To become an expert in computer vision, start with foundational topics like image processing, feature extraction, and traditional computer vision techniques (e.g., edge detection, filtering, and keypoint detection). Then, delve into machine learning and deep learning, focusing on models such as convolutional neural networks (CNNs) and transformers for vision tasks. Master frameworks like OpenCV, PyTorch, and TensorFlow, which are essential for implementing and experimenting with computer vision algorithms. Familiarity with datasets like ImageNet, COCO, and Open Images is important for training and evaluating models. Advanced topics include 3D vision, stereo imaging, SLAM (Simultaneous Localization and Mapping), and multimodal learning. Staying updated with the latest research and participating in projects or competitions can also accelerate your expertise.
What should I learn to become an expert in Computer Vision?
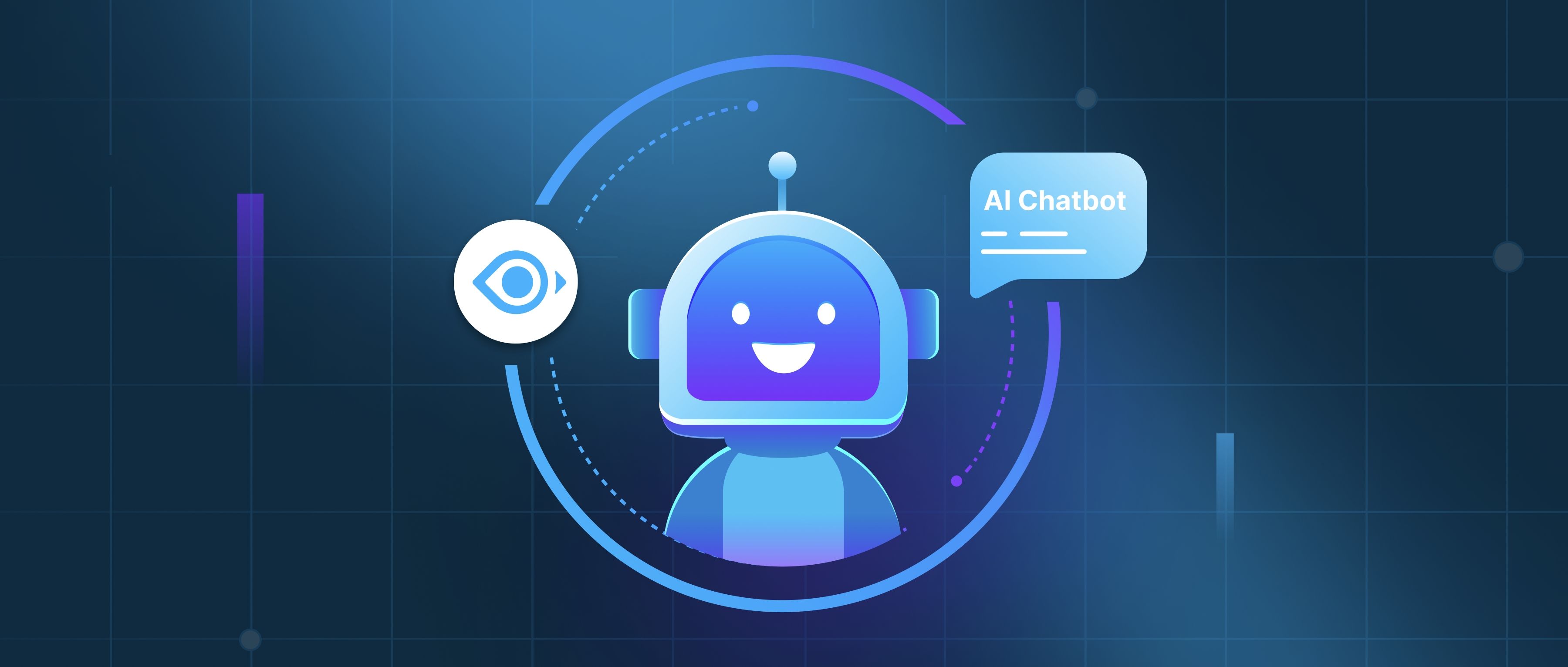
- AI & Machine Learning
- Large Language Models (LLMs) 101
- Getting Started with Zilliz Cloud
- Vector Database 101: Everything You Need to Know
- Natural Language Processing (NLP) Basics
- All learn series →
Recommended AI Learn Series
VectorDB for GenAI Apps
Zilliz Cloud is a managed vector database perfect for building GenAI applications.
Try Zilliz Cloud for FreeKeep Reading
What is the difference between data lakes and data warehouses?
Data lakes and data warehouses are two distinct types of data storage systems, each serving different needs and purposes
What is strong consistency?
Strong consistency is a data consistency model where all read operations return the most recent write at any given time.
What is the role of ETL in data movement?
ETL, which stands for Extract, Transform, and Load, plays a crucial role in data movement by facilitating the process of