit about yourapproach. Mm-Hmm. Yeah, I think Probably the most important contextbefore we dive too deep into that is just the, the shapeof our production trafficand workloads, which is, we have a lot of users,and as Dmitri mentioned, uh, the majorityof them are building kindof internal facing enterprise applications.
So, you know, um,Dmitri you can probably speak a little better than I canto types of datathat people are uploading into their datasources that we are storing. But the gist of it is that we are not doing a lotof like high read workflows. So we, um, and there's a lot of kind of like,someone might build out asales training assistant,and as part of that, they'll upload a lot of conversationsand product manualsand all kinds of things like that into the datasource for their application. And then that application might be used, you know,during predictable hours during the work weekand only be used by a few peopleto make a few queries here and there. So we can do some simple kind of heuristics on top ofthat usage and dynamically loadand unload the data from, um, the Vector database.
So I think that that's been the biggest thingthat's allowed us to do multi-tenancy in a pretty scalableand cost effective way, has just beenthat we don't have the constraint of allof our customer's data needsto be loaded into memory a hundred percent of the time. So yeah, we, um, load things inand out pretty aggressively. And, um, the other thing too is that we have basically,we've basically built out adapters in such a way that forour enterprise clients who do demand or,or do require ratherthat their data be accessible at all timesand be loaded into memory, we can just let them, you know,plug into their own whatever reserved instance if they wantto do that and, and get, move offof the shared infrastructure. Cool. Um, the other sort of question I have is,um, when we talk to the community, mostof people when they build wrap, they use some sortof framework, let's say launchand log index to make the whole process much easier.
Uh, what I've heard is you guys actuallynot adopting that approach. I would love to hear the rationale behindMm-Hmm. Um,I think we didn't, honestly,implementing your guys' APIs was easier than learning howto use one of those frameworks and try and figure it out. Um, I think it was, yeah, I, I, um,this could be a whole separate, separate conversation. Like, the work that Lama and Dexand Link Chain have done has been amazing,and I think it's very valuable, especially for, you know,lots of developers who wanna scaffoldthings up and get things going.
Uh, we kind of wanted to march to the beatof our own drum a little bitand not find ourselves going downthe paths that others had kind of drawn, especially givenhow fast this, this space is moving. So like, I basically just wanted kind of some API endpointsto be able to load in data, query data and,and manage it, and,and then we can compose that in the way we want to,rather than, oh, we're kind of locked into a patternthat's been prescribed in some other way. So I think like if you look at, you know, if you wereto look at the architecture behind Mind Studio, it's a lotof, basically just at the, at the core layer is allof these, you know, foundational services, the LLMs,the Vector databases, um, you know, as wellas just generic kind ofless exciting tech things like just third party APIsthat let you do things or, or scrape websitesor user management, whatever. And then us building kind of adapters on top of thatand then composing those. So in effect, we're, we're doing a similar thing towhat some of these frameworks are doing, andbecause of that, it felt important to basically ownand define our own pathways to that infrastructure.
And then, you know, in some, I think you could think aboutwhat Mind Studio is, is, is a basicallyno code version of something like a Lang chain, you know,for, it, it, it's opinionated in some senses,but I think much more accessible than, you know. 'cause I think, and I mean this in the nicest way, likeif you know enough about programming to integrateand implement L Chain, you might find yourself gettingfrustrated enough that you end up just doing the thingyourself versus where we sit, which is, you know, mostof our users have never seen a line of code in their lifeand have no desire to evertouch a line of code in their life. So I think we can get away with a lot more in termsof being opinionated about the way we express access to,to our tools than something that sits a little closer to,um, like raw programming. Mm-Hmm. I, I think a follow up question for me, uh, forthat is, um, or that Dimitri, uh, mentioned earlier,you just really don't want your userto worry about the newest kind of attack,the model, et cetera.
Uh, how are you make sure you can keep involving,uh, and just like, uh, do the best thing for, for them?'cause it's hard just like keep upwith technology to go so fast. Mm-Hmm. Yeah. I think that's where us being able toleverage the economies of scale that come from sittingat this unique vantage point come into play. So we, like, if you're just a, you know, someof our customers are like random, you know, restaurantsin random parts, they, they're not technical.
They don't want to sit there and read news about, you know,benchmarks on different models and doing things,but we actually can, we have the resources to be able to sitand implement all of these things and,and look out at the world and decide what's happening. Um, the other thingthat I think becomes interesting moving forward, you know,for certain types of customers that wantto opt into these sorts of things is, um, cost savingand efficiency that we will be ableto basically look at different models relative to pricesand relative to specific actions in workflowsand help customers pick, you know,what is the most effective thing for me to do here. Like we had, um, because a lot of people just don't know,like we had Dmitriand I had a call this morning with a customer who, um,they were trying to troubleshoot a data source,and they had built this full data source to upload one,maybe like 50 line text file into it and vectorize thatand put that into Zillow and be querying it. And it's like, okay, that's awesome that it's so easyfor you to do that, that you're not thinking about allof the steps required to do this. But at the same time, really what you should be doingand what, you know, our automated tooling in the futureshould catch and help you do is you can just pastethat into a prompt if that's how you want to access it.
Like, that doesn't need to be used in this way. So helping, um, these end users, you know,not only access all of these things,but help them understand when they don't needto be using things or when they'd actually bebetter off using something else. Like a, that's, I think a common pattern we've seenwith customers is they show upand they want to use all the latest and greatest everything. They want to use, you know, GPT-4 Turbo and,and Cloud Three Opus, and they want to vectorize. We've had people show up and be like, I have12 million words, can, why can't I do that?And I'm like, okay, do you actually need any of this stuff?And the answer most of the time is, is no.
And we've actually found kind of an interesting correlationbetween how deep people go intoLearning Mind Studio and playing with thingsand building the applicationsand their kind of jadednesswith the shininess of the new technologies. Like a lot of these people who start out using expensivemodels and building complicated workflows over time findthat actually they would rather have lower latency,you know, use a smaller model for certain actionsand then bring in GPT-4 at the end to synthesize allof this stuff, or, you know, be more thoughtful rather thanlet me take every single file on my company's shared harddrive and just dump it into this thingand pray that it works. I'm actually gonna be a little more thoughtful aboutwhat are the data sources that I'm choosing to include,you know, and, and what are the ways in which I invoke themand can I do something like, you know,split them up into maybe three separate databasesand based on, you know, classify the user's intent firstbefore querying, though. So I think you get a little bit more of the kindof sophistication you see as it, it,it actually tracks pretty well toward kind of seniorityand programming is kind of the analog that comes to mindof like, junior developers getting excited about all thesenew frameworks and all these new toolsand building these crazy things versus, you know, the,the jaded gray-haired senior engineer who just wantsto write as littleand as boring code as possible to get something done. Cool.
Uh, let's get backto the Vector database for one more question. Uh, we actually, I think the community would loveto hear if you ever encounter any issues when you startedto use Zeus, and if there's anything we could dobetter to help you guys. Um, I don't think so. I think there were a few places where,and this may have been resolved,but where the documentation between VISand Zillow, um, wasn't quite in sync. So I would be like, trying to use some parameterand I'm like, why isn't this working?Um, but you know, that that's I think, the funof implementing any, any new technology.
No, I think we've had a, a really smooth, um,great experience so far. You know, it, it does what it says it's supposed to do,and it does it really reliably. And yeah, it's been, it's been great. It's nice, especially in such a complex and,and, you know, wide reaching software product as my studio. Anytime there are things where I'm like, I can set this upand then I don't need to, you know, set a mental reminderto check up on it every couple daysbecause I just trust that it's gonna work.
That's, I'm beyond grateful for that. So, yeah. Thank you. Yeah. That, that's actually, I mean,you guys don't know us, but like we're, uh, uh, that's rareto get us to feel like that mostly we're,we're b******g about somethingor another, uh, that, that we wish it was better.
And, and so the fact that this thing just runsand, uh, we tend not to have to think about it, there's aas, um, mm-Hmm. Big blessing. We really appreciate it. Mm-Hmm. We're glad to hear thatand we'll let the doc team know.
Actually, we had some improvement about the documentation,so if anyone wantsto check in now, it should be a little bit better. By the way. Uh, heads up, we are, uh, uh,we're growing really, really rapidly andtherefore we're always playing catch up. And so, uh, our documentation is often out of date. Yeah.
Uh, fortunately our support is awesome, uh,in primarily in Discord, so jump in there. So don't be surprised if you are like, what is this?This interface doesn't exist. Oh, that's right. It's evolved. And so probably,Probably bad karma for me to be complaining aboutquality of documentation.
Well,No, no, that's what I'm saying. You weren'tcomplaining, you, you were actuallycomplimenting Mm-Hmm. Uh, because, uh, ours is, uh, radically worse. Okay, cool. Was that, uh, Dmitri,do you wanna show a demo to the audience? Oh, sure.
Uhhuh. Yeah. Yeah. So let me show you a quick demo here. And by the way, the best way to get a demo here is like,I'll tease you a bit, but,but the right way to do it is, um, uh, just to goto learn over hereand, you know, uh, watch this 18 minute videoor this two hour course, or on our YouTube channel.
There's a bunch of deep dives. We also host weekly webinars where we do basically sortof a version of this and we host an advanced webinarwhere we show you how to integrate it, you know,with things like, um, uh, Zapierand Make and things like that. And we do an eight hour, uh, on Saturdays, uh,like every other Saturday certification cohort. And so you can take an eight hour classand then complete a project and, and be gradedand become a certified developer. 'cause we now have a lot of people that are reaching outto us enterprises that are reaching out to us wanting,you know, help in implementing these things.
Again. And by the way, here's a quick ma matrixbefore I show you this demo of just, again,we're bubbling up some like, common patterns that we tendto see of like what people are doing with Mind Studio,what kinds of things are they building. So, you know, take a look at thatand in that super helpful, uh, as well. Okay, so once you've, you know, uh, signed upor, you know, continued with, with Google or,or, um, apple, uh,or email, um, you basically get an interface like this. One thing you get by default is basically a replacement for,um, again, chat GPTor any other model that you wanna use by default.
By default here, it's GPT-3 0. 5 turbo, but I can edit itand completely change what model. My default, this is my conversational assistant. Again, it's made to just replace my use of, uh,large language models directly because I can do it here andbecause I can edit it and it can be trained either by meor by my enterprise on, you know, what we doand what my job function is,it can be much smarter about that. Uh, and then you can hit plus into create, you know,more tabs here.
Obviously I've got a bunch of things that I've created,but you would just have this create AIbutton and you can click that. And so you can start creation of ai,uh, in a number of ways. Well, first of all, I'm aboutto show you in this next screen sort of the anatomyof these AI applications. But part of that anatomy is, is, you know,I think it was like a system message, a promptthat sets the context for this AI thing you're building. Like, what is it, what is it supposed to do?And generally, you know, how, how should it behave?And you can do that either by choosing a template,or you can do that by telling the AIwhat it is you're trying to build.
And just some words. And it will automatically using ai generate this prompt. Or you can just start from scratch. Uh, it's actually not completely blank, but mostly blank. And that's what I'll do.
And so it gives you sortof this blank prompt, you know, the role of the assistant isto help the user by responding to their requests. That doesn't really say anything. 'cause you didn't even have to say that,but this is sort of the thing. Okay, so now you get our id. Um, it's basically broken up into like three columns, left,middle, right on the right hand side.
You've got this live preview, so you can chatwith this thing as you're using it, uh,on the left hand side or various resourcesand, you know, air detection and debugger. Um, and then the in the middle is kind of where the,the majority of the work, uh, happens. Um, so an AI consists of, uh, again, uh, uh, oneor more of these things we call workflows. You get this main workflow, but you can add additional ones. And workflows consist of a system prompt, uh,a default model that you get to choose,but you can override it with each step that you take.
Um, and by the way,you can also using these custom functions that we allow youto do, call any on-premise modelor again, any other API endpoint. Uh, and, and primarily these things we call automations. Uh, and these are these multi-step workflows, um,that are represented by these, uh, these workflow thingsand by the, and you add nodes to themby clicking this plus sign. And so they, they have a start, they have an end,and they have, you know, things that they do in the middle. And here, you, you can create different types of nodes.
I'll just quickly run you through some, if you're building,uh, an AI that is meant to be used by a human,let's say an employee, it's a tool for employees to use to,you know, uh, generate some, you know,work product that, that they're doing. Uh, and so it requires some user screens, user inputs. And so you can use this user input block right hereand create these different types of user inputs. You know, on the right hand side are these previews thingsthat are long text, short text, you know, multiple choice,visual, multiple choice, you know, rating things. These are constantly sortof being extended, uh, of what you can do.
And, you know, obviously manipulate themhere, give 'em nice images. You can make these look really niceand create really nice, uh, forms, screens basically, uh,for, uh, uh, people to use. Uh, the other thing you can do, of course,is you can send messages to, uh, models. And again, as I said, there's this default model herethat you can choose, but at every step you can override thedefault model of what model are you calling in. Most AI applications that we see people build nowuse multiple models and typically from different providers.
And we make it real easy to do. They don't even need their own API keys. All of these are just multi-tenants on our API keys. And then we just bill you for usage. And so you can use, you know, anthropic and, and open AIand minstrel and, and meta and all of that,and then send them messages.
And then you can, you know, send them messagesas a user, as a system. And then the response can either be displayed to the useror can be assigned to a variable,and then sort of piped through to, you know, other things. The other thing you can do is you can, uh, goto this data sources area and say, plus,and you can, uh, upload a data source, uh,upload files and create a data source. I'm gonna zoom in. Here's upload pdf, CSV, Excel text doc,anything textual, uh, directly from your computer.
Uh, we set a limit here that you can ask us to override,but, uh, just sort of for abuse protection,but like half a million wordsand up to 150 files simultaneously. I'm just gonna upload one. And I happen to have a copy here of the text, of the artof words, about 60,000 words, and I can say open. And as soon as I do that,we basically start taking this thing, uploading it,parsing it, and creating vector embeddings. You can see the progress that's happening here.
And then once this has been vectorized here in a moment,we'll be able to sort of browse through, uh, the, the text,the, the vector embeddings, see the chunks, uh,and then I'll go back to the main flow. And of course, I could have just donethat if I was to this reel. I didn't wanna watch this and,and go start to implement the query, uh, block. But I just wanna sort of have you guys watch thisas it's happening behind the scenes. So now, obviously, again, so this can be usedby completely non-technical people.
We now have thousands, you know, tons of thousands of peoplethat have learned to use this vectorization without doing. Okay. So, uh, we've now created almost 140,000 vectors. We can click on this little thing here,and again, see the preview, see the extracted text,see the chunks, uh, see the raw vectors,and then we can reference a query this, uh, data sourceby this query data block. And then choosing the source,giving it the output variable name, specifyinghow many results, and basicallycreating this query template, uh, to be ableto extract what we want.
Other things you can do here is you can, uh, uh, createand, uh, and run custom functions. Uh, and so we've got,first you can browse our community of functions. This is sort of, kind of like Zapier has, you know,over 6,000. We've got like three dozen of these,but you're welcome to use them directly from here. Uh, and again here you see like things like Airtableand Calendly and sort of general, uh, fetch requests and,and image generation, stable diffusion, dolly, uh, you know,YouTube, Google search, things like that.
Or you can, you know, create and write your own functionsand test them and,and sort of do all of that directly in here in this id, uh,and create tests, run them and, and,and call them, uh, here. And, uh, you can also create things like menus, for example. And if you create menus, you can then, you know,take those menus and then create multiple workflowsand call them, you know, logic blocks, things like that. Throughout all of this, you get to sort of see errorsthat still exist as you run this thing. You got a, a debugger, so you can, you can trace stuff,you can, uh, preview this at any moment.
There's a draft and another tab. Again, these are just like web applications,and then you can publish them. And as you publish them, you know, you get to provide,uh, some metadata. This is also the place where you would, you know, set your,um, be able to get your API keys. If you are gonna deploy this as a, as a headless, uh,ai, you can embed these.
So there's like thousands of websites nowthat embed these directly on their website and,and, you know, mostly create like a customer serviceor sales assistance or things like that. Um, and then, uh, and then you publish it,and once you publish it, you get a, uh, a URLand you, you get a web application. And, and yeah, that's generally, uh, again,there's a lot more to it,but, uh, you guys can find that, uh, on your own timeand, and dive into that. That looks really awesome. Um,I guess the next things we would love to know is like,what are some of the exciting feature on the roadmap?Actually, uh, Tom was asked like, same questions.
Uh, what you plan for expanding the capability of my studio,and how do you envision it involvesto meet the changing needs of the AI developmentlandscape? Mm-Hmm. Yeah. So the first thing I think the,that Sean touched uponand I touched upon that I'll bring back,I think like really important is to understand likehow we see, uh, where we sitand like where we draw the lines. Like what, what are the abstractions that we are creating?So, uh, uh, we believe obviously, uh, that, uh,forever and ever, we will continue to see, you know,accelerating innovation in sort of the different layersof the AI stack innovationand sort of this like infrastructureand hardware layer that we're already seeing, um, uh,you know, with the stock price of Nvidia, uh, going upand up and up and, and the demand for computeand, you know, lots of innovation there on, on, uh,you know, rack density and coolingand all this stuff that data centersneed to be able to, to run this. So this can be con continued innovation there.
We don't play any part in it, but we leverage all of that,and we assume that's gonna keep going faster and fasterand get cheaper and cheaper, uh, on top ofthat infrastructure layer, you know, we, we,we call this next layer the intelligence layer,and the intelligence layer has all the models,all the models today and moving forward, that too is movingludicrously fast. Like if that's all you do is like track the space,it's impossible to keep up every week,massive innovations and changes. You know, two weeks ago, you know,GPT-4 turbo was the thing. Then all of a sudden anthropic you, uh, launched, uh,you know, their latest modeland all of a sudden everybody's like, no, you gotta switch. That's the latest model, and, you know,next week it's gonna be something else.
And also, obviously in this case, uh, meaning in the case ofof AI models, uh,open source is clearly gonna play a massive role insort of the evolution of this. Uh, uh, and, and so, uh, that's already happening. And, and we believe that will continueto be the case and get even stronger. And also, uh, you know, as enterprises start to, um, uh,implement these things, they start to realize thatfor most things, you don't need large language models. You would be much better served by taking a small model,being able to fine tune it sort of on an ongoing basis.
It's, uh, much more performant, you know, lower latency,you can run it yourselfso you get better security, compliance, whatever. Uh, you can keep it fresh, sortof retrain it much more often. And so we believe all of that will continue to evolve. We don't play in that layer either. You know, we intentionally sit one layer above thatwhere we are the, the gateway to those layerswhere the application layer sitting on top of those things,uh, and never want to, uh, pick winners there.
Simply want to allow, you know, our users to be ableto leverage all the power now and in the future ofhowever that evolution happens. Um, so, so, uh, hopefully that makes sense. So like there's gonna be tremendous amount of change there,uh, but Mind Studio supports it nowand will support it moving forwardbecause all models are basically just, you know, cloud,metered cloud services. They're, they're, they're, they're, uh, thingsthat are meant to be accessible to, you know,developers or consumers. And we are the abstraction layer for that.
Okay. The places are, sorry, quickly,ETRI, I wanted to interruptand kind of just share to that effect. So like, this is something just, it's just visually fun. So I feel like we're sharing right now, which is, uh,something we released last week, call the Profiler. And what the profiler does is let you run the same inputthrough multiple l omsor through the same modelwith different settings at the same time in orderto actually be able to visualize, uh, right me,uh, to actually visualize the differencesbetween these models in terms of speed and output and costand stuff like that, in order to help, um,end users make more informed choicesabout what it is they're using.
Because it, it's one thingto read about all these advancementsand read about benchmarksand comparisons and watch the videos. It's another thing to be able to seewith your own eyes in your specific unique workflow. So there's just a, a fun thing that you can see allof these side by side like that. Yeah. And you can keep adding more and more of these.
At the bottom left, there are new profiles. So like you, you can run a lot of these simultaneouslyand show, uh, Sean, where you change the model,uh, on each one of those. You can just click on thatand choose the model, choose other parameters,and, and run it with that. Yeah. Uh, okay.
So yeah, this is by the way, really powerful tool. Uh, so, uh, it's, it's a bit hidden. It's in these workflowsand then this like, uh, middle section here,it's called profiler. So take note of that. This two I think is now documented,but we're catching up on all of that.
Um, okay, so, so our, um, the placewhere we spend our time is inthe subtraction layer in this application layer of ai. And so we, uh, uh, commit to being ableto support any model because all models are callable viaAPIs, and that's how we use them. Uh, by the way, when, uh, new modelsthat we already support these anthropic, Google meta, uh,menstrual, uh, OpenAI, when they come out, uh,if they come out sort of during our waking hours, we tend toannounce the, it's already supported 15 minutesafter they've been released, meaning we, we,we put 'em in our list and allow you to call them VAP. And, uh, and so, um, uh, check. The other thing is again, uh, it is clear that for manyenterprises specifically, uh, that they will be, you know,using their own modelsand connecting to private cloudson premise, things like that.
And so today we have to periodically, you know,they reach out to us and ask us how to do it,but soon will make it even easier for them to be able to dothat than these like, model settings ofconfigure your own API keys and,and call your own models on this. Um, the, uh, we are working on, like,we've got everything obviously running, uh, being usedby over 40,000 of these, uh, of these AI deployed,but there's still a bunch of sort of fine finish work to do. And that's where we're spending our time right now is,is making it more robust, making it, uh, more easy to use,um, uh, providing enterprises with more capabilities theythat they need from a, uh, compliance standpointand privacy standpoint. Uh, a lot of this, like, you know, logging, auditing, um,things like that are, are possible,but still sort of require some work on their part. We're gonna make it much easier for them, uh, logging, uh,in enterprises of all of this AI usage.
Especially once you've done all the automationsand you've done the custom, uh, created custom business appsfor, for enterprise users,and you've given them these specialized assistance,and now you can see all of thatas it flows through the enterprise. Uh, that's a lot of really valuable data today. It gets stored, but there's not much you can do with it,you know, uh, at least through us. Uh, but soon, um,we'll be building this business intelligence component to itwhere we will make it easier for enterprisesto keep an eye on that data and sort of proactively watch itand detect all kinds of things. Uh, you know, primarily inefficiencies of like,even though you've automated a bunch of things,here's a bunch of things your employees are doing manuallythat we might want to automate, including by the way.
Uh, obviously we'd have a capability of sortof pre creating the app formand say, here's the automation already created,do you want to deploy it?Uh, so things like that. Um, so this business intelligence thing is a big thing on,uh, leveraging these models. As I mentioned, most of the aisthat are being created today are already use, uh,multiple models and oftentimes modelsfrom different providers. But today, the, the builder, the, the,the person creating the ai, you know,this business user in some enterprise, uh, needsto make decisions on like,what model should I use for this step?And while we help 'em with this profileor tool, for example, to be able to compare things and,and play around and, and create constraints and,and do that, um, certainly it makes sense to makethat sort of more, uh, uh, assistive, uh,and intelligent where we can propose, uh, that this modelfor this step of the process would be the right model. And here it's parametersand it's already sort of been pret tuned for you to do that.
You don't have to think about that stuff. Uh, the other thing that is really powerfuland we will make even more powerful is,so these model providers tend to go up and down, right?Like they're reliability still is a bit wonky. Again, early days of this expected. So, uh, you know, there's a few fail whales that that happenand, you know, enterprises complain,but like, oh, I've been using GPT-4 and it's down. Um, and today we make it easy for themto just like open up the AIand switch that, you know, just drop down, switch itto another model and, and go.
Um, but, uh, you know, certainly we can makethat much better and have an automatic failoverand fall tolerance and optimization for, um, you know,basically quality latency and, and priceand sort of intelligent, uh, way of doing that. So that's another area. Um, we're a small team, so, you know,at this moment we've got sort of finite things in mind,but we are growing our team,and so as we get bigger, I think we'll startto dream a bit further, further out. Mm-Hmm. Uh, we have about one minute left.
Let's try to answer David's question. Uh, what do you think is the futureof abstraction use cases, for example, oneor two years from now, will JI to beprogressively more visible to a business user?What's your opinion?Uh, sorry, this t what's, what's the futureof abstraction, uh, as models evolve,capabilities of models evolve? Is that the question?It's like a chain AI will be more invisibleto business user, do you think?So?Probably, yes. Um, meaningI think from the spirit of like, will it be more sort ofnaturally and intuitively baked into toolsand workflows rather than a little bit of what we see today,which is like you go to a Google Docs and you tryand write something and there's, you know,the 2024 equivalent of Microsoft Clippy saying, Hey,do you want use AI to do this? Yeah,Yeah. Today AIis front and center, you know,because it's cool, right?And so everybody's sort of putting, and,and again, the way people think about ai,we've been conditioned by the release of chat GPT,that AI is a chat bot, you know,that you, you chat with this. Um, and, and that's simplybecause that's the way it was packaged.
Obviously AI is not a chat bot. Um, and, and so, uh, people tend to think of it as this,it's this thing that assists you and you can chat with. And when you build applications with it,that's this like model thingthat you put into a, a workflow. Uh, uh, uh, I'm certain that over timeand probably, you know, two years is a in, in today's world,and this world is I think is a very, very long time that,uh, a AI is gonna be much less sort of in your faceand, and, and doing things behind the scenesand that most users will never think about, uh, oh,I need to use AI. Or, you know, uh, how, how do I build things with ai?You know, the, the people who are responsiblefor making organizations operate efficiently, right?So, uh, again, our targets when we go and,and, you know, all of our stuff is inbound.
Today, as I mentioned, we don't have direct salespeople. Uh, but there's a bunch of agencies, for example,that are now starting to, you know, it integration agenciesand things like that who are starting to, uh, build aisfor enterprises using Mind Studio. And as they go out and, and,and, you know, they, they talk to these, um, uh,enterprises, the, uh, they have to sort of retrain themto think of AI in this right way,that AI shouldn't be something that employees generally are,you know, thinking about or learning to use. Like you don't need to teach anybodyto do prompt engineering. You the person responsible for enabling your organizationto operate efficiently, whether you're an operations manageror sometimes now you get this like title Chief AI officer,or you are, uh, sort of sales enablementor, you know, customer support enablement or whatever.
You're like these people inside these organizationsthat are responsible for operational efficiency. You are the only ones that should ever think about ai. And AI is simply this like thing that, that you can sortof throw into these various types of, of, of workflowsthat you're creating, of these apps you're creating. And, and it just does these things. So I think that, um, very quickly, the,the general concept of how we even sort of think about and,and, and, and say what is AIis gonna change quite, quite dramatically.
That's very insightful and we're at the hour. Um, thank you so much Dmitriand Chang for providing us the story of my studio. And, uh, hope we'll talk with you guys sometime soon. Yeah, thanks for having us,and thanks everyone for joining. Thank you.
Have a great day. Thank you. Bye-Bye bye.
Meet the Speaker
Join the session for live Q&A with the speaker
Dmitry Shapiro
CEO and Co-Founder of MindStudio
Dmitry Shapiro, the CEO of MindStudio, ran product on three machine learning teams at Google from 2012-2016, was CTO of MySpace Music, and built two other venture-backed companies (raising over $140M in venture capital).Sean Thielen
CTO and Co-Founder of MindStudio
Sean Thielen is a self-taught developer who studied Literature in college. The two met on Hacker News, a social networking site geared towards technology entrepreneurs. Together they ushered in the new era of the metaverse by founding GoMeta in 2016 - a company way ahead of its time. Soon after they founded Koji, one of the most innovative interactive content companies. Now their latest venture, MindStudio is a platform that allows for rapid creation of model-agnostic AI-powered applications. MindStudio is being used by tens of thousands of AI developers inside of enterprises, government agencies, SMBs, non-profits, and more.