Vector search, or vector similarity search, is a technique to find similar items within a dataset by representing them as high-dimensional vectors. Unlike keyword search, which relies on exact term matching, vector search evaluates semantic relationships, enabling it to retrieve results based on meaning or context. For instance, a search for "apple" could return results about the fruit or the company based on contextual clues. The core idea is to measure how "close" vectors are in a multi-dimensional space to identify related items. This process involves mapping data—like text, images, or audio—into numerical vectors. These vectors reside in a high-dimensional space where similar items cluster together. The similarity between vectors is determined using metrics such as Euclidean distance or cosine similarity. For example, two similar images might have vectors that are closer together than those of unrelated images. Indexing methods like k-d trees or HNSW graphs help efficiently organize and retrieve vectors. Applications of vector search are vast. It's used in recommendation systems (e.g., Netflix suggesting movies), semantic search engines (e.g., retrieving contextually relevant documents), and image retrieval systems (e.g., finding visually similar photos). By leveraging the power of embeddings and proximity-based comparisons, vector search offers precise and contextually relevant results, bridging the gap between raw data and actionable insights.
What is vector search?
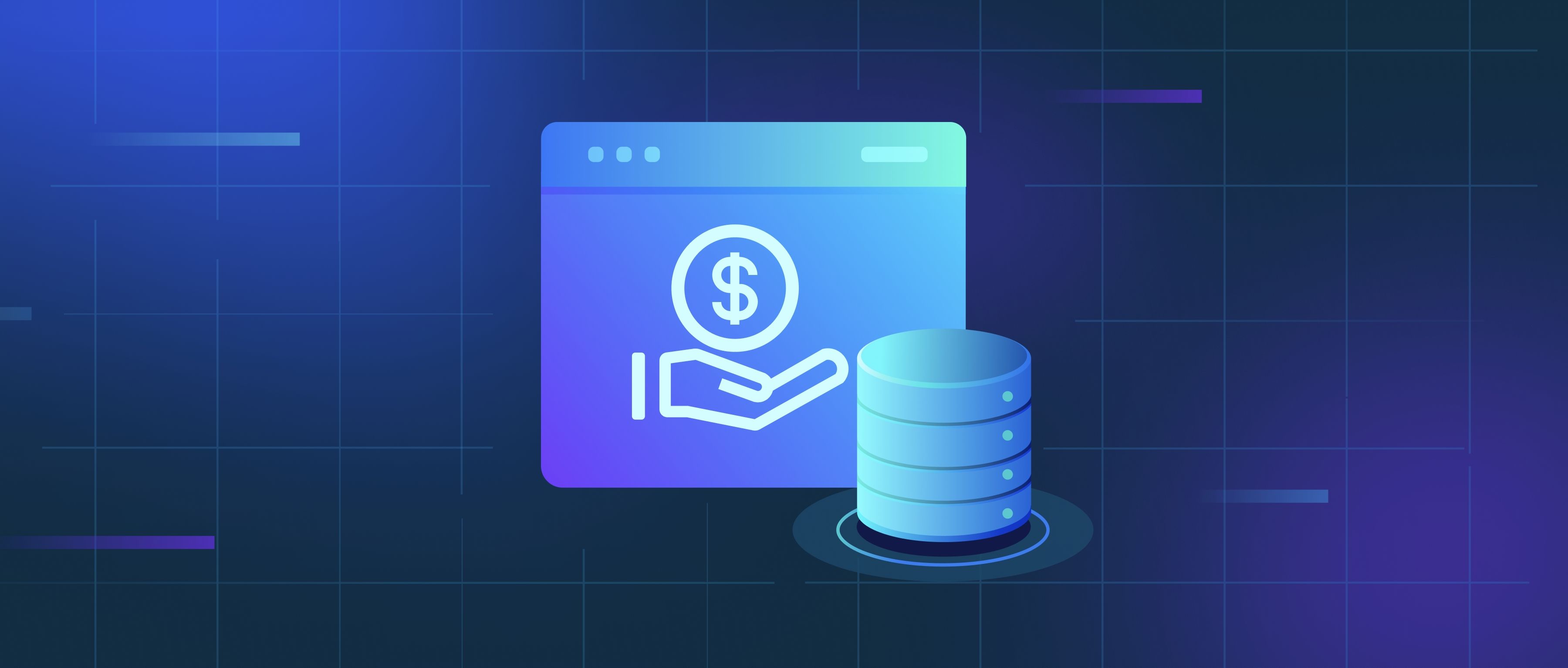
- Information Retrieval 101
- Evaluating Your RAG Applications: Methods and Metrics
- GenAI Ecosystem
- Large Language Models (LLMs) 101
- Getting Started with Milvus
- All learn series →
Recommended AI Learn Series
VectorDB for GenAI Apps
Zilliz Cloud is a managed vector database perfect for building GenAI applications.
Try Zilliz Cloud for FreeKeep Reading
How do robots recognize objects and environments?
Robots recognize objects and environments primarily through sensors and computer vision algorithms. Sensors collect data
What is real-time data analytics?
Real-time data analytics refers to the process of continuously analyzing data as it becomes available, allowing organiza
How do document databases handle concurrency?
Document databases handle concurrency by using various techniques that enable multiple users or applications to read and